1
Introduction and Fundamental Concepts
From a very young age, most humans recognize each other easily. A familiar voice, face, or manner of moving helps to identify members of the family—a mother, father, or other caregiver—and can give us comfort, comradeship, and safety. When we find ourselves among strangers, when we fail to recognize the individuals around us, we are more prone to caution and concern about our safety.
This human faculty of recognizing others is not foolproof. We can be misled by similarities in appearance or manners of dress—a mimic may convince us we are listening to a well-known celebrity, and casual acquaintances may be incapable of detecting differences between identical twins. Nonetheless, although this mechanism can sometimes lead to error, it remains a way for members of small communities to identify one another.
As we seek to recognize individuals as members of larger communities, however, or to recognize them at a scale and speed that could dull our perceptions, we need to find ways to automate such recognition. Biometrics is the automated recognition of individuals based on their behavioral and biological characteristics.1
1 |
“Biometrics” today carries two meanings, both in wide use. (See Box 1.1 and Box 1.2.) The subject of the current report—the automatic recognition of individuals based on biological and behavioral traits—is one meaning, apparently dating from the early 1980s. However, in biology, agriculture, medicine, public health, demography, actuarial science, and fields related to these, biometrics, biometry, and biostatistics refer almost synonymously to statistical and mathematical methods for analyzing data in the biological sciences. The two usages of |
BOX 1.1 History of the Field—Two Biometrics “Biometrics” has two meanings, both in wide use. The subject of this report—the automatic recognition of individuals based on biological and behavioral traits—is one meaning, which apparently dates from the early 1980s. In biology, agriculture, medicine, public health, demography, actuarial science, and fields related to these, “biometrics,” “biometry,” and “biostatistics” refer almost synonymously to statistical and mathematical methods for analyzing data in the biological sciences. This usage stems from the definition of biometry, proffered by the founder of the then-new journal Biometrika in its 1901 debut issue: “the application to biology of the modern methods of statistics.” The writer was the British geneticist Francis Galton, who made important contributions to fingerprinting as a tool for identification of criminals, to face recognition, and to the central statistical concepts of regression analysis, correlation analysis, and goodness of fit. Thus, the two meanings of “biometrics” overlap both in subject matter—human biological characteristics—and in historical lineage. Stigler (2000) notes that others had preceded the Biometrika founders in combining derivatives of the Greek βíος (bios) and μετρον (metron) to have specific meanings.1 These earlier usages do not survive. Johns Hopkins University opened its Department of Biometry and Vital Statistics (since renamed the Department of Biostatistics) in 1918. Graduate degree programs, divisions, and service courses with names incorporating “biostatistics,” “biometrics,” or “biometry” have proliferated in academic departments of health science since the 1950s. The American Statistical Association’s 24 subject-matter sections began with the Biometrics Section in 1938, which in 1945 started the journal Biometrics Bulletin, renamed Biometrics in 1947. In 1950 Biometrics was transferred to the Biometric Society (now the International Biometric Society), founded in 1947 at Woods Hole, Massachusetts. The journal promotes “statistical and mathematical theory and methods in the biosciences through … application to new and ongoing subject-matter challenges.” Concerned that Biometrics was overly associated with medicine and epidemiology, in 1996 the Society and the American Statistical Association jointly founded the Journal of Agricultural, Biological, and Environmental Statistics (JABES). The latter, along with other journals such as Statistics in Medicine and Biostatistics, have taken over the original mission of Biometrika, now more oriented to theoretical statistics. Automated human recognition began with semiautomated speaker recognition systems in the 1940s. Semiautomated and fully automated fingerprint, handwriting, and facial recognition systems emerged in the 1960s as digital computers became more widespread and capable. Fully automated systems based on hand geometry |
and fingerprinting were first deployed commercially in the 1970s, almost immediately leading to concerns over spoofing and privacy. Larger pilot projects for banking and government applications became popular in the 1980s. By the 1990s, the fully automated systems for both government and commercial applications used many different technologies, including iris and face recognition. Clearly both meanings of biometrics are well-established and appropriate and will persist for some time. However, in distinguishing our topic from biometrics in its biostatistical sense, one must note the curiosity that two fields so linked in Galton’s work should a century later have few points of contact. Galton wished to reveal the human manifestations of his cousin Charles Darwin’s theories by classifying and quantifying personal characteristics. He collected 8,000 fingerprint sets, published three books on fingerprinting in four years,2 and proposed the Galton fingerprint classification system extended in India by Azizul Haque for Edward Henry, Inspector General of Police, in Bengal. It was documented in Henry’s book Classification and Uses of Finger Prints. Scotland Yard adopted this classification scheme in 1901 and still uses it. But not all of Galton’s legacy is positive. He believed that physical appearances could indicate criminal propensity and coined the term “eugenics,” which was later used to horrific ends by the Third Reich. Many note that governments have not always used biologically derived data on humans for positive ends. Galton’s work was for understanding biological data. And yet biostatisticians, who have addressed many challenges in the fast-moving biosciences, have been little involved in biometric recognition research. And while very sophisticated statistical methods are used for the signal analysis and pattern recognition aspects of biometric technology, the systems and population sampling issues that affect performance in practice may not be fully appreciated. That fields once related are now separate may reflect that biometric recognition is scientifically less basic than other areas of interest, or that funding for open research is lacking, or even that most universities have no ongoing research in biometric recognition. A historical separation between scientifically based empirical methods developed specifically in a forensic context and similar methods more widely vetted in the open scientific community has been noted in other contexts and may also play a role here.3,4 |
BOX 1.2 A Further Note on the Definition of Biometrics The committee defines biometrics as the automated recognition of individuals based on their behavioral and biological characteristics. This definition is consistent with that adopted by the U.S. government’s Biometric Consortium in 1995. “Recognition” does not connote absolute certainty. The biometric systems that the committee considers always recognize with some level of error. This report is concerned only with the recognition of human individuals, although the above definition could include automated systems for the recognition of animals. The definition used here avoids the perennial philosophical debate over the differences between “persons” and “bodies.”1 For human biometrics, an individual can only be a “body”. In essence, when applied to humans, biometric systems are automated methods for recognizing bodies using their biological and behavioral characteristics. The word “individual” in the definition also limits biometrics to recognizing single bodies, not group characteristics (either normal or pathological). Biometrics as defined in this report is therefore not the tool of a demographer or a medical diagnostician nor is biometrics as defined here applicable to deception detection or analysis of human intent. The use of the conjunction “and” in the phrase “biological and behavioral characteristics” acknowledges that biometrics is about recognizing individuals from observations that draw on biology and behaviors. The characteristics observable by a sensing apparatus will depend on current and, to the extent that the body records them, previous activities (for example, scars, illness aftereffects, physical symptoms of drug use, and so on). |
Many traits that lend themselves to automated recognition have been studied, including the face, voice, fingerprint, and iris. A key characteristic of our definition of biometrics is the use of “automatic,” which implies, at least here, that digital computers have been used.2 Computers, in turn, require instructions for executing pattern recognition algorithms on trait samples received from sensors. Because biometric systems use sensed traits to recognize individuals, privacy, legal, and sociological factors are
involved in all applications. Biometrics in this sense sits at the intersection of biological, behavioral, social, legal, statistical, mathematical, and computer sciences as well as sensor physics and philosophy. It is no wonder that this complex set of technologies called biometrics has fascinated the government and the public for decades.
The FBI’s Integrated Automatic Fingerprint Identification System (IAFIS) and smaller local, state, and regional criminal fingerprinting systems have been a tremendous success, leading to the arrest and conviction of thousands of criminals and keeping known criminals from positions of trust in, say, teaching. Biometrics-based access control systems have been in continuous, successful use for three decades at the University of Georgia and have been used tens of thousands of times daily for more than 10 years at San Francisco International Airport and Walt Disney World.
