7
Innovations in Antimicrobial Resistance Surveillance Tools and Technologies
The sixth session of the workshop explored nontraditional approaches to antimicrobial resistance (AMR) research, including community science, simulation modeling, and network modeling. Jeff LeJeune, food safety officer in the Food Systems and Food Safety Division of the Food and Agriculture Organization of the United Nations, moderated the session. Wieland Meyer, deputy head of the Curtin Medical School and associate dean of Biomedical Science, Pharmacy, and Medical Radiation Science at Curtin University, Perth, Australia, described community science research currently being conducted on Aspergillus fumigatus azole resistance in Latin America. Brian Bailey, associate professor of plant sciences at University of California, Davis, discussed how simulation models can increase the pace of response to emergent threats and optimize current resistance management practices. Karen Garrett, Preeminent Professor of plant pathology and the Institute for Sustainable Food Systems and Emerging Pathogens Institute at the University of Florida, provided an overview of dynamic network models, impact network analysis, and applications for antifungal resistance research.
ASPERGILLUS FUMIGATUS AZOLE RESISTANCE SURVEY BY AIR SAMPLING THROUGH A COMMUNITY SCIENCE APPROACH
Meyer described the impetus and methodology of a study that he and colleagues are currently conducting on A. fumigatus azole resistance in Latin America utilizing a community science approach. Azole resistance research is not geographically uniform, and data from Latin America are limited, he said. A Brazilian study examined 199 isolates in a clinical collection
and located two azole-resistant isolates with mutation in the cyp51A gene (Pontes et al., 2020). A study in Peru also examined a clinical collection and among 143 A. fumigatus samples, 3 were azole-resistant (Bustamante et al., 2020). Mexican research on Aspergillus spp. detected 49 A. fumigatus isolates, 6–10 percent of which were azole-resistant (Trevino-Rangel et al., 2021). A larger study compared samples from Mexico, Paraguay, and Peru with those from African countries, and researchers found a variable resistance rate between 7–10 percent in the Latin American countries (Resendiz-Sharpe et al., 2021).
Resistance Survey Project Objectives
Meyer remarked that he and his colleagues responded to a call by the U.S. Centers for Disease Control and Prevention for data on the prevalence of antifungal resistance in A. fumigatus in low- and middle-income countries (LMIC). He acknowledged Matthew Fisher, professor of Fungal Disease Epidemiology at Imperial College London, for pointing him toward this opportunity. His team developed a project to “evaluate the presence of azole-resistant A. fumigatus isolates in air samples in Latin America.” He noted that the ease with which air samples can be collected factored into the project design. Once collected, azole-resistant A. fumigatus isolates from the samples will be assessed for the presence of mutations in the cyp51A gene. Researchers will compare genetic relationships between resistant strains found in different Latin American countries. Additionally, they will examine environmental sampling and azole agricultural usage data to determine whether a correlation exists between resistant isolates detected and azole fungicide use. The project is also an opportunity to establish a network of medical mycologists in Latin America to monitor resistant Aspergillus and conduct future studies, he added.
Latin American Medical Mycology Network Laboratories
During previous research on Cryptococcus isolates in Latin America, Meyer and colleagues created a loose network of researchers with expertise in medical mycology, infectious diseases, environmental sampling, strain isolation, and molecular characterization of isolates and epidemiology. The group served as a basis for the Latin American Medical Mycology Network (LAMMN), a network of laboratories established for the Aspergillus project. Comprised of 26 laboratories, LAMMN spans across Mexico, Colombia, Venezuela, Brazil, Paraguay, Uruguay, Argentina, Chile, Peru, Ecuador, Costa Rica, and Guatemala. Meyer noted organizational challenges in working with such a large network in which three languages—Spanish, Portuguese, and English—are spoken. Although English serves as
the common language, many of the researchers have limited English skills. Moreover, considerable organizational difficulties arose from the varied regulations and postal systems among the countries involved. In addition to the Latin American partners, the project aims to include isolates collected from Antarctica. Meyer remarked that Antarctica is one of the most pristine environments on earth in terms of limited human populations. Thus, knowing whether Aspergillus is found there would add to data on the reach of these fungi. He added that Australia and Brazil are contributing molecular genotyping expertise to the project.
