The third (October 2021) workshop began with a session focused on driving systems change by leveraging data that connect obesity with its underlying determinants. Carlos Crespo, professor at Oregon Health and Science University and Portland State University School of Public Health and vice provost for undergraduate training in biomedical research at Portland State University, moderated the session.
HOW DATA SCIENCE, ARTIFICIAL INTELLIGENCE, AND OTHER TECHNOLOGICAL APPROACHES CAN HELP ADDRESS THE SYSTEMS CONTRIBUTING TO OBESITY
Bruce Y. Lee, professor of health policy and management at the City University of New York School of Public Health, discussed opportunities for data science, systems modeling, artificial intelligence, and other types of technological approaches to improve how the complex systems that drive obesity are understood and addressed.
Lee offered a series of examples to illustrate what it would look like if society approached meteorology and weather forecasting in the same simplistic way that it approaches obesity prevention and control. Although these areas may seem unrelated, he pointed out that they both involve complex systems. These examples, he suggested, demonstrate the need to account for the entire system of factors when addressing the obesity epidemic.
First, Lee highlighted the need to consider a suite of indicators when trying to understand a complex system. Applying his analogy with meteorology, he pointed out that using a single measure, such as the temperature, to understand the weather would be inadequate because it would exclude information about such variables as precipitation and humidity that affect how a person prepares for the day’s weather. Similarly, Lee continued, it is insufficient to rely on single measures, such as an individual’s body mass index (BMI) or the population prevalence of obesity, to understand the broad set of factors that contribute to obesity and how best to intervene. He referenced a computational model that accounts for not only an individual’s BMI but also the person’s chronic health condition(s) to estimate specific disease outcomes and associated costs. This model can then be used
to estimate specific disease outcomes and costs that could be prevented by helping the patient transition from a BMI category of overweight or obesity to one of normal weight (Fallah-Fini et al., 2017). Multiple complex and dynamic measures provide a more complete picture of what is happening with a patient, he reiterated, compared with single measures.
Second, Lee explained the need for a combination of bottom-up and top-down approaches. In this example, he asked what would happen if simple associations and correlations were used to forecast longer-term changes in the weather. He shared a graph charting an increase in global average temperatures that has occurred over time alongside a decrease in the number of seafaring pirates. Lee pointed out that, although an association exists between global average temperatures and numbers of pirates, this association does not demonstrate that decreases in the number of pirates have caused the increases in global temperatures. He argued that top-down methods that involving scrutinizing data for patterns and correlations to detect potentially important factors should be used in combination with bottom-up methods that re-create systems to elucidate mechanisms of interest.
Third, Lee described the need to integrate data collection across different parts of a system. If a meteorologist collected data on barometric pressure but did not examine those data in the context of other measures and dynamics in the environment, many insights about the system’s interconnectivity would be missed. Data collected to support obesity prevention often occur in isolation from other activities focused on the same goal, which may result in mischaracterizing the system. A better approach, Lee suggested, is to integrate data collection, systems mapping and modeling, and policies and interventions to gain a holistic understanding of what is happening. Such insights can guide and prioritize subsequent data collection and intervention design, he added, which in turn generate more information that can be used to update maps and models in a circular, iterative process that gradually yields better solutions.
Lee’s fourth example focused on the importance of open data sharing. He asked the audience to think about the problems that would occur if weather data were not readily shared from location to location and people thus could not understand weather patterns in different parts of the country. Lee maintained that open sharing of data about the factors and processes that affect obesity at various scales and levels—genetics, physiology, individual behavior, social environments, physical environments, and societal forces such as policies—would help paint a more complete picture and reveal major forces driving the outcomes observed.
In his fifth example, Lee explained the value of understanding the variations among different locations. In meteorology, he said, it is illogical to assume that weather conditions in one location are the same everywhere,
as it is well known that weather varies geographically. The same is true with the obesity epidemic, he contended. Referring to a study by his group, Lee discussed how the effects of warning labels on sugar-sweetened beverages on the prevalence of obesity were found to vary by geographic location, reflecting differences in the environments studied (Lee et al., 2018).