There are challenges, however. For nearly 50 years, the promise of biometrics has outpaced the application of the technology. Many have been attracted to the field, only to leave as companies go bankrupt. In 1981, a writer in the New York Times noted that “while long on ideas, the business has been short on profits.”3 The statement continues to be true nearly three decades later. Technology advances promised that biometrics could solve a plethora of problems, including the enhancement of security, and led to growth in availability of commercial biometric systems. While some of these systems can be effective for the problem they are designed to solve, they often have unforeseen operational limitations. Government attempts to apply biometrics to border crossing, driver licenses, and social services have met with both success and failure. The reason for failure and the limitations of systems are varied and mostly ill understood. Indeed, systematic examinations that provide lessons learned from failed systems would undoubtedly be of value, but such an undertaking was beyond the scope of this report. Even a cursory look at such systems shows that multiple factors affect whether a biometric system achieves its goals. The next section, on the systems perspective, makes this point.
THE SYSTEMS PERSPECTIVE
One underpinning of this report is a systems perspective. No biometric technology, whether aimed at increasing security, improving throughput, lowering cost, improving convenience, or the like, can in and of itself achieve an application goal. Even the simplest, most automated, accurate, and isolated biometric application is embedded in a larger system. That system may involve other technologies, environmental factors, appeal policies shaped by security, business, and political considerations, or
idiosyncratic appeal mechanisms, which in turn can reinforce or vitiate the performance of any biometric system.
Complex systems have numerous sources of uncertainty and variability. Consider a fingerprint scanner embedded in a system aimed at protecting access to a laptop computer. In this comparatively simple case, the ability to achieve the fingerprint scan’s security objective depends not only on the biometric technology, but also on the robustness of the computing hardware to mechanical failures and on multiple decisions by manufacturer and employer about when and how the biometric technology can be bypassed, which all together contribute to the systems context for the biometric technology.
Most biometric implementations are far more complex. Typically, the biometric component is embedded in a larger system that includes environmental and other operational factors that may affect performance of the biometric component; adjudication mechanisms, usually at multiple levels, for contested decisions; a policy context that influences parameters (for example, acceptable combinations of cost, throughput, and false match rate) under which the core biometric technology operates; and protections against direct threats to either bypass or compromise the integrity of the core or of the adjudication mechanisms. Moreover, the effectiveness of such implementations relies on a data management system that ensures the enrolled biometric is linked from the outset to the nonphysical aspects of the enrolling individual’s information (such as name and allowed privileges). The rest of this report should be read keeping in mind that biometric systems and technologies must be understood and examined within a systems context.
MOTIVATIONS FOR USING BIOMETRIC SYSTEMS
A primary motivation for using biometrics is to easily and repeatedly recognize an individual so as to enable an automated action based on that recognition.4 The reasons for wanting to automatically recognize individuals can vary a great deal; they include reducing error rates and improving accuracy, reducing fraud and opportunities for circumvention, reducing costs, improving scalability, increasing physical safety, and improving convenience. Often some combination of these will apply. For example, almost all benefit and entitlement programs that have utilized
biometrics have done so to reduce costs and fraud rates, but at the same time convenience may have been improved as well. See Box 1.3 for more on the variety of biometric applications.
Historically, personal identification numbers (PINs), passwords, names, social security numbers, and tokens (cards, keys, passports, and other physical objects) have been used to recognize an individual or to verify that a person is known to a system and may access its services or benefits. For example, access to an automatic teller machine (ATM) is generally controlled by requiring presentation of an ATM card and its corresponding PIN. Sometimes, however, recognition can lead to the denial of a benefit. This could happen if an individual tries to make a duplicate claim for a benefit or if an individual on a watch list tries to enter a controlled environment.
But reflection shows that authorizing or restricting someone because he or she knows a password or possesses a token is just a proxy for verifying that person’s presence. A password can be shared indiscriminately or a physical token can be given away or lost. Thus, while a system can be
BOX 1.3 The Variety of Biometric Applications Biometric technology is put to use because it can link a “person” to his or her claims of recognition and authorization within a particular application. Moreover, automating the recognition process based on biological and behavioral traits can make it more economical and efficient. Other motivations for automating the mechanisms for recognizing individuals using biometric systems vary depending on the application and the context in which the system is deployed; they include reducing error rates and improving accuracy; reducing fraud and circumvention; reducing costs; improving security and safety; improving convenience; and improving scalability and practicability. Numerous applications employ biometrics for one or more of these reasons, including border control and criminal justice (such as prisoner handling and process), regulatory compliance applications (such as monitoring who has access to certain records or other types of audits), determining who should be entitled to physical or logical access to resources, and benefits and entitlement management. The scope and scale of applications can vary a great deal—biometric systems that permit access might be used to protect resources as disparate as a nuclear power plant or a local gym. Even though at some level of abstraction the same motivation exists, the systems are likely to be very different and to merit different sorts of analysis, testing, and evaluation (see Chapter 2 for more on how application parameters can vary). The upshot of this wide variety of reasons for using biometric systems is that much more information is needed to assess the appropriateness of a given system for a given purpose beyond the fact that it employs biometric technology. |
confident that the right password or token has been presented for access to a sensitive service, it cannot be sure that the item has been presented by the correct individual. Proxy mechanisms are even more problematic for exclusion systems such as watch lists, as there is little or no motivation for the subject to present the correct information or token if doing so would have adverse consequences. Biometrics offers the prospect of closely linking recognition to a given individual.
HUMAN IDENTITY AND BIOMETRICS
Essential to the above definition of biometrics is that, unlike the definition sometimes used in the biometrics technical community, it does not necessarily link biometrics to human identity, human identification, or human identity verification. Rather, it measures similarity, not identity. Specifically, a biometric system compares encountered biological/behavioral characteristics to one or more previously recorded references. Measures found to be suitably similar are considered to have come from the same individual, allowing the individual to be recognized as someone previously known to the system. A biometric system establishes a probabilistic assessment of a match indicating that a subject at hand is the same subject from whom the reference was stored.
If an individual is recognized, then previously granted authorizations can once again be granted. If we consider this record of attributes to constitute a personal “identity,” as defined in the NRC report on authentication,5 then biometric characteristics can be said to point to this identity record. However, the mere fact that attributes are associated with a biometric reference provides no guarantee that the attributes are correct and apply to the individual who provided the biometric reference.
Further, as there is no requirement that the identity record contain a name or other social identifier, biometric approaches can be used in anonymous applications. More concisely, such approaches can allow for anonymous identification or for verification of an anonymous identity. This has important positive implications for the use of biometrics in privacy-sensitive applications. However, if the same biometric measure is used as a pointer to multiple identity records for the same individual across different systems, the possibility of linking these records (and hence the various social identities of the same person) raises privacy concerns. See Box 1.4 for a note on privacy.
BOX 1.4 A Note on Privacy Privacy is an important consideration in biometric systems. The report Who Goes There? Authentication Through the Lens of Privacy,1 focused on the intersection of privacy and authentication systems, including biometrics. Much of that analysis remains relevant to current biometric systems, and this report does not have much to add on privacy other than exploring some of the social and cultural implications of biometric systems (see Chapter 4). This reliance on an earlier report does not suggest that privacy is unimportant. Rather, the committee believes that no system can be effective without considerable attention to the social and cultural context within which it is embedded. The 2003 NRC report just referred to and its 2002 predecessor, which examined nationwide identity systems,2 should be viewed as companions to this report. |
The Fundamental Dogma of Biometrics
The finding that an encountered biometric characteristic is similar to a stored reference does not guarantee an inference of individualization—that is, that a single individual can be unerringly selected out of a group of all known individuals (or, conversely, that no such individual is known). The inference that similarity leads to individualization rests on a theory that one might call the fundamental dogma of biometrics:
An individual is more similar to him- or herself over time than to anyone else at any time.
This is clearly false in general; many singular attributes are shared by large numbers of individuals, and many attributes change significantly over an individual’s lifetime. Further, it will never be possible to prove (or falsify) this assertion precisely as stated because “anyone else” will include all persons known or unknown, and we cannot possibly prove the assertion for those who are unknown.6 In practice, however, we can relate similarity to individualization in situations where:
An individual is more likely similar to him- or herself over time than to anyone else likely to be encountered.
This condition, if met, allows us to individualize through similarity, but with only a limited degree of confidence, based on knowledge of probabilities of encounters with particular biometric attributes. The goal in the development and applications of biometric systems is to find characteristics that are stable and distinctive given the likelihood of encounters. If they can be found, then the above conditions are satisfied and we have a chance of making biometrics work—to an acceptable degree of certainty—to achieve individualization.