Among the LAMMN laboratories, four were selected to serve as reference laboratories responsible for collecting samples from four regions determined by the historical strength of networking between institutions, Meyer explained. The State University of Campinas (Universidade Estadual de Campinas) in Brazil is the LAMMN reference laboratory for institutions in Brazil, Argentina, and Venezuela. Pontifical Javierian University (Pontificia Universidad Javeriana), Colombia, serves as the reference laboratory for Colombia, Ecuador, Paraguay, and Uruguay. Cayetano Heredia University (Universidad Peruana Cayetano Heredia) in Peru is the reference laboratory for Peru and Chile, and the National Autonomous University of Mexico (Universidad Nacional Autónoma de México) represents Mexico, Costa Rica, and Guatemala.
Project Design
Meyer and his team based their project on the community science approach utilized in Jennifer Shelton’s1 research described by Fisher in Chapter 4 (Shelton et al., 2020), recognizing this simple method would enable coverage of a large part of Latin America. Structured into two levels, the first half of the project involves collecting 200 samples from each participating country four times in 1 year. Two samples are collected from each participant—taken at a distance of approximately 1 kilometer from one another—necessitating 100 participants per country. All samples are sent to one of the four above specified reference laboratories, which is then responsible for identifying resistant isolates via microbiological culture and minimum inhibitory concentration (MIC) testing. All resistant isolates are then sent to Fundação Oswaldo Cruz laboratories for further genotyping.
Participant recruitment for sampling efforts began in November 2021. Meyer acknowledged this process proved to be more challenging than expected. Specific social media feeds were used to encourage participants
___________________
1 As described in Chapter 4, Jennifer Shelton, applications scientist at Oxford Nanopore Technologies, developed a citizen science approach to collecting data samples during her doctoral research at Imperial College London.
to register via Twitter, Facebook, and Instagram, but he found that word-of-mouth appears to have contributed more to participant recruitment than the social media campaign. A website was created for registration, a challenging endeavor due to the need to translate the content from English to Spanish and Portuguese in order to make it available in three languages.2 When a participant registers online, they are first directed to complete a registration form that collects contact information. Next, they are given instructions on how to receive the sampling kit, with options to have it mailed to a personal address or pick a kit up in person from one of the participating institutions. The form collects location information where the participant will be collecting a sample and includes a field to indicate whether it is a rural or urban setting.
As of June 21, 2022, approximately 1,600 participants had registered, with roughly half recruited via the webpage and social media and the other half recruited directly by participating laboratories. Registered participants represent all 12 countries in the survey, constituting a substantial percentage of coverage of Latin America. Not surprisingly, areas such as the Amazon rainforest are under-sampled, so efforts are being made to increase coverage of these locations. Meyer noted that baseline data generated in this project will largely cover the entire South American continent. During the summer of 2022, State University of Campinas is preparing sampling kits to ship to the laboratories that correspond geographically to the participants. The kits are pre-labeled with the names and home addresses of those participants receiving them by mail or with the names and institutions of those picking them up in person.
Meyer described that each kit contains supplies for two samples, which participants will use and send them back to the appropriate national laboratory, which in turn will send the samples to the corresponding reference laboratory for isolation (see above). Given the 1,600 participants and four testing periods over the course of a year, 12,800 sampling kits must be prepared and distributed. Each sampling kit includes an information leaflet with the objectives of the study, sampling instructions, a form for recording the sampling conditions, two air sampling adhesive films, envelopes to place the samples in, and a larger prepaid envelope for returning both samples to the laboratory. Organizational challenges required rescheduling the collection dates several times. In addition, the sample strips used in both a preliminary study and in Shelton’s research were determined to be cost prohibitive and in short supply due to supply chain and shipping issues in South America. Thus, a locally sourced sticky film tape was evaluated and selected as a substitute. Meyer and colleagues confirmed that this less
___________________
2 The Latin American Aspergillus fumigatus Azole-Resistance Survey website is available at https://www.latasp.com/ (accessed August 15, 2022).
expensive material has the same performance as polymerase chain reaction (PCR) plate covers used as the adhesive collection film in Shelton’s study. The first round of sampling kits was mailed to participants in June 2022, with collection taking place during the second week of July 2022. The collection process will repeat every 3 months, concluding in April 2023.
