3
Innovative Methodologies and Technologies
The August 11 session of the workshop opened with an overview of the industry landscape in personalized nutrition, which was followed by reviews of selected innovative methodologies and technologies for personalized nutrition at the genetic, physiologic/microbiome, individual, and social-ecologic scales, with each scale being discussed by three speakers. Bruce Y. Lee, City University of New York Graduate School of Public Health & Health Policy, moderated the speaker presentations and an ensuing panel discussion.
INDUSTRY LANDSCAPE IN PERSONALIZED NUTRITION
Mariëtte Abrahams, Qina, reviewed the landscape of the personalized nutrition industry over the past decade. In 2012, she began, limited scientific evidence was available to indicate the impact of giving personalized nutrition advice beyond that in existing medical nutrition therapy guidelines. The market consisted of a few niche players focused on the “worried well,” she observed, who could afford personalized guidance. She noted that early adopters tended to be highly educated and affluent females, and the solutions dispensed were based primarily on the data obtained from the specimens of individuals; behavior change was an afterthought that was not incorporated into proposed solutions. Abrahams pointed to a subsequent gradual shift in focus from nutrigenetics to today’s more actionable, behavior change–oriented solutions.
Abrahams highlighted a randomized controlled trial (RCT) carried out in 2015 on the use of glycemic response data to develop personalized
nutrition guidance (Zeevi et al., 2015), and a white paper during the same year about the Food4Me study, a web-based RCT on personalized nutrition in seven European countries (Celis-Morales et al., 2017). At the end of that year, she reported, one of the first personalized nutrition companies—which focused on demonstrating effects in terms of behavior change as well as health outcomes—received a large investment, and another company was launched in 2017. By 2018, she said, the personalized nutrition market was gaining steam, and consumer awareness of and interest in its offerings were on the rise.
In 2019, Abrahams launched her own company, Qina, whose aim she described as connecting industry, academia, and health care professionals to ensure an industry focus on science-based solutions with input from practicing providers. She listed a handful of personalized nutrition companies that had been acquired by larger corporations by the end of 2019, and said that in 2020, the COVID-19 pandemic drove consumer interest in and demand for telehealth, smart-eating apps, supplements, and content on how to improve personal health. Another shift occurred at the beginning of 2021, she noted, in terms of the types of solutions offered as well as the business models and partnerships underpinning the offerings.
Abrahams estimated that in 2012, the personalized nutrition market consisted of 16 companies, a number that is now at nearly 400. The industry is highly fragmented, she observed, and difficult to track in terms of both solutions offered and companies’ movement within industry segments through partnerships and collaborations (Figure 3-1). Qina was developed, she explained, to help stakeholders navigate and understand the industry by creating a curated database of personalized nutrition solutions. The company helps industry and patient-facing health care professionals understand which solutions are available, what technologies and data points they leverage, who offers them, and which can be integrated.
According to Abrahams, Qina has observed at least four areas in which the number of companies offering a particular type of solution increased between 2014 and 2021: nutritional supplements; microbiome approaches; dietary preference approaches; and devices, including wearables for tracking biologic parameters.
Abrahams next described key aspects of the current personalized nutrition market. She pointed out that the COVID-19 pandemic has increased consumer interest in nutrition-related solutions based in their dietary preferences and health goals, as well as in additional values around which people are personalizing their diets, such as procuring foods locally, choosing organic varieties, and reducing their carbon footprint. She added that companies are increasingly emphasizing behavior change techniques and adding services to their products, such as connections with registered dietitians, to help people implement personalized advice. She noted that the
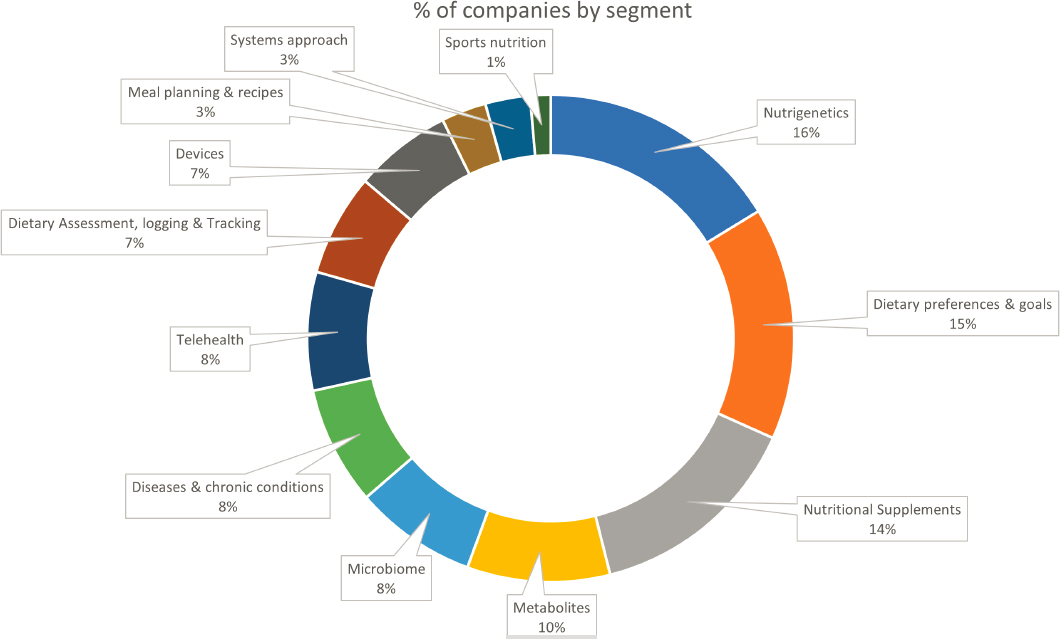
SOURCE: Presented by Mariëtte Abrahams on August 11, 2021.