A better fundamental understanding of the distinctiveness of human individuals would help in converting the fundamental dogma of biometrics into grounded scientific principles. Such an understanding would incorporate learning from biometric technologies and systems, population statistics, forensic science, statistical techniques, systems analysis, algorithm development, process metrics, and a variety of methodological approaches. However, the distinctiveness of biometric characteristics used in biometric systems is not well understood at scales approaching the entire human population, which hampers predicting the behavior of very large scale biometric systems.
The development of a science of human individual distinctiveness is essential to the effective and appropriate use of biometrics as a means of human recognition and encompasses a range of fields. This report focuses on the biometric technologies themselves and on the behavioral and biological phenomena on which they are based. These phenomena have fundamental statistical properties, distinctiveness, and varying stabilities under natural physiological conditions and environmental challenges, many aspects of which are not well understood.
BASIC OPERATIONAL CONCEPTS
In this section, the committee outlines some of the concepts underlying the typical operation of biometric systems in order to provide a framework for understanding the analysis and discussion in the rest of the report.7 Two concepts are discussed: sources of (1) variability and
(2) uncertainty in biometric systems and modalities, including multibiometric approaches.
Sample Operational Process
The operational process typical for a biometric system is given in Figure 1.1. The main components of the system for the purposes of this discussion are the capture (whereby the sensor collects biometric data from the subject to be recognized), the reference database (where previously enrolled subjects’ biometric data are held), the matcher (which compares presented data to reference data in order to make a recognition decision), and the action (whereby the system recognition decision is revealed and actions are undertaken based on that decision.8
This diagram presents a very simplified view of the overall system. The operational efficacy of a biometric system depends not only on its technical components—the biometric sample capture devices (sensors) and the mathematical algorithms that create and compare references—but also on the end-to-end application design, the environment in which the biometric sensor operates, and any conditions that impact the behavior of the data subjects, that is, persons with the potential to be sensed.
For example, the configuration of the database used to store references against which presented data will be compared affects system performance. At a coarse level, whether the database is networked or local is a primary factor in performance. Networked databases need secure communication, availability, and remote access privileges, and they also raise more privacy challenges than do local databases. Local databases, by contrast, may mean replicating the reference database multiple times, raising security, consistency, and scalability challenges.9 In both cases, the accuracy and currency of any identification data associated with reference
issued reports that elaborate on biometrics systems with an eye to meeting government needs. See, for example, “The National Biometrics Challenge,” available at http://www.biometrics.gov/Documents/biochallengedoc.pdf, and “NSTC Policy for Enabling the Development, Adoption and Use of Biometric Standards,” available at http://www.biometrics.gov/Standards/NSTC_Policy_Bio_Standards.pdf. |
8 |
The data capture portion of the process has the most impact on accuracy and throughput and has perhaps been the least researched portion of the system. The capture process, which involves human actions (even in covert applications) in the presence of a sensor, is not well understood. While we may understand sensor characteristics quite well, the interaction of the subject with the sensors merits further attention. See Chapter 5 for more on research opportunities in biometrics. |
9 |
Both the NRC report Who Goes There? (2003) and the EC Data Protection Working Party discuss the implications of centralized or networked data repositories versus local storage of data. The latter is available at http://ec.europa.eu/justice_home/fsj/privacy/docs/wpdocs/2003/wp80_en.pdf. |
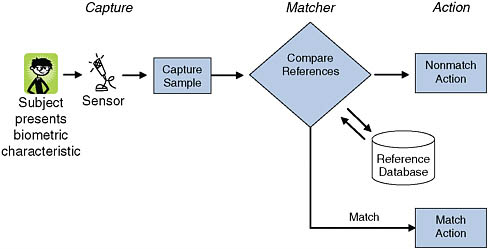
FIGURE 1.1 Operation of a biometric system.
characteristics in a biometric system are independent of the likelihood that a sample came from the individual who provided the reference. In other words, increasing confidence in a recognition result does not commensurately increase our confidence in the validity of any associated data.
Measures of Operational Efficacy
Key aspects of operational efficacy include recognition error rates; speed; cost of acquisition, operation, and maintenance; data security and privacy; usability; and user acceptance. Generally, trade-offs must be made across all of these measures to achieve the best-performing system consistent with operational and budgetary needs. For example, recognition error rates might be improved by using a better but more time-consuming enrollment process; however, the time added to the enrollment process could result in queues (with loss of user acceptance) and unacceptable costs.
In this report the committee usually discusses recognition error rates in terms of the false match rate (FMR; the probability that the matcher recognizes an individual as a different enrolled subject) and the false nonmatch rate (FNMR; the probability that the matcher does not recognize a previously enrolled subject). FMR and FNMR refer to errors in the matching process and are closely related to the more frequently reported false acceptance rate (FAR) and the false rejection rate (FRR). FAR and FRR refer to results at a broader system level and include failures arising from additional factors, such as the inability to acquire a sample. The committee uses these terms less frequently as they can sometimes intro-
duce confusion between the semantics of “acceptance” and “rejection” in terms of the claimed performance for biometric recognition versus that for the overall application. For example, in a positive recognition system, a false acceptance occurs when subjects are recognized who should not be recognized—either because they are not enrolled in the system or they are someone other than the subject being claimed. In this case the sense of false acceptance is aligned for both the biometric matching operation and the application function. In a system designed to detect and prevent multiple enrollments of a single person, sometimes referred to as a negative recognition system, a false acceptance results when the system fails to match the submitted biometric sample to a reference already in the database. If the system falsely matches a submitted biometric sample to a reference from a different person, the false match results in a denial of access to system resources (a false rejection).
Variability and Uncertainty
Variability in the biometric data submitted for comparison to the enrolled reference data can affect performance. As mentioned above, the matching algorithm plays a role in how this variability is handled. However, many other factors can influence performance, depending on how the specific biometric system is implemented. Generally speaking, biometric applications automatically capture aspects of one or more human traits to produce a signal from which an individual can be recognized. This signal cannot be assumed to be a completely accurate representation of the underlying biometric characteristic. Biometric systems designers and experts have accepted for some time that noise in the signal occurs haphazardly; while it can never be fully controlled, it can be modeled probabilistically.10 Also, not all uncertainty about biometric systems is due to random noise. Uncertainty pervades a biometric system in a number of ways. Several potential sources of uncertainty or variation are discussed here and should be kept in mind when reading the rest of this report. These sources are listed based on the order of events that take place as an individual works with the system to gain recognition.
-
Depending on the biometric modality, information content presented by the same subject at different encounters may be affected by changes in age, environment, stress, occupational factors, training and prompting, intentional alterations, sociocultural aspects of the situation in which the presentation occurs, changes in human interface with the bio-
10 |
See for example, J.P. Campbell, Jr., Testing with the YOHO CD-ROM Voice Verification Corpus, The Biometrics Consortium, available at http://www.biometrics.org/REPORTS/ICASSP95.html. |
-
metric system, and so on. These factors are important both at the enrollment phase and during regular operation. The next section describes within-person and between-person variability in depth.
-
Sensor operation is another source of variability. Sensor age and calibration can be factors, as well as how precisely the system-human interface at any given time stabilizes extraneous factors. Sensitivity of sensor performance to variation in the ambient environment (such as light levels) can play a role.
-
The above sources may be expected to induce greater variation in the information captured by different biometric acquisition systems than in the information captured by the same system. Other factors held constant, information on a single subject captured from repeated encounters with the same sensor will vary less than that captured from different sensors in the same system, which will vary less than that captured from encounters with different systems.
In addition to information capture, performance variation across biometric systems and when interfacing components of different systems depends on how the information is used, including the following:
-
Differences in feature extraction algorithms affect performance, with effects sometimes aggravated by the need for proprietary systems to be interoperable.
-
Differences between matching algorithms and comparison scoring mechanisms. How these algorithms and mechanisms interact with the preceding sources of variability of information acquired and features extracted also contributes to variation in performance of different systems. For instance, matching algorithms may differ in their sensitivity to biological and behavioral instability of the biometric characteristic over time, as well as the characteristic’s susceptibility to intentional modification.