Sampling and Analysis Processes
The materials for sample collection feature a circle the size of a petri dish with a sticky arm on each side. The participant peels the supply paper off the back of the arms and uses them to adhere the samples to windowsills in two different locations for an exposure of 10–12 hours, Meyer noted. The participants then recover the sampling film and mail the samples in sterile envelopes to the laboratories. Each of the four reference laboratories will culture the collected material to look for A. fumigatus, and all isolates that look morphologically similar to A. fumigatus will be confirmed via matrix-assisted laser desorption/ionization-time of flight (i.e., MALDI-TOF) or beta tubulin (i.e., β-TUB) sequencing, depending on the reference laboratory’s facilities. The A. fumigatus isolates will then be screened for resistance against itraconazole and voriconazole. The reference laboratories will send resistant isolates to either the Federal University of Mato Grosso do Sul (Universidade Federal de Mato Grosso do Sul) or Adolfo Lutz Institute (Instituto Adolfo Lutz), both in Brazil, where MIC testing will be conducted against a wider range of azoles. Resistance isolates showing high MICs will be sent to State University of Campinas or Nantes University in France for amplification of the cyp51A gene regions. Depending on the number of isolates available, microsatellite analysis or whole genome sequencing will be carried out at Nantes University (Nantes Université), the Oswaldo Cruz Foundation (Fundação Oswaldo Cruz) in Brazil, or Curtin University in Australia. These data will enable comparisons between isolates found in Latin America and those in other parts of the world. In a parallel process, the LAMMN laboratories are collecting agricultural azole usage data to determine whether a correlation exists between agricultural azole usage and the azole-resistant isolates found in the same region.
Proposed Project Impact
Meyer emphasized that as of June 2022, the project has only been under way for 6 months, and no data are yet available to present. However, preliminary studies indicated A. fumigatus isolates in a number of locations using the proposed methodology. The project has several aims.
- Provide baseline data on environmental A. fumigatus resistance throughout Latin America.
- Provide Latin American governments with data to identify links between agricultural antifungal usage and emerging resistance in environmental A. fumigatus isolates.
- Provide evidence to governments to influence the usage of antifungals in agriculture.
- Provide the World Health Organization with baseline information on A. fumigatus azole resistance to develop better treatment guidelines.
- Enable decision-making for clinical treatment of invasive aspergillosis between monotherapy with a first-line triazole or a combination therapy of a triazole with an echinocandin or liposomal amphotericin B in cases with environmental triazole-resistance prevalence greater than 10 percent, as recommended by earlier studies (Verweij et al., 2015).
Although the study is at an early stage, Meyer shared his optimism about this project, given that it has already overcome organizational challenges regarding varying government regulations, work in remote areas, and complications with the different postal systems involved. Furthermore, the number of participants enrolled—from government employees to community scientists learning of the project online—is encouraging, he said. A growing global knowledge of resistance in the environment will inform whether a link between resistance and agricultural azole usage is at play.
INNOVATION IN DATA COLLECTION TOOLS FOR SURVEILLANCE AND MITIGATION: CROP AND ENVIRONMENT SIMULATION TOOLS
Bailey discussed simulation models that could aid in creating more efficient fungicide management programs to reduce the development of resistance and respond more rapidly to novel fungal pathogen threats. He noted that changing conditions can transform a pathogen that historically has not posed problems to humans into both a food security and human health threat. Rapid response to changing pathogens can minimize these negative effects.
Trial-and-Error Versus Simulation Modeling
Various mitigation and management strategies are used to disrupt the fungal disease cycle in agricultural settings, said Bailey (see Figure 7-1). Most of these strategies center around disrupting pathogen development
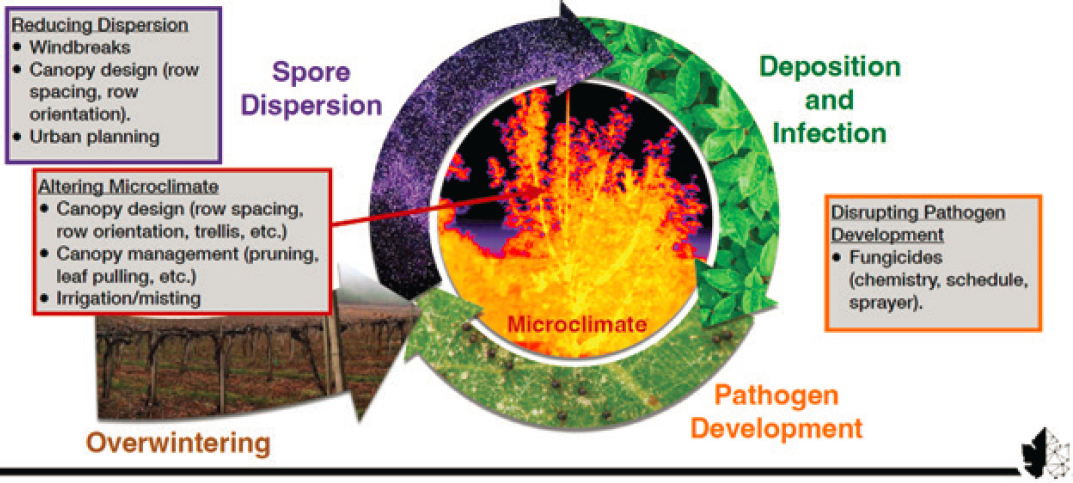
SOURCE: Bailey presentation, June 22, 2022.