Sixth, Lee discussed the importance of data surveillance systems that provide real-time updates. He asked the audience to imagine what it would be like if weather forecast systems were not regularly updated over time. In the same way that weather systems are based on immediately available information, Lee continued, decisions about obesity prevention and control need to be informed by real-time surveillance data because many of obesity’s contributing factors shift regularly.
Lee’s seventh example emphasized that external forces matter. He asked the audience to imagine what would happen if individuals were to be singularly blamed for the weather. Thus he urged consideration of external factors, such as social determinants, that influence an individual’s development of obesity.
In his eighth example, Lee underscored the need for multilevel interventions. Addressing the obesity epidemic effectively, he argued, will require multiple multiscale, layered, and integrated policies and interventions that address the multifaceted contributors to obesity.
Ninth, Lee urged the use of new, innovative technologies to understand and address complex systems, asking, what if society were to rely on old technologies to understand weather patterns? Clearly, he said, using old technologies to predict the weather would not provide the most accurate understanding of what weather to expect. Lee urged the use of advanced technologies, such as agent-based modeling and machine learning, to address the obesity problem, as they can provide new insights about obesity prevention and control.
Lastly, Lee asserted that the overly complex nature of problems should not prevent society from making concerted efforts to address them. Despite the fact that meteorology is complex and difficult to understand completely, he stressed the importance of not allowing these challenges to discourage people from trying. “We have to start somewhere,” he argued, “even if initial efforts provide only partial solutions to the problem. The iterative nature of systems modeling will gradually enable better understanding and in turn, better solutions.”
MORTALITY CONSEQUENCES OF THE U.S. OBESOGENIC ENVIRONMENT
Ryan Masters, assistant professor of sociology and faculty associate of the Population Program and the Health and Society Program in the
Institute of Behavioral Science at the University of Colorado Boulder, discussed efforts to estimate the mortality consequences of the U.S. obesity epidemic. This issue can be approached at the population level by examining death rates from cardiometabolic diseases, he explained, which are the greatest contributor to poor trends in U.S. life expectancy. At the individual level, on the other hand, he pointed out that characterizing the relationship between obesity and mortality risk is a major challenge.
Masters began by reviewing recent trends in cause-specific mortality at the population level. He shared data indicating that among several age groups of middle- to older-aged adults, trends of decreases in death rates from cardiometabolic diseases since 1990 have begun to stall. This flattening of death rates began sooner after 1990 among people in their 40s and 50s compared with people in their early 60s, he noted, and was more pronounced in all age groups among women compared with men (Figure 10-1). The latter differential, he explained, is thought to be related to differential exposures to the U.S. obesogenic environment.
According to cohort-based trends in average rates of mortality from cardiometabolic diseases, Masters elaborated, both Black and White men and women born in the early 20th century experienced steady reductions in those rates. These decreases were reversed among those born in the 1950s, 1960s, and 1970s, he relayed, with the increasing mortality from cardiometabolic diseases presumably being driven by prolonged exposure to the rising U.S. obesogenic environment. When U.S. trends in cardiometabolic disease mortality are compared with those in other high-income countries, Masters pointed out, an “alarming” widening of the difference between the United States and its peers can be seen to have occurred since 2008–2009 (Figure 10-2). This gap is suspected to be linked to obesity-related deaths, he noted, but has not been formally analyzed.
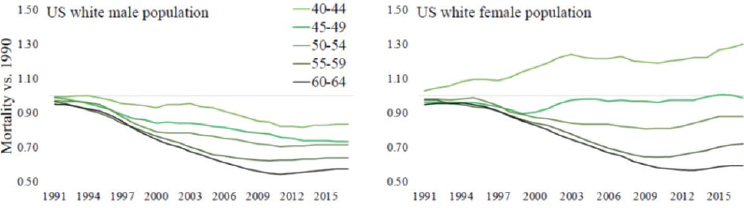
SOURCE: Presented by Ryan Masters, October 28, 2021 (data from NASEM, 2021). Reprinted with permission.