-
The potential for individuals attempting to thwart recognition for one reason or another is another source of uncertainty that systems should be robust against. See below for a more detailed discussion on security for biometric systems.
In light of all of this, determining an appropriate action to take—where possible actions include to recognize, to not recognize, or to transition the system to a secondary recognition mechanism based on the signal from a biometric device—involves decision making under uncertainty.
Within- and Between-Person Variability
Variability in the observed values of a biometric trait can refer to variation in a given trait observed in the same person or to variation in
the trait observed in different persons. Effective overall system performance requires that within-person variability be small—the smaller the better—relative to between-person variability.
Within-Person Variation
Ideally, every time we measure the biometric trait of an individual, we should observe the same patterns. In practice, however, the different samples produce different patterns, which result in different digital representations (references). Such within-person variation, sometimes referred to as “intraclass variation,” typically occurs when an individual interacts differently with the sensor (for example, by smiling) or when the biometric details of a person (for example, hand shape and geometry or iris pigmentation details) change over time. The sensing environment (for example, ambient lighting for a face and background noise for a voice) can also introduce within-person variation. There are a number of ways to reduce or accommodate such variation, including controlled acquisition of the data, storage of many references for every user, and systematic updating of references. Reference updating, although essential to any biometric system since it can help account for changes in characteristics over time, introduces system vulnerabilities. Some biometric traits are more likely to change over time than others. Face, hand, and voice characteristics, in particular, can benefit from suitably implemented reference update mechanisms. Within-person variation can also be caused by behavioral changes over time.
Between-Person Variation
Between-person variation, sometimes referred to as “interclass variation,” refers generally to person-to-person variability. Since there is an inherent similarity between biometric traits among some individuals (faces of identical twins offer the most striking example), between-person variation between two individuals may be quite small. Also, a chosen digital representation (the features) for a particular biometric trait may not very effective in separating the observed patterns of particular subjects. In contrast, demographic heterogeneity among enrolled subjects in a biometric system database may contribute to large between-person variation in measurements of a particular biometric trait, although fluctuations in the sensing environment from which their presentation samples are obtained may contribute to large within-person variation as well.
It is the magnitude of within-person variation relative to between-person variation (observed in the context of a finite range of expression of human biometric traits) that determines the overlap between distributions of biometric measurements from different individuals and hence limits
the number of individuals that can be discriminated and recognized by a biometric system with acceptable accuracy. When within-person variation is small relative to between-person variation, large biometric systems with high accuracy are feasible because the distributions of observed biometric data from different individuals are likely to remain widely separated, even for large groups. When within-person variation is high relative to between-person variation, however, the distributions are more likely to impinge on each other, limiting the capacity of a recognition system. In other words, the number of enrolled subjects cannot be arbitrarily increased for a fixed set of features and matching algorithms without degrading accuracy. When considering the anticipated scale of a biometric system, the relative magnitudes of both within-person and between-person variations should be kept in mind.
Stability and Distinctiveness at Global Scale
Questions of variation and uncertainty become challenging at scale. In particular, no biometric characteristic, including DNA, is known to be capable of reliably correct individualization over the size of the world’s population. Factors that make unlikely the discovery of a characteristic suitably stable and distinctive over such a large population include the following:
-
Individuals without traits. Almost any trait that can be noninvasively observed will fail to be exhibited by some members of the human population, because they are missing the body part that carries the trait, because environmental or occupational exposure has eradicated or degraded the trait, or because their individual expression of the trait is anomalous in a way that confuses biometric systems.
-
Similar individuals. In sufficiently small populations it is highly likely that almost any chosen trait will be sufficiently distinctive to distinguish individuals. As populations get larger, most traits (and especially most traits that can be noninvasively observed) may have too few variants to guarantee that different individuals are distinguishable from one another. The population statistics for most biometric traits are poorly understood.
-
Feature extraction effects. Even in cases where a biometric trait is distinctive, the process of converting the analog physical property of a human to a digital representation that can be compared against the properties of other individuals involves loss of detail—that is, information loss—and introduction of noise, both of which can obscure distinctions between individuals.
However, the lack of an entirely stable and distinctive characteristic at scale need not stand in the way of effective use of biometrics if the system is well designed. Some biometric systems might not have to deal with all people at all times but might need only to deal with smaller groups of people over shorter periods of time. It may be possible to find traits that are sufficiently stable and distinctive to make many types of applications practicable.
Implicit in all biometric systems are estimated probabilities of the sameness and difference of source of samples and stored references, separately for presenters of different types, such as residents and impostors. This leads to the explicit use of ratios of probabilities in some biometric recognition algorithms. Because assessing the likelihood that a sample came from any particular reference may involve computing similarity to many references through use of ratios of probabilities or other normalization techniques, it cannot be strictly said that any form of recognition involves comparison of only one sample to one known reference. However, verification of a claim of similarity of a sample with a specific reference may appear to those unfamiliar with the algorithmic options to involve only a single comparison. This form of verification is often referred to as “one to one.” Verification of claims of similarity to an unspecific reference is often referred to as a “one-to-many” application because many comparisons to assess similarity over the enrolled individuals are required.
Biometric Modalities
A biometric modality11 refers to a system built to recognize a particular biometric trait. Face, fingerprint, hand geometry, palm print, iris, voice, signature, gait, and keystroke dynamics are examples of biometric traits.12 In the context of a given system and application, the presentation of a user’s biometric feature involves both biological and behavioral aspects. Some common biometric modalities described by Jain et al. (2004)13 are
summarized briefly here. Many of the issues associated with biometric systems (and correspondingly much of the discussion in this report) are not modality-specific, although of course the choice of modality has implications for system design and, potentially, system performance.
Face
Static or video images of a face can be used to facilitate recognition. Modern approaches are only indirectly based on the location, shape, and spatial relationships of facial landmarks such as eyes, nose, lips, and chin, and so on. Signal processing techniques based on localized filter responses on the image have largely replaced earlier techniques based on representing the face as a weighted combination of a set of canonical faces. Recognition can be quite good if canonical poses and simple backgrounds are employed, but changes in illumination and angle create challenges. The time that elapses between enrollment in a system and when recognition is attempted can also be a challenge, because facial appearance changes over time.
Fingerprints
Fingerprints—the patterns of ridges and valleys on the “friction ridge” surfaces of fingers—have been used in forensic applications for over a century. Friction ridges are formed in utero during fetal development, and even identical twins do not have the same fingerprints. The recognition performance of currently available fingerprint-based recognition systems using prints from multiple fingers is quite good. One factor in recognition accuracy is whether a single print is used or whether multiple or tenprints (one from each finger) are used. Multiple prints provide additional information that can be valuable in very large scale systems. Challenges include the fact that large-scale fingerprint recognition systems are computationally intensive, particularly when trying to find a match among millions of references.
Hand Geometry
Hand geometry refers to the shape of the human hand, size of the palm, and the lengths and widths of the fingers. Advantages to this modality are that it is comparatively simple and easy to use. However, because it is not clear how distinctive hand geometry is in large populations, such systems are typically used for verification rather than identification. Moreover, because the capture devices need to be at least the size of a hand, they are too large for devices like laptop computers.
Palm Print
Palm prints combine some of the features of fingerprints and hand geometry. Human palms contain ridges and valleys, like fingerprints, but are much larger, necessitating larger image capture or scanning hardware. Palm prints, like fingerprints, have particular application in the forensic community, as latent palm prints can often be found at crime scenes.
Iris
The iris, the circular colored membrane surrounding the eye’s pupil, is complex enough to be useful for recognition. The performance of systems using this modality is promising. Although early systems required significant user cooperation, more modern systems are increasingly user friendly. However, although systems based on the iris have quite good FMRs, the FNMRs can be high. Further, the iris is thought to change over time, but variability over a lifetime has not been well characterized.14
Voice
Voice directly combines biological and behavioral characteristics. The sound an individual makes when speaking is based on physical aspects of the body (mouth, nose, lips, vocal cords, and so on) and can be affected by age, emotional state, native language, and medical conditions. The quality of the recording device and ambient noise also influence recognition rates.