and include (1) applying fungicide, (2) alternating the chemicals used, (3) rotating crops, (4) shifting to a different mode of action, and (5) altering the schedule application method. A single change in the system carries the potential to affect the entire fungal life cycle. Traditionally, determining the most effective management practices has been a process of trial and error. Prior knowledge about existing diseases is utilized as a foundation in developing modified practices for new threats. Experiments and approaches are conducted and evaluated under changing field conditions, though some experiments may first take place in the laboratory before moving into field trials. An iteration process continues until an acceptable outcome (e.g., efficacy of crop protection) is achieved. Bailey remarked on the time-consuming nature of this traditional experimentation approach. Testing a method under various weather conditions and other pertinent factors can require several seasons to establish sufficient confidence in the method to translate into production. Additionally, the efforts may converge toward a local optimum rather than to a global one.
In contrast, industries outside of agriculture are using simulation and modeling approaches to accelerate discovery and innovation, Bailey stated. For example, a design engineer creating a component of a jumbo jet would likely use a simulated environment where all variables can be controlled, thus enabling rapid iteration through various designs. These designs would be tested through realistic, physically based models to determine the critical variables. Only a satisfactory prototype would be built to test in a wind tunnel, and then progressing to build a full-scale aircraft. Models and simulations have accelerated the pace at which many industries can optimize designs and bring products to market. Furthermore, simulations can test variables that are not readily measurable in the physical world.
Utilizing simulation technology in agriculture—and specifically in fungicide resistance—could bypass much of the trial-and-error process, said Bailey. In silico iteration could enable all the various parameters to be tested simultaneously, thus narrowing down to the most promising methods to test in the field. This simulation loop could potentially decrease experimentation time, control for different conditions, and increase the speed of response to an identified problem. An ideal scenario would include a field experiment, but in situations requiring immediate response, the method could potentially move directly from simulation to rollout.
Resistance Modeling Considerations and Model Types
Bailey acknowledged challenges inherent in simulating a system of AMR, given that it entails numerous variables that all interact with one another. This system must consider many potentially significant processes and inputs, a number of which are not readily acquirable for a given site
or under certain conditions. Additionally, researchers may want to investigate multiple mitigation strategies. AMR modeling needs to be capable of incorporating relevant inputs, determining how the mitigation strategies interact with each other, and predicting outputs ranging from fungicide resistance to efficacy in controlling disease to human pathogen exposure. Moreover, model parameterization requires experimental data in which simultaneous measurements of important sub-processes are collected (see Figure 7-2). Bailey remarked that a potentially beneficial initial approach to this complex task might be selecting a few prioritized inputs and conducting a limited number of experiments to evaluate them.
Empirical, Mechanistic, and Heuristic Models
The level of detail needed to create a helpful model can vary depending on specific needs. Bailey explained that determining which variables to include depends on the particular problem being investigated and the end goal. Different types of models can be utilized based on specific needs. Empirical models use observed data trends to infer relationships between variables. An advantage of empirical models is that all of the mechanisms at play do not necessarily need to be understood in order to use them. These models only require that variables can be measured as they change within a dataset, and that a set of mathematical equations can be determined that describe the observed trends. However, empirical models typically require substantial amounts of data; more variables require larger amounts of data to cover the parameter space.