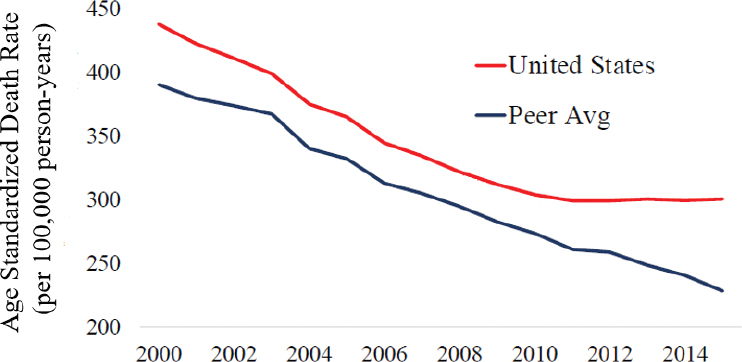
SOURCE: Presented by Ryan Masters, October 28, 2021 (data from NASEM, 2021). Reprinted with permission.
Masters transitioned to discussing work by Acosta and colleagues (2022) that examined drivers of this widening gap between the United States and its peer countries. The primary hypothesis is that the gap is an effect of changes in behavioral risk factors, he explained, including an increase in the prevalence of obesity, a slowdown in smoking reduction, and an indirect effect of the opioid abuse epidemic. At a more granular level, Masters shared additional data from Acosta and colleagues (2022) indicating that prior to the gap’s widening, both the U.S. male and female populations had begun catching up to peer countries (the convergence period) in terms of cardiovascular disease death rates before they started falling behind again (the divergence period). According to Lee, the convergence was due primarily to faster rates of reduction in deaths from ischemic heart disease in the United States versus peer countries, while the divergence was driven by U.S. increases in hypertensive diseases and stroke.
Shifting to discuss the specific causes generating these trends, Masters characterized trends in obesity-related deaths as prominent and highly concerning. The U.S. disadvantage (compared with peer countries) in terms of obesity-related mortality has widened over the past one to two decades. Essentially, Masters observed, obesity-related mortality has driven the increasing gap in cardiometabolic disease–related mortality between the United States and other high-income countries over this period. However, Masters added, it has been difficult to demonstrate empirically that the mortality consequences of having BMIs in the obesity category or other individual-level indicators of obesity translate into elevated mortality risk.
Related to this challenge, Masters referenced two key events in 2013: the first was publication of a systematic review and meta-analysis indicating that mortality rates were similar between populations with obesity and populations with low BMIs, and that individual-level mortality risk was lowest among people with BMIs in the overweight category (Flegal et al., 2013); the second was a call for researchers to identify better metrics than BMI for investigating the individual-level association between obesity and mortality (Ahima and Lazar, 2013). Masters expounded on the latter, relaying the authors’ observation that both individuals with low BMIs and with BMIs in the obesity category exist on the low end of mortality risk as well as the high end. The difference in mortality risk is believed to be an outgrowth of differences in individuals’ metabolic health profiles, he continued, with low mortality risk reflecting such indicators as greater fitness and normal insulin and blood sugar levels, and higher mortality risk reflecting such indicators as chronic illness, sarcopenia, lower fitness, and inflammation. These observations spurred a new era of proposed indices, Masters noted, that use measures of body size, shape, mass, and adiposity distribution to assess metabolic health and mortality risk more accurately.
In Masters’s view, instead of pursuing new indices of body composition, it may be more worthwhile to examine individuals’ life-course exposures to high or low BMIs. Whether an individual is metabolically healthy or not, he elaborated, can sometimes be associated with that person’s cumulative duration of time spent in different BMI states. To illustrate this point, he reported that about 22 percent of respondents to the National Health and Nutrition Examination Survey with BMIs in the normal weight category at the time of the survey had had BMIs in the overweight or obesity category a decade prior. Similarly, many respondents with higher BMIs had been classified as having BMIs in the normal weight category 10 years previously. Masters observed that people who have been stable at BMIs in the normal weight category exhibit more metabolically healthy profiles (i.e., they are less likely to report having hypertension, diabetes, or hyperlipidemia, for example), compared with those who moved from higher BMIs to BMIs in the normal weight category. He added that people who have only recently developed high BMI levels are in better metabolic health than those who have lived with high BMIs for longer periods of time.