Signature
How a person signs his or her name typically changes over time. It can also be strongly influenced by context, including physical conditions and the emotional state of the signer. Extensive experience has also shown that signatures are relatively easy to forge. Nevertheless, signatures have been accepted as a method of recognition for a long time.
Gait
Gait, the manner in which a person walks, has potential for human recognition at a distance and potentially, over an extended period of
14 |
See Sarah Baker, Kevin W. Bowyer, and Patrick J. Flynn, Empirical evidence for correct iris match score degradation with increased time lapse between gallery and probe images, International Conference on Biometrics, pp. 1170-1179 (2009). Available at http://www.nd.edu/~kwb/BakerBowyerFlynnICB_2009.pdf. |
time. Laboratory gait recognition systems are based on image processing to detect the human silhouette and associated spatiotemporal attributes. Gait can be affected by several factors, including choice of footwear, the walking surface, and clothing. Gait recognition systems are still in the development stage.
Keystroke
Keystroke dynamics are a biometric trait that some hypothesize may be distinctive to individuals. Indeed, there is a long tradition of recognizing Morse code operators by their “fists”—the distinctive patterns individuals used to create messages. However, keystroke dynamics are strongly affected by context, such as the person’s emotional state, his or her posture, type of keyboard, and so on.
Comparison of Modalities
Each biometric modality has its pros and cons, some of which were mentioned in the descriptions above. Moreover, even if some of the downsides could be overcome, a modality itself might have inherent deficiencies, although very little research into this has been done. Therefore, the choice of a biometric trait for a particular application depends on issues besides the matching performance. Raphael and Young identified a number of factors that make a physical or a behavioral trait suitable for a biometric application.15 The following seven factors are taken from an article by Jain et al.:16
-
Universality. Every individual accessing the application should possess the trait.
-
Uniqueness. The given trait should be sufficiently different across members of the population.
-
Permanence.17 The biometric trait of an individual should be sufficiently invariant over time with respect to a given matching algorithm. A trait that changes significantly is not a useful biometric.
-
Measurability. It should be possible to acquire and digitize the biometric trait using suitable devices that do not unduly inconvenience the
-
individual. Furthermore, the acquired raw data should be amenable to processing to extract representative features.
-
Performance. The recognition accuracy and the resources required to achieve that accuracy should meet the requirements of the application.
-
Acceptability. Individuals in the target population that will use the application should be willing to present their biometric trait to the system.
-
Circumvention. The ease with which a biometric trait can be imitated using artifacts—for example, fake fingers in the case of physical traits and mimicry in the case of behavioral traits—should conform to the security needs of the application.
Multibiometrics
As the preceding discussions make clear, using a single biometric modality may not always provide the performance18 needed from a given system. One approach to improving performance (error rates but not speed) is the use of multibiometrics, which has several meanings:19
-
Multisensors. Here, a single modality is used, but multiple sensors are used to capture the data. For example, a facial recognition system might employ multiple cameras to capture different angles on a face.
-
Multiple algorithms. The same capture data are processed using different algorithms. For example, a single fingerprint can be processed using minutiae and texture. This approach saves on sensor and associated hardware costs, but adds computational complexity.
-
Multiple instances. Multiple instances of the same modality are used. For example, multiple fingerprints may be matched instead of just one, as may the irises of both eyes. Depending on how the capture was done, such systems may or may not require additional hardware and sensor devices.
-
Multisamples. Multiple samples of the same trait are acquired. For example, multiple angles of a face or multiple images of different portions of the same fingerprint are captured.
-
Multimodal. Data from different modalities are combined, such as face and fingerprint, or iris and voice. Such systems require both hard-
18 |
The term “performance,” used in the biometrics community generally, refers broadly to error rates, processing speed, and data subject throughput. See, for example, http://www.biometrics.gov/Documents/Glossary.pdf. |
19 |
A. Ross and A.K. Jain, Multimodal biometrics: An overview. Proceedings of 12th European Signal Processing Conference. Available online at http://biometrics.cse.msu.edu/Publications/Multibiometrics/RossJain_MultimodalOverview_EUSIPCO04.pdf. |
-
ware (sensors) and software (algorithms) to capture and process each modality being used.
Hybrid systems that combine the above also may prove useful. For example, one could use multiple algorithms for each modality of a multimodal system. The engineering of multibiometric systems presents challenges as does their evaluation. There are issues related to the architecture and operation of multibiometrics systems and questions about how best to model such systems and then use the model to drive operational aspects. Understanding statistical dependencies is also important when using multibiometrics. For example, Are the modalities of hand geometry and fingerprints completely independent—beyond, say, the trivial correlation between a missing hand and the failure to acquire fingerprints? As a large-scale biometric system becomes multimodal, it is that much more important to adopt approaches and architectures that support interoperability and implementation of best-of-breed matching components. This would mean, for example, including matching software, image segmentation software, and sample quality assessment software as they become available.20 This approach to interoperability support would also facilitate replacing an outdated matcher with a newer, higher performing matcher without having to scrap the entire system and start from scratch. Likewise, new multibiometric fusion algorithms could be implemented without requiring a major system redesign. Finally, new human interface issues may come into play if multiple observations are needed of a single modality or of multiple modalities.21
COPING WITH THE PROBABILISTIC NATURE OF BIOMETRIC SYSTEMS
The probabilistic aspect of biometric systems is often missing from popular discussions of the technology. For the purposes of this discussion, the committee will ignore FAR and FRR and consider only the
sources of error that contribute to the false match rates (FMR) and false nonmatch rates (FNMR). In this context, the FMR is the probability that the incorrect trait is falsely recognized and the FNMR is the probability that a correct trait is falsely not recognized. The probability a correct trait is truly recognized is 1 − FNMR and the probability an incorrect trait is truly not recognized is 1 − FMR. Complicating matters, biometric match probabilities are only one part of what we need to help predict the real-world performance of biometric systems.22
It seems intuitively obvious that a declared nonmatch in a biometric system with both FMRs and FNMRs of 0.1 percent is almost certainly correct. Unfortunately, intuition is grossly misleading in this instance, and the common misconception can have profound sociological impacts (for example, it might lead to the assumption that a suspected criminal is guilty if the fingerprints or DNA samples from the suspect “match” those at the crime scene). Understanding why this natural belief is often wrong is one of the keys to understanding how to use biometrics effectively. From the perspective of statistical decision theory, it is not enough to focus on error rates. All they provide is the conditional probability of a recognition/nonrecognition given that the presenting individual should be recognized and the conditional probability of a nonrecognition given that the presenting individual should not be recognized.
To illustrate, we will consider first an access control system. Let us assume that we know by experience or experiment the probability that a false claim by an impostor will be accepted and the probability that a true claim by a legitimate user will be accepted. In an operational environment, however, we would like to know the converse: the probability that the claimant is an impostor, given that the claim was accepted by the system. That is, we wish to know the probability of a false claim given a recognition by the system. To perform such a probability inversion, it is necessary to use Bayes’ theorem. A fundamental characteristic of this theorem is that it requires the prior probability of a false claim (impostor) (see Box 1.5). That is, some information about the frequency of false claims/impostors is needed in order to know the probability that any given recognition by the system is in error. The following series of examples illustrates how the percentage of “right” decisions by a biometric system depends upon the impostor base rate,23 the percentage of “impostors” actually encountered by the system, not just on the error rates of the technology. The error rates
BOX 1.5 Decision Theory Components Required for Biometric Recognition A formal decision-theoretic formulation of biometric recognition, whether from a classical frequentist or a Bayesian perspective, is beyond the scope of this report. However, the flavor of such formulations is conveyed by some of their components:
Here is a simple formulation of Bayes’ theorem in this context: ![]() In this formulation, P(B|A) is the conditional probability of B given A, that is, the fraction of instances that B is true among instances when A is true, and the prior probability of a false claim is P(Impostor). Also, P(Not Impostor) = 1 – P(Impostor). |
(the FMR and FNMR) are independent of the impostor base rate, but all of these pieces of information are needed to understand the frequency that a given recognition (or nonrecognition) by the system is in error.