Mechanistic models, guided by laws of nature rather than trends in datasets, are used to quantify relationships between variables. Bailey noted that no model is fully mechanistic because empirical parameterizations are needed at some point. In many cases, mechanistic models offer the advantage of requiring less data, given that the laws of nature constrain some of the relationships between variables. In theory, these models are more robust when conditions change because they do not require complete datasets in order to represent every relationship. Mechanistic models also have the potential to be more robust when applied to future events because they do not necessarily require data on conditions that have not yet occurred. A downside to these models is the need for theoretical understanding of how systems work, which can be challenging to achieve in life sciences, biological systems, and agriculture. For this reason, researchers in life science tend to use models that are more empirical in nature rather than mechanistic ones, said Bailey.
Heuristic models describe relationships more generally between variables, with trends typically reproducible. Such models may be utilized when data are insufficient to develop an empirical model—for example,
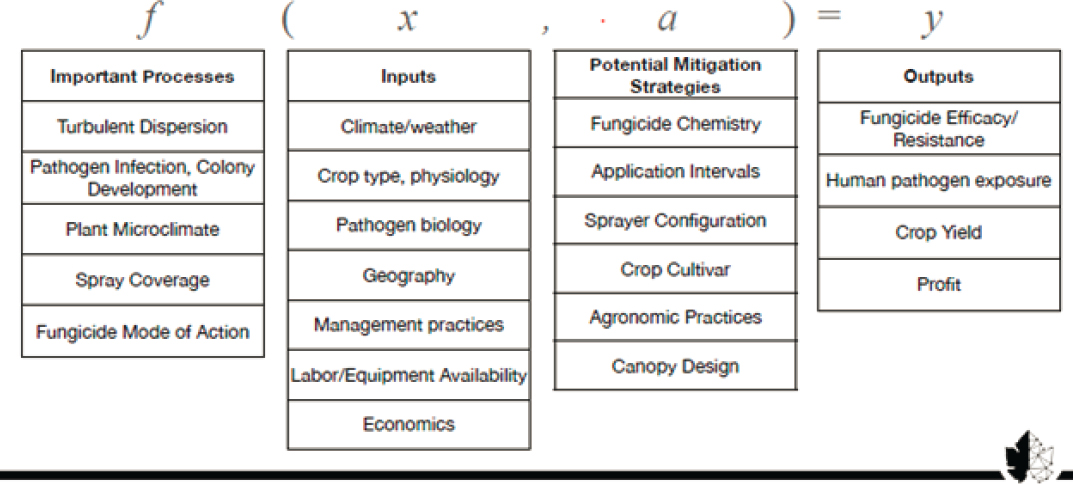
SOURCE: Bailey presentation, June 22, 2022.
limited data may be available about an epidemic. Bailey remarked that disease data are often sparse in comparison to other fields. However, automated and community science approaches to data collection are efforts to address gaps in disease data.
Helios Modeling Framework
Most models for antifungal resistance target one or two phases of the fungal pathogen cycle (see Figure 7-1), said Bailey. For instance, the host is generally not addressed due to the assumption that susceptible host tissue is abundant and does not limit disease development. The environmental effects of microclimate are often ignored; even in cases where ambient weather data are considered, the differences between weather and the actual microclimate that the pathogen is experiencing are often neglected, Bailey stated. He and colleagues have been working in recent years to develop Helios, a scalable, three-dimensional (3D) simulation framework for plant and environmental modeling. Helios combines all processes together in a systems level model with a mechanistic approach. For example, the model is able to predict the local microclimate at the pathogen and tissue level and the evolution of the host based on the local microenvironment. The model is also able to resolve impacts of changing the canopy, geometry, or architecture, and it can determine effects at the local pathogen scale in terms of colony development or spread across tissue. Bailey said that work is under way to incorporate fungicide aspects to enable simulated fungicide application. This could make it possible to predict the effect of ultraviolet exposure on chemicals, the trajectory of efficacy decline over time, expected levels of fungicide resistance by genotype, and the likelihood of resistance evolving throughout and beyond the agricultural system to potentially affect human health.