characteristics of users have also broadened beyond those of the early adopters, explaining that younger consumers are now interested in disease prevention and health optimization, whereas older consumers want to manage and treat symptoms.
As for the corporate structure of the market, Abrahams highlighted an increase in strategic partnerships that she said was driven by the realization that single companies typically are not good at everything, and that teaming could improve the customer experience and provide more value from the data collected. Consumers are demanding more transparency from companies, she observed, including information about the science supporting their products. Companies are no longer focused mainly on funding large clinical trials, she added; they are also interested in data that can inform hyperpersonalization of the delivery and implementation of their services. She cited insights into the optimal interface for consumer interaction and format and frequency for providing information as examples of factors that contribute to consumer success with behavior change.
Abrahams also reviewed key trends in the market, starting with scientific advances driving the development of new solutions. Cardiometabolic health is becoming a driver for new solutions, she observed, and the microbiome is a growing area of focus for many new product launches. She also highlighted the superior outcomes achieved with hybrid approaches that pair personalized nutrition technology with experts who help consumers understand and operationalize the advice they have been given compared with approaches focused mainly on technology. She referenced the earlier perception that the development of sophisticated technology would replace the expertise of health care practitioners. Instead, she argued, it has become clear that such technologies enhance practitioners’ effectiveness in supporting patient goals.
Abrahams turned to another trend toward new entrants into the market, such as the pharmaceutical industry. As an example, she cited a German startup’s digestive symptom tracking app, which pharmacists recommend to patients who purchase products to treat symptoms of irritable bowel syndrome. Additionally, she pointed to the increasing availability of wider applications of some solutions, such as continuous glucose monitors for patients who do not have diabetes or elevated glucose but are interested in tracking their responses to foods. Lastly, she highlighted a demand for the expertise of health care practitioners both in understanding the science and technologies underlying personalized nutrition and in translating the guidance offered into tailored behavior change strategies for individual patients.
Abrahams next shared her thoughts on the future of personalized nutrition. She identified the concept of food as medicine or food for optimal health as a key focus for future developments. The newest solutions in personalized nutrition are closing the gap between the food and health
industries, she observed, with food shopping lists, meal plans, and education being integrated to help patients achieve greater success in following dietary patterns that prevent or treat certain health conditions. Similarly, she described how retailers are playing a larger role in helping consumers improve their health by offering healthy food options and education and in-store guidance to aid them in selecting those foods, wellness services such as biometric screenings, and personalized rewards or discounts. Abrahams cited sustainability as another area of future growth, noting that it is a growing priority for many consumers, fueled by growing interest in food options that are plant-based and produced in environmentally conscience ways. She pointed as well to the emergence of new regulations designed to keep consumers safe as regulators catch up with digital tools. Regulators are giving increasing attention to what types of data are collected by these tools and how they are stored and used, she elaborated, with implications for whether health information being shared on digital platforms is considered medical advice and regulated accordingly.
To conclude her presentation, Abrahams discussed equitable access to personalized nutrition solutions, emphasizing that Qina believes in equity by design. She also suggested that companies should build in levels of product personalization, starting by guiding consumers to credible sources of nutrition education and food literacy or using existing algorithms to provide food-based personalization. Also important, she stressed, is ensuring that the right solutions meet the goals and needs of the relevant population group. She suggested that to this end, companies can use insights into how consumers interact with their products to pair them with solutions they are most likely to use and benefit from. Finally, she highlighted the need for reimbursement for evidence-based solutions for improved health.