To return to the example above, imagine that we have installed a rather accurate biometric verification system to control entry to a college dormitory. Suppose that the system has a 0.1 percent FMR and a 0.1 percent FNMR. The system lets an individual into the dorm if it matches the
individual to a stored biometric reference—if the system does not find a match, it does not let the individual in. We would like to know how often a nonmatch represents an attempt by a nonresident “impostor” to get into the dorm.24 The answer, it turns out, is “it depends.”25
First, consider the case where the impostor base rate is 0 percent—that is, no impostors ever try to get into the dorm. In this case, all of the people using the biometric system are residents. Since the system has a 0.1 percent FNMR, it will generate a false nonmatch once every 1,000 authentication attempts. All of these nonmatches will be errors (because in this case all the people using the system are residents). In this case, over time we will discover that our confidence in a nonmatch is zero—because nonmatches are always false. Table 1.1 contains the calculations for this case. Figure 1.2 presents the information in Table 1.1.
Now consider a different case: For every 999 times a resident attempts entry, one nonresident impostor tries to get into the building. In this case, since the system has a 0.1 percent FMR, it will (just as in the preceding case) generate one false nonmatch for each 1,000 recognition attempts. But since the system also has a 0.1 percent FNMR, it will (with 99.9 percent probability) generate a nonmatch for the one nonresident impostor. On the average, therefore, every 1,000 recognition attempts will include one impostor (who will generate a correct nonmatch with overwhelming probability) and one resident who will generate an incorrect nonmatch. There will therefore be two nonmatches—50 percent of them correct and 50 percent of them incorrect—in every 1,000 authentication attempts. In this case, using the same system as in the preceding case, with the same sensor and the same 0.1 percent FNMR, we will observe 50 percent true nonmatches and 50 percent false nonmatches. Table 1.2 shows how to calculate confidence that a nonmatch will be true in this case. Figure 1.3 presents the information in Table 1.2.
Tables 1.3 and 1.4 calculate confidence in the truth of a nonmatch in cases where the impostor base rate is 1 percent (that is, where 1 percent of the people trying to get into the dorm are nonresident impostors) and in cases where the impostor base rate is 50 percent (that is, half the people trying to get into the dorm are nonresident impostors). (Figures 1.4 and 1.5 present calculations of the data in Tables 1.3 and 1.4, respectively.) Note that confidence in the truth of a nonmatch approaches 99.9 percent
24 |
For the purposes of the discussion, we assume that the imposter is ready and able to enter the dorm and in possession of any information or tokens needed to initiate the biometric verification process. |
25 |
This discussion and associated examples draw heavily on Weight-of-Evidence for Forensic DNA Profiles by David J. Balding (New York: Wiley, 2005) and are based primarily on material in its Chapter 2, “Crime on an Island,” and Chapter 3, “Assessing Evidence via Likelihood Ratios.” |
TABLE 1.1 Impostor Base Rate of 0%
Proffered Identity |
Authentication Attempts |
Biometric Decision |
Conclusion |
|
Match |
Nonmatch |
|||
Authentic |
1,000 |
1,000 × 99.9% = 999 |
1,000 × 0.1% = 1 |
Confidence that a nonmatcher is an impostor = fraction of impostors among nonmatches = 0/1 = 0% |
Impostor |
0 |
0 × 0.1% = 0 |
0 × 99.9% = 0 |
|
Total |
1,000 |
999 |
1 |
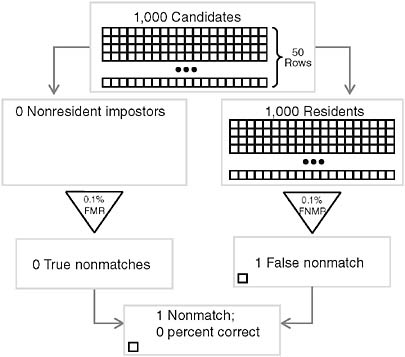
FIGURE 1.2 Authenticating residents (impostor base rate 0 percent; very low nonmatch accuracy).
(the true nonmatch rate of the system) only when at least half the people trying to get into the dorm are impostors!
These examples teach two lessons:
-
It is impossible to specify accurately the respective fractions of a biometric system’s matches and nonmatches that are correct without knowing how many individuals who “should” match and how many individuals who “should not” match are presenting to the system.
-
A biometric technology’s FMR and its FNMR are not accurate mea-
TABLE 1.2 Impostor Base Rate of 0.1%
Proffered Identity |
Authentication Attempts |
Biometric Decision |
Conclusion |
|
Match |
Nonmatch |
|||
Authentic |
999 |
999 × 99.9% = 998 |
999 × 0.1% = 1 |
Confidence that a nonmatcher is an impostor = fraction of impostors among nonmatches = 1/2 = 50% |
Impostor |
1 |
1 × 0.1% = 0 |
1 × 99.9% = 1 |
|
Total |
1,000 |
998 |
2 |
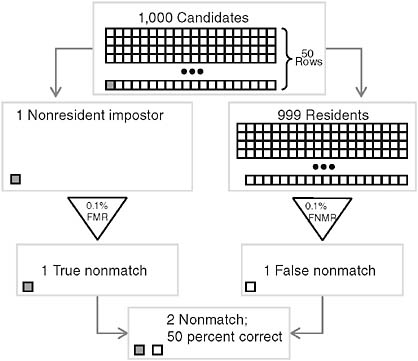
FIGURE 1.3 Authenticating residents (impostor base rate 0.1 percent; moderate nonmatch accuracy).
-
sures of how often the system gives the right answer in an operational environment and will in many cases greatly overstate the confidence we should have in the system.
The bad news, therefore, is that even with a very accurate biometric system, correctly identifying rare events (an impostor’s attempt to get into the dorm, in our first example) is very hard. The good news, by the same token, is that if impostors are very rare, our confidence in correctly identifying people who are not impostors (that is, determining that we are
TABLE 1.3 Impostor Base Rate of 1.0%
Proffered Identity |
Authentication Attempts |
Biometric Decision |
Conclusion |
|
Match |
Nonmatch |
|||
Authentic |
990 |
990 × 99.9% = 989 |
990 × 0.1% = 1 |
Confidence that a nonmatcher is an impostor = 10/11 = 91% |
Impostor |
10 |
10 × 0.1%= 0 |
10 × 99.9% = 10 |
|
Total |
1,000 |
989 |
11 |
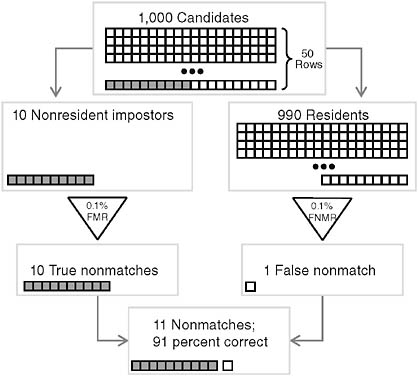
FIGURE 1.4 Authenticating residents (impostor base rate 1 percent; high nonmatch accuracy).
not in a rare-event situation) can be very high—far higher than 99.9 percent. In our first example, when the impostor base rate is 0.1 percent, our confidence in the correctness of a match is almost 100 percent (actually 99.9999 percent)—much higher than suggested by the FMR and FNMR. It is easy to see why this is true. Almost everyone who approaches the sensor in the dorm is actually a resident. For residents, all of whom are supposed to match, false matches are possible (one resident could claim to
TABLE 1.4 Impostor Base Rate of 50%
Proffered Identity |
Authentication Attempts |
Biometric Decision |
Conclusion |
|
Match |
Nonmatch |
|||
Authentic |
500 |
500 × 99.9% = 499 |
500 × 0.1% = 1 |
Confidence that a nonmatcher is an impostor = 499/500 = 99.8% |
Impostor |
500 |
500 × 0.1% = 1 |
500×99.9% = 499 |
|
Total |
1,000 |
500 |
500 |

FIGURE 1.5 Authenticating residents (impostor base rate 50 percent; very high nonmatch accuracy).