Artificial Intelligence Modeling
Bailey stated that machine learning and other types of artificial intelligence (AI) models are steadily gaining in popularity. He described machine learning as the “ultimate empirical model,” requiring massive amounts of data to extract trends and make predictions for the future. Agricultural settings—and plant pathology specifically—present substantial challenges in applying machine learning models; collecting adequate data for simplified empirical models is already difficult and AI-based models require significantly larger quantities of data. Bailey clarified that AI uses in agricultural modeling are possible, depending on the data source and desired output. This modeling is commonly used for high throughput screening. Multispectral, thermal, lidar, and red-green-blue (RGB) color imaging techniques
can be used to detect plant stress caused by disease (Oerke et al., 2014). Therefore, AI modeling could use these images to infer what is taking place within the plant images as various conditions change. Bailey noted that such models are not diagnostic per se: For example, they may indicate stress in the plant without providing a diagnosis. Practitioners would then need to conduct more intensive sampling to determine which pathogen is affecting the plant. Furthermore, AI may not be able to detect problems until the signature symptoms of the disease present, at which point it could be too late to treatment.
Simulated Data
When sufficient data are lacking, simulated data can be used as a supplement in developing AI-based models, said Bailey. For example, machine learning models have been used to test self-driving cars in environments created with simulated data. These detailed simulated environments enable researchers to vary a wide range of parameters. A simulated data approach can also be applied to agricultural environments. Bailey and colleagues developed an initial test case for fruit detection in which they generated a 3D simulation that contained numerous images of the crop in various conditions (Fei et al., 2021). The simulation allows for every pixel within an image to be auto-labeled and used to train the machine learning model. Bailey is hopeful that simulated data modeling will lead to applications relevant to disease detection and management. For instance, generated 3D models could predict multiple factors affecting the system, simulate an intervention—such as changing the way that plants interact with light—and generate large amounts of data, which would then train a machine learning model that would be applied to agricultural practices in the real world. He added that this technology could potentially extend beyond detecting disease to inferring risk or areas likely to have fungicide resistance. Scaling this technology could enable the prediction of human exposure to resistant fungi. Bailey emphasized that field-level disease data are often sparse in agricultural environments, and alternative modeling methods are needed to accelerate the pace of response to emergent threats and for optimization of current practices.
IMPACT NETWORK ANALYSIS FOR GUIDING SURVEILLANCE AND MITIGATION
In a prerecorded presentation, Garrett introduced the general concept of dynamic network models and described impact network analysis and applications for antifungal resistance research. Garrett remarked that “scenario analysis allows users to consider likely outcomes from potential
mitigation strategies.” For instance, a manager weighing various options can utilize scenario analysis to determine the costs and benefits of different approaches. A socioecological approach integrates the process of human decision-making into a platform to assess human influence on the management of factors such as potential pesticide resistance.
Dynamic Network Models
Network models consist of nodes and links that connect them, Garrett explained. The links between pairs of nodes can be weighted in relation to the level of interaction between the nodes. In many systems, these link weights are of a dynamic nature in that they can change over time. Possibilities for network model structures are infinite. The nodes can be locations in spatial networks, such as counties, fields, plants, leaves, cells, organelles, or microbes. Nodes can also be entities like individual people, species, genes, or molecules. The links between the nodes can indicate the strength or likelihood of interaction; these links are dependent on environmental variables. In the context of potential movement of pesticide resistance genes, nodes could include individual human hosts and farms, while the links could indicate the probability of movement of pesticide resistance genes between the people and farms (e.g., through spread of resistance microbes from one location to another).
A network has several traits and features that, once characterized, can be used to draw conclusions about the importance of different nodes in the network, said Garrett. The structure of a network can be socioeconomic or biophysical. A socioeconomic network contains nodes that represent people or human institutions. In the context of antifungal resistance, a socioeconomic network could contain nodes representing farmers, extension agents, and scientists. A biophysical network features nodes that represent geographic locations, such as individual plants, farms, storage facilities, and wildlands. Once the structure of a network is established, conclusions can be drawn about the nodes from several aspects related to centrality. Degree centrality is the number of links connected to a node. All else being equal, the more links a node has in the network, the greater the likelihood that the node is important, she remarked. Closeness centrality measures how readily other nodes can be reached. Betweenness centrality refers to how often a node serves as a bridge between other nodes. Centrality of neighbors ranks nodes as more important when they have proximity to neighboring nodes of importance.