Abrahams ended her talk by stating that the personalized nutrition market is dynamic, rapidly growing, and increasingly shifting toward the mainstream, with scientific advances supporting its merits. She added, however, that more could be done to improve inclusivity in research study populations and to provide equitable access to personalized nutrition for a wider consumer base.
THE GENETIC SCALE
Denise Ney, University of Wisconsin–Madison, was the first of three speakers to discuss innovative methodologies and technologies focused on matching nutrition guidance to an individual’s genetic makeup. Ney addressed the topic from the angle of population-wide newborn screening for inborn errors of metabolism using whole-exome sequencing (WES). To introduce the topic, she discussed phenylketonuria (PKU), an inborn error of metabolism caused by more than 800 mutations in the gene that
produces the enzyme phenylalanine hydroxylase. She described PKU as “the poster child for precision nutrition,” given that it exemplifies a condition for which a strong evidence base supports the importance of following a specific diet for a specific genotype. The phenotype of profound cognitive impairment that results from untreated PKU is rare today in the developed world, she observed, because of mandatory newborn screening programs and initiation of a low-phenylalanine diet in affected infants, which was devised in 1953 well before the genetic basis of PKU was understood.
Ney believes newborn screening for inborn errors of metabolism provides an ideal model for evaluating the role of sequencing in population screening. She explained that WES consists of sequencing the protein-coding regions of genes, which are thought to constitute only 1 percent of the genome but to be the location of most known pathogenic mutations. She noted that WES can be compared directly in sensitivity and specificity with tandem mass spectrometry (MS/MS), the established technique for newborn screening programs.
Ney described a recent study on the role of WES in newborn screening for inborn errors of metabolism (Adhikari et al., 2020b), in which the researchers analyzed variants within an exome slice of 78 genes linked with 48 inborn errors of metabolism, ascertained by newborn screening in California using archived dried blood spots from 4.5 million births between 2005 and 2013. Ney emphasized the critical importance of using population-level newborn screening to identify all positive cases of inborn errors of metabolism, many of which are life-threatening, while minimizing false positives to reduce undue stress on families and unnecessary use of medical resources. She explained that MS/MS screening in the study cohort had a sensitivity of 99.0 percent and a specificity of 99.8 percent, which she said is consistent with the quality control standards of the Centers for Disease Control and Prevention for newborn screening programs in the United States. She reported that the sensitivity of WES was 88 percent (571 cases identified, 103 cases missed) overall, but varied among different inborn errors of metabolism. For example, she elaborated, WES detected 100 percent of cases of methylmalonic acidemia caused by known genetic mutations, but only 86 percent of cases of the fatty acid oxidation disorder, very long-chain acyl-CoA dehydrogenase deficiency (VLCADD). According to Ney, the 11 false positives in the study cohort would extrapolate to 8,000 cases per year based on California’s birth rate. In contrast, MS/MS yielded 1,362 false positives in 2015. Ney asserted that these results indicate that WES alone is insufficiently sensitive or specific to be the primary screen for most inborn errors of metabolism (Adhikari et al., 2020a).
Ney described another implication of the study: that WES has advantages as a secondary test for infants with abnormal MS/MS screens before further biochemical and clinical studies are undertaken. Elaborating on
how WES reduced false positives as a second-tier test, she reported that of 108 false-positive VLCADD cases, WES identified 48 as true negatives, and it identified all 16 of 16 maple syrup urine disease cases as true negatives (Adhikari et al., 2020a). According to Ney, conducting WES in a timely, cost-effective manner could have avoided the cost and family stress of follow-up testing for those inborn errors of metabolism. She added that WES also has the capacity to reveal new genetic variants and to suggest the need for whole-genome sequencing, a diagnostic tool used for critically ill patients with unknown diagnoses.
Jim Kaput, Vydiant, was the second speaker to discuss the genetic scale. He described results from a micronutrient intervention study that assessed genetic contributions to phenotype. Results reported in the first article published by the research team indicated that an individual’s genetic ancestry was responsible for at least a portion of baseline levels of vitamin B1 for Europeans, vitamin B12 for Native Americans, and folate for Native Americans (Mathias et al., 2018). Although he characterized these results as important, Kaput said they lack the level of detail needed for application to personalized nutrition guidance.
Because single nucleotide polymorphisms (SNPs) typically do not have effect sizes sufficient for explaining an individual’s response to a nutrient or susceptibility to disease, Kaput and his collaborators next pursued assessment of polygenic risk scores (PRSs) (combinations of SNPs) to determine the genetic contribution to vitamin B12 levels. According to Kaput, formal guidelines for assessing multiple variants do not exist, but most groups rely on genome-wide association studies (GWAS)–identified SNPs or SNPs in specific candidate genes. The limitation of this method, he observed, is that GWAS-identified SNPs are based primarily on populations of European descent. To mitigate