be another resident and match with that other resident’s reference), but a false match never results in a false acceptance, since a false match has the same system-level result—entrance to the dorm—as correct identification. A false match is possible only when an impostor approaches the sensor and is incorrectly matched. But almost no impostors ever approach the
TABLE 1.5 Impostor Base Rate of 0.1%
Proffered Identity |
Authentication Attempts |
Biometric Decision |
Conclusion |
|
Match |
Nonmatch |
|||
Authentic |
999 |
999 × 99.9% = 998 |
999 × 0.1% = 1 |
Confidence that a matcher is not an impostor = fraction of nonimpostors among matches = 998/998 = 100% |
Impostor |
1 |
1 × 0.1% = 0 |
1 × 99.9% = 1 |
|
Total |
1,000 |
998 |
2 |
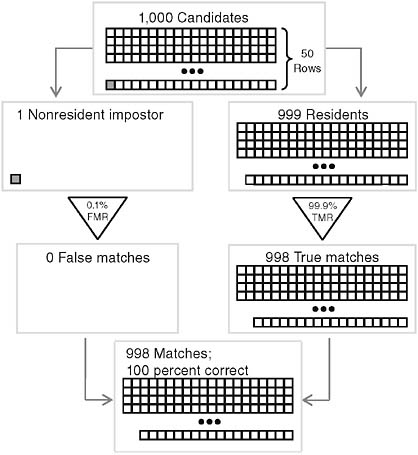
FIGURE 1.6 Authenticating residents (impostor base rate 0.1 percent; high match accuracy).
door, and (because the technology is very accurate) impostors who do approach the door are very rarely incorrectly matched. Table 1.5 provides the information for this case, and Figure 1.6 illustrates the case. Note that Figure 1.6 depicts only matches, in contrast to Figures 1.2 through 1.5, which depict only nonmatches.
The overall lesson is that as the impostor base rate declines in a recognition system, we become more confident that a match is correct but less confident that a nonmatch is correct. Examples of this phenomenon are common and well documented in medicine and public health. People at very low risk of a disease, for example, are usually not routinely screened, because positive results are much more likely to be a false alarm than lead to an early diagnosis. Unless the effects of the base rate on system performance are anticipated in the design of a biometric system, false alarms may consume large amounts of resources in situations where very few impostors exist in the system’s target population. Even more insidiously, frequent false alarms could lead screeners to a more lax attitude (the problem of “crying wolf”) when confronted with nonmatches. Depending on the application, becoming inured to nonmatches could be costly or even dangerous.26
ADDITIONAL IMPLICATIONS FOR OPEN-SET IDENTIFICATION SYSTEMS
The above discussion concerned an access control application. Large-scale biometric applications may be used for identification to prevent fraud arising from an individual’s duplicate registration in a benefits program or to check an individual’s sample against a “watch list”—a set of enrolled references of persons to be denied benefits (such as passage at international borders). This is an example of an open-set identification system, where rather than verifying an individual’s claim to an identity, the system determines if the individual is enrolled and may frequently be processing individuals who are not enrolled. The implications of Bayes’ theorem are more difficult to ascertain in this situation because here biometric processing requires comparing a presenting biometric against not just a single claimed enrollment sample, but against unprioritized enrollment samples of multiple individuals. Here, as above, the chances of erroneous matches and erroneous nonmatches still depend on the frequencies with which previously enrolled and unenrolled subjects present to the system. Such chances also depend on the length of the watch list and on how this length and the distribution of presenters27 to the system
interrelate. The overall situation is complex and requires detailed analysis, but some simple points can be made.
In general, additions to a watch list offer new opportunities for an unenrolled presenter to match with the list, and for an enrolled presenter to match with the wrong enrollee. If additions to the watch list are made in such a way as to leave the presentation distribution unchanged—for example, by enrolling persons who will not contribute to the presentation pool—then the ratio of true to false matches will decline, necessarily reducing confidence in a match. Appendix B formalizes this argument, incorporating a prior distribution for the unknown proportion of presenters who are previously enrolled.
We may draw an important lesson from this simple situation: Increasing list size cannot be expected to improve all aspects of system performance. Indeed, in an identification system with a stable presentation distribution, as list length increases we should become less confident that a match is correct.
A comment from the Department of Justice Office of the Inspector General’s report on the Mayfield mistake28 exemplifies this point: “The enormous size of the FBI IAFIS [Integrated Automated Fingerprint Identification System] database and the power of the IAFIS program can find a confusingly similar candidate print. The Mayfield case indicates the need for particular care in conducting latent fingerprint examinations involving IAFIS candidates because of the elevated danger in encountering a close nonmatch.”29
But this is not the end of the story, because in some circumstances changes in watch-list length may be expected to alter the presentation distribution. The literature distinguishes between open-set identification systems, in which presenters are presumed to include some persons not previously enrolled, and closed-set identification systems, in which presentations are restricted to prior enrollees. Closed-set identification systems meet the stable presentation distribution criterion de jure, so that the baseline performance response still automatically applies to the expanded list. But the actual effect of list expansion on system performance, when the presentation distribution in an open-set identification system may change, will depend on the net impact of modified per-presenter error rates and the associated rebalancing of the presentation distribution. In other words, the fact that the list has expanded may affect who is part
28 |
Brandon Mayfield, an Oregon attorney, was arrested by the FBI in connection with the Madrid train bombings of 2004 after a fingerprint on a bag of detonators was mistakenly identified as belonging to Mayfield. |
29 |
See http://www.usdoj.gov/oig/special/s0601/PDF_list.htm; http://www.usdoj.gov/oig/special/s0601/exec.pdf. |
of the pool of presenters. This rebalancing may occur without individuals changing their behavior simply because of the altered relationship between the length of the watch list and the size of the presenting population. But it may also occur as a result of intentional behavior change by new enrollees, who may stop or reduce their presentations to the system as a response to enrollment. Clearly, increasing watch list size without very careful thought may decrease the probability that an apparent matching presenter is actually on the list.
That lengthening a watch list may reduce confidence in a match speaks against the promiscuous searching of large databases for individuals with low probability of being on the list, and it tells us that we must be extremely careful when we increase “interoperability” between databases without control over whether increasing the size of the list has an impact on the probability that the search subject is on the list. Our response to the apparent detection of a person on a list should be tempered by the size of the list that was searched. These lessons contradict common practice.
The designers of a biometric system face a challenge: to design an effective system, they must have an idea of the base rate of detection targets in the population that will be served by the system. But the base rate of targets in a real-world system may be hard to estimate, and once the system is deployed the base rate may change because of the reaction of the system’s potential detection targets, who in many cases will not want to be detected by the system. To avoid detection, potential targets may avoid the system entirely, or they may do things to confuse the system or force it into failure or backup modes in order to escape detection. For all these reasons, it is very difficult for the designers of the biometric system to estimate the detection target base rate accurately. Furthermore, no amount of laboratory testing can help to determine the base rate. Threat modeling can assist in developing estimates of imposter base rates and is discussed in the next section.
SECURITY AND THREAT MODELING
Security considerations are critical to the design of any recognition system, and biometric systems are no exception. When biometric systems are used as part of authentication applications, a security failure can lead to granting inappropriate access or to denying access to a legitimate user. When biometric systems are used in conjunction with a watch list application, a security failure can allow a target of investigation to pass unnoticed or cause an innocent bystander to be subjected to inconvenience, expense, damaged reputation, or the like. In seeking to understand the security of biometric systems, two security-relevant processes are of interest: (1) the determination that an observed trait belongs to a living human who is
present and is acting intentionally and (2) the proper matching (or non-matching) of the observed trait to the reference data maintained in the system.
Conventional security analysis of component design and system integration involves developing a threat model and analyzing potential vulnerabilities—that is, where one might attack the system. As described above, any assessment of the effectiveness of a biometric system (including security) requires some sense of the impostor base rate. To estimate the impostor base rate, one should develop a threat model appropriate to the setting.30 Biometric systems are often deployed in contexts meant to provide some form of security, and any system aimed at security requires a well-considered threat model.31 Before deploying any such system, especially on a large scale, it is important to have a realistic threat model that articulates expected attacks on the system along with what sorts of resources attackers are likely to be able to apply. Of course, a thorough security analysis, however, is not a guarantee that a system is safe from attack or misuse. Threat modeling is difficult. Results often depend on the security expertise of the individuals doing the modeling, but the absence of such analysis often leads to weak systems.
As in all systems, it is important to consider the potential for a malicious actor to subvert proper operation of the system. Examples of such subversion include modifications to sensors, causing fraudulent data to be introduced; attacks on the computing systems at the client or matching engine, causing improper operation; attacks on communication paths between clients and the matching engine; or attacks on the database that alter the biometric or nonbiometric data associated with a sample.
A key element of threat modeling in this context is an understanding of the motivations and capabilities of three classes of users: clients, imposters, and identity concealers. Clients are those who should be recognized by the biometric system. Impostors are those who should not be recognized but will attempt to be recognized anyway. Identity concealers are those who should be recognized but are attempting to evade recognition. Important in understanding motivation is to envision oneself as the
30 |
For one discussion of threat models, see Microsoft Corporation, “Threat Modeling” available at http://msdn.microsoft.com/en-us/security/aa570411.aspx. See also Chapter 4 in NRC, Who Goes There? Authentication Through the Lens of Privacy (2003). |
31 |
The need to consider threat models in a full system context is not new nor is it unique to biometrics. In his 1997 essay “Why Cryptography Is Harder Than It Looks,” available at http://www.schneier.com/essay-037.html, Bruce Schneier addresses the need for clearly understanding threats from a broad perspective as part of integrating cryptographic components in a system. Schneier’s book Secrets and Lies: Digital Security in a Networked World (New York: Wiley, 2000) also examined threat modeling and risk assessment. Both are accessible starting points for understanding the need for threat modeling. |
impostor or identity concealer.32 Some of the subversive population may be motivated by malice toward the host (call the malice-driven subversive data subject an attacker), others may be driven by curiosity or a desire to save or gain money or time, and still others may present essentially by accident. This mix would presumably depend on characteristics of the application domain:
-
The value to the subversive subject of the asset claimed—contrast admission to a theme park and physical access to a restricted research laboratory.
-
The value to the holder of the asset to which an attacker claims access—say, attackers intent on vandalism.
-
The ready accessibility of the biometric device.
-
How subversive subjects feel about their claim being denied or about detection, apprehension, and punishment.
A threat model should try to answer the following questions:
-
What are the various types of subversive data subjects?
-
Is it the system or the data subject who initiates interaction with the biometric system?
-
Is auxiliary information—for example, a photo ID or password—required in addition to the biometric input?
-
Are there individuals who are exempt from the biometric screening—for example, children under ten or amputees?
-
Are there human screening mechanisms, formal or informal, in addition to the automated biometric screening—for example, a human attendant who is trained to watch for unusual behavior?
-
How can an attack tree33 help to specify attack modes available to a well-informed subversive subject?
-
Which mechanisms can be put in place to prevent or discourage repeated attempts by subversive subjects?
Here are some further considerations in evaluating possible actions to be taken:
32 |
This is often referred to as a “red team” approach—see, for example, the description of the Information Design Assurance Red Team at Sandia National Laboratories, at http://idart.sandia.gov/. |
33 |
For a brief discussion of attack trees, see G. McGraw, “Risk Analysis: Attack Trees and Other Tricks,” August 1, 2002. Available at http://www.drdobbs.com/184414879. |
-
Will the acceptance of a false claim seriously impact the host organization’s mission or damage an important protected resource?
-
Have all intangibles (for example, reputation, biometric system disruption) been considered?
-
How would compromise of the system—for example, acceptance of a false claim for admission to a secure facility—damage privacy, release or degrade the integrity of proprietary information, or limit the availability of services?
In summary, as discussed at length above, FMRs and FNMRs in themselves are insufficient to describe or assess the operational performance of a biometric system and may be seriously misleading. It is necessary to also anticipate the fraction of reported matches that are likely to be true matches and the fraction of reported nonmatches that are likely to be true nonmatches. The analysis above shows that these will vary greatly with the base rate of impostors presenting to the system. The base rate should be estimated using one or more reasonable threat models. Biometric system design should then incorporate this information, as well as the costs and/or utilities of actions resulting from true and false matches and nonmatches.
All information systems are potentially vulnerable, but the design of biometric systems calls for special considerations:
-
Probabilistic recognition. This fundamental property of biometric systems means that risk analysis must recognize that there is a probability of making incorrect recognitions (positive or negative). If an attacker can gain access to a large-scale biometric database, then he or she has the opportunity to search for someone who is a biometric doppelganger—someone for whom there is a close enough match given the target false match rate for the system.34
-
Exposure of biometric traits. This can occur either through direct observation or through access to biometric databases. It allows an attacker to create fraudulent copies of those traits to be used in an attempt to mislead a biometric sensor.35
-
Concealment of biometric traits. In some applications, such as those intended to prevent multiple enrollments or to identify persons on watch lists, an attacker can avoid detection by concealing biometric traits through relatively simple actions.36
These considerations show the importance of how a sample is presented. Since biometric data must be thought of as public information, a system must take appropriate precautions to verify that the sample presented belongs to the individual presenting the sample and that the individual is voluntarily presenting himself or herself for identification. In some cases, this may mean supervised recognition; in others it may mean that a technical mechanism is employed to validate the sensor and that the sensor can differentiate genuine samples from fraudulent synthesized samples. In the latter case, an appropriate sensor takes the place of a human observer of the presentation ceremony.
In response to growing concerns over identity theft and fraud, some advocates suggest that legislation be enacted to prohibit the selling or sharing of an individual’s biometric data.37 By making it illegal to traffic in biometric data and by requiring encryption for the storage of biometric data in authentication systems, the hope is that the chance of inappropriate data disclosure will be reduced significantly, preserving the utility of biometric authentication for widespread use. However, the encryption of data does not guarantee that the underlying data will not be exposed. Furthermore, covert observation of many biometric traits is possible, making acquisition of these data hard to avoid. Accordingly, the more ubiquitous biometric systems become, the more important it is that each system using biometrics perform a threat analysis that presumes public knowledge of a subject’s biometric traits. Those systems should then deploy measures to verify that the presentation ceremony is commensurate with the risk of impersonation.38 Furthermore, in high-assurance and high-criticality
in Advances in Biometrics, Massimo Tistarelli and Mark S. Nixon, eds., Third International Conference, Alghers, Italy (2009). |
36 |
Sometimes identity is concealed using more radical techniques. Recently it was reported that a Chinese national had had her fingerprints surgically transferred from one hand to another to avoid recognition by Japanese border control. Available at http://mdn.mainichi.jp/mdnnews/news/20091207p2a00m0na010000c.html. The Interpol fingerprint department provides a historical perspective on fingerprint alteration at http://www.interpol.int/Public/Forensic/fingerprints/research/alteredfingerprints.pdf. |
37 |
P. Swire, “Lessons for biometrics from SSNs and identity fraud,” presentation to the committee on March 15, 2005. |
38 |
Note, for instance, the recent shutdown of the “Clear” air traveler program by Verified Identity Pass. The company held personal information, including biometric information, on thousands of individuals. At the time of this writing, a court had enjoined the company from selling the data. |
applications, biometric recognition should not be the sole authentication factor.
ON REPORT SCOPE AND BOUNDARIES
This report explores the strengths and limitations of biometric systems and their legal, social, and philosophical implications. One core aim of the report is to dispel the common misconception that a biometric system unambiguously recognizes people by sensing and analyzing their biometric characteristics. No biometric technology is infallible; all are probabilistic and bring uncertainty to the association of an individual with a biometric reference, some of it related to the particular trait being scrutinized by the system. Variability in biometric traits also affects the probability of correct recognition. In the end, probability theory must be well understood and properly applied in order to use biometric systems effectively and to know whether they achieve what they promise.
This report does not address whether a biometric system is the best way to meet a particular application goal. It does not compare biometric technologies with potential alternatives for particular applications, because such alternatives would have to be evaluated case by case. This chapter has reviewed the fundamental properties of biometric systems. Chapter 2 will offer a framework for considering the requirements of an application from the engineering standpoint. Chapter 3 outlines lessons learned from other types of systems. Chapter 4 examines the social, cultural, and legal issues related to biometric systems. Finally, Chapter 5 summarizes the research challenges and open questions identified in the earlier chapters.