Garrett described how she and colleagues used networks to characterize potential movement of pesticide resistance and other traits in grain storage (Hernandez Nopsa et al., 2015). Managers of grain storage facilities make decisions that can affect the likelihood that fungi using the grain as a
substrate will select for resistance. Once pesticide resistance is established at one location, it can spread to other facilities. A characteristic network of wheat movement by U.S. railroads aids in identifying locations that are prime for transmitting spread. The network used in this study includes nodes for each U.S. state, proportionally sized to wheat production in that state. Links between nodes represent grain volume transported between locations; the thicker the weight of the link, the greater the volume of grain moving between two states. The network model revealed that Illinois—a state that is not one of the top grain producers—serves as a key hub in wheat transportation. Thus, managing pesticide resistance in Illinois locations connected to this transport system is important in preventing spread, said Garrett.
Networks can also be used in the context of agricultural lands. Garrett was involved in research on cropland connectivity that used gravity models to predict the probability of a pest or pathogen moving from one location to another (Xing et al., 2020). She noted that these models could also be used to predict spread of pesticide resistance genes. The gravity model factored in the abundance of host crop available at both locations, the distance between them, and the dispersal patterns of the pathogen species that is potentially spreading. This example demonstrates that networks can be used to predict risk of movement, including risk of movement through landscapes. These types of data can be used in the context of impact network analysis (INA).
Impact Network Analysis
Garrett noted her work on INA and the associated R program in various contexts including problems, such as pathogen movement, and benefits, such as the spread of improved crop varieties.3 The networks can be characterized based on observational data or in terms of potential scenarios. She described using INA to evaluate management technologies for stored grain that are currently available for adoption, including strategies that are less likely to lead to fungal selection for pesticide resistance but may have potential trade-offs in cost (Garrett, 2021). Managers are faced with a decision of whether a lowered risk of pesticide resistance is worth the additional cost. She created a socioeconomic network to represent the exchange of information among decision-makers, with each node representing people forming an opinion about this tradeoff of extra cost for decreased resistance risk. A biophysical network was created to represent the dispersal of
___________________
3 The R program is an open-source software suite used for data manipulation, calculation, and graphical display in statistical computing and graphics. More information about the R program is available at https://www.r-project.org/about.html (accessed August 18, 2022).
a focus bioentity—in this case, pathogens with resistance genes—and how management adoption influences the establishment of the bioentity. In order to define a management scenario, INA enables various components of these networks to be adjusted to arrive at potential outcomes. For example, INA can be used to predict the likelihood that a policy to subsidize better management strategies will be successful in decreasing the spread of pesticide resistance.
Scenario analysis with INA technology facilitates exploration of questions of interest, said Garrett. For example, it can provide insight on key locations of focus for antibiotic resistance management efforts. INA can also indicate the influence of subsidies and policies on system outcomes. For instance, INA could develop scenarios for both a policy that subsidizes improved management systems and one that imposes a penalty if management strategies are not implemented. The outcomes of these approaches could then be compared to see which is more likely to decrease the risk of pesticide resistance. INA can also be used in informing surveillance strategy. Garrett created networks to represent the potential spread of an invasive bioentity, such as pesticide-resistant genes (Garrett, 2021). The nodes in these networks represent individual points of potential spread of pesticide resistance. INA generated various scenarios, with nodes shaded by color to represent their potential value in surveillance efforts. These scenarios then inform which points of potential spread should be prioritized for surveillance and management efforts. Garrett acknowledged that small systems may not require INA to achieve these insights. However, INA can be helpful in representing scenarios for systems that are too large to visualize in traditional ways. INA can also be used to determine target management adoption rates in the event of increased probability of the establishment of resistant genes (Garrett, 2021). For each percentage of increased probability of establishment, INA can generate the mean adoption rate required to keep the risk of resistance from increasing. This information can inform the level of intervention needed to maintain the sustainability of a system when one factor is disrupting the balance.
Garrett emphasized that complete analysis via modeling methods demands large volumes of data. When specific data are insufficient, more general questions can inform the process of characterizing the system. For example, researchers can consider how a change in the impact network components can maintain system stability and account for increased risk (Garrett, 2021). As additional data are collected, models can become more realistic. At this point, researchers can consider how changes in network traits, including mechanisms of influence between actors, affect system outcomes. Once more information is known, questions can become more precise, such as identifying specific decision-makers or location nodes as “key control points for successful management.”
DISCUSSION
Data Considerations in Simulation Modeling
Given that real world data may be lacking, LeJeune asked how models can be validated under that circumstance. Bailey replied that the data requirement is often substantially lower for validation than it is for developing an empirical model from the start. He acknowledged that the more available data will support stronger validation. However, validation can be performed by targeting specific inputs and outputs of different scenarios, without necessarily measuring every subprocess. In instances where data are lacking but physical relationships or theory are represented, direct validation may not be possible. In these cases, focus would be placed on input mapping to various outputs. Statistical approaches can also be used in which certain parameters or variables are treated as unknown, and the importance of random variables are assessed using sensitivity analyses.
LeJeune asked how robust these models are in terms of application—i.e., whether a new model needs to be developed for each pathogen and for each commodity. Bailey stated that fundamental inputs are needed in terms of different pathogens and crops, in addition to pests and microclimate features. His team worked to build the Helios model to be as modular as possible. Directly representing the 3D geometry of the system allows for a more generalizable framework. Different and more specific data are needed when moving from system to system. For example, data from fungal pathogen isolates may be required without needing other data from a full-scale field experiment. The model uses the specific pathogen-related data and essentially scales up to field scale. In other words, not all the components need to be remeasured each time a new model is developed. Bailey noted that the development of crop models has been limited by the amount of data on crop growth and yield needed to parameterize the model, as this can equate to decades’ worth of work. He and his colleagues are working to create generalizable models that facilitate the ability to move between systems and to predict future occurrences.
LeJeune asked how data gaps are identified and prioritized, given the number of variables involved in modeling fungal spread—including crop production, fungal growth, fungicide usage, pathogen emergence, and fungicide resistance. Bailey remarked that an advantage of this modeling approach is that a model can be initially formulated based on well-understood environmental interactions, and then used to determine the key variables. This determination enables the identification of narrowed and system-specific data needs. He added that current data gaps include grower management practices, both in terms of fungicide records and other practices. Complementary measurements on the local microclimate and crop
performance would also contribute to a more complete picture of what is occurring in the crop.
In response to LeJeune’s query about whether these models can be used to study A. fumigatus in waste management to determine strategies for a different portion of the fungi life cycle, Bailey replied that this has not been a priority for his research thus far, but it is important to many applications. His research framework would allow for that type of study, provided that data were available related to residues on plant waste. The local microclimate and dispersion from waste sites could be predicted; therefore, the framework should be able to accommodate this type of study.
Data Challenges in Community Science
LeJeune asked about the strengths and weaknesses of the community science approach to data collection and how these data can be validated to draw stronger conclusions from the results. Meyer responded that community science can involve some discrepancies. For instance, the random distribution of sites results in uneven representation across countries and across the continent. The collection method will also vary, with some people more carefully adhering to the instructions than others. Additionally, the lack of actual agricultural data could conceivably result in challenges, because participants may be willing to collect samples but unwilling to disclose the type of antifungal products being used, potentially out of concern about causing problems for their suppliers. He added that governments can also be hesitant about providing agricultural antifungal usage data. Meyer remarked that the project should provide baseline data that is currently lacking in the literature. Obtaining the isolates to establish genetic characterization across a continent is a challenge. Variance is expected in terms of accurate reporting of sampling position, climate and weather conditions, and description of the setting. LeJeune acknowledged the logistical and economic challenges in conducting a global survey. Meyer noted that he preferred to use the same type of adhesive film as the community science study in the United Kingdom, but the expense and supply logistics made this unfeasible. Locally produced film is affordable and available, but ensuring consistent quality over the duration of the project is a challenge. He stated that having more reproducible points from the same location would have been ideal for statistical confidence but this is difficult with the community science approach.
REFLECTIONS ON DAY TWO
LeJeune reflected that the workshop sessions explored the agricultural use of antifungal agents, the possible effects of that usage on human
medicine, the One Health aspects of that interplay, and the need to balance food security with the risks associated with AMR. That balance may be influenced by climate change and the economics of each country. Historical patterns of AMR related to occupational exposure in agriculture systems and the complexity of fungicide registration requirements were described, including the lengthy timeline involved in registering a new agent. Speakers discussed molecular epidemiology, molecular mechanisms of resistance, and global surveillance methods. Substantial agricultural and environmental data gaps were identified, and nontraditional community science and modeling approaches were detailed. Antifungal resistance is a global, multisectoral problem that will require input from plant and agriculture, human health, and surveillance communities, said LeJeune.