Masters explained that, after adjusting for a person’s lifetime history of being in different BMI states, the relationship between BMI and individual-level mortality risk shifts from a U-shape (where risk is elevated at both underweight and very high BMI levels) to a nearly linear association where mortality risk rises as BMI rises (Berrington de Gonzalez et al., 2010; Prospective Studies Collaboration, 2009). This finding raises the question, he proposed, of whether it is best to pursue better metrics or to pursue better research designs and understanding of lifetime exposures to different weight
states and body types. For population health, Masters suggested collecting measures early and often across individual and cohort life courses to observe trajectories and durations of time spent in different body shapes, sizes, and adiposity distributions. Current efforts appear to be shifting away from BMI and toward new metrics, he observed, but he believes it is worth considering the payoff for pursuing new metrics compared with that for pursuing new questions and designs with which to explore associations.
PANEL AND AUDIENCE DISCUSSION
Following their presentations, Lee and Masters answered questions about barriers to implementing obesity solutions; obesity, life expectancy, and mortality risk; and the relationship between health care spending and life expectancy.
Barriers to Implementing Obesity Solutions
A participant commented that evidence-based obesity solutions take a long time to develop (e.g., to satisfy concerns about rigor and replication in different environments) and to implement (e.g., to change complex political and economic systems), and asked Lee what else impedes the implementation of solutions. Lee cited three obstacles that he said reflect broken underlying systems. First was that different disciplines and sectors operate in silos. Lee acknowledged that deep, sector-specific understanding of an issue can be helpful, but stressed that obesity is not a discipline-specific issue and called for more efforts that cut across disciplines and sectors. As a second obstacle, Lee referenced the truism, “perfect is the enemy of the good,” arguing that instead of waiting for optimal conditions, efforts must start somewhere. Third, Lee urged reframing obesity as a systems problem instead of an individual problem, noting that inaccurate framing will not lead to the types of solutions needed to make population-level differences.
Obesity, Life Expectancy, and Mortality Risk
Masters answered a participant’s questions about the relationship between obesity and the recent decrease in U.S. life expectancy, as well as the role of the duration of obesity exposure. He reiterated that trends of increased U.S. life expectancy have flattened since 2010. Compared with peer countries’ trends in life expectancy, he continued, the United States has been diverging away since at least the 1980s. At the population level in the United States, he observed, the link between the rising prevalence of obesity and the causes of death most responsible for the stall in life expectancy is increasingly clear, although it has not been fully demonstrated in a convincing way.
Masters added that the field does not yet have robust measures or data designs to capture lifetime exposure to or indicators of time spent at high BMIs or other individual-level metrics of obesity. He clarified that the study designs he espoused in his presentation would require longitudinal follow-up of large cohorts, which is costly because datasets need to be large enough and to represent a sufficient duration of time to enable observation of individual-level trajectories and long-term outcomes.
Another participant asked Masters whether any long-term studies had followed a cohort from childhood to examine the relationship between duration of exposure to obesity and mortality. Masters replied that the National Longitudinal Study of Adolescent to Adult Health (Add Health) cohort will probably be a solid indicator of the health consequences of an extended duration of time spent in the U.S. obesogenic environment because its members have been exposed to that environment since first enrolling in the study (as adolescents in 1994–1995). He expressed hope that, as the cohort ages, this dataset will yield the data needed to inform how exposure to the U.S. obesogenic environment affects mortality risk.
Relationship between Health Care Spending and Life Expectancy
Crespo asked Lee to comment on why the United States spends more money on health care than peer countries do yet has inferior life expectancy outcomes. Lee suggested that this is an example of a single measure failing to tell the full story, and offered three explanations for the complex relationship between health care spending and life expectancy outcomes. First, health care spending includes administrative and other costs that do not go directly toward patient care, and evidence suggests that administrative costs are rising more rapidly than costs associated with patient care. A second issue is the need to separate costs for treatment from costs for disease prevention and risk factor mitigation, Lee continued, noting that evidence supports the value of investing in the latter. Third, Lee argued that to understand the mismatch between health care spending and life expectancy, one must pay attention to the characteristics of patients on the receiving end of different types of spending. Disparities exist in the demographics of patients who can access health care resources, he observed, and these disparities contribute to different health outcomes among population groups.
This page intentionally left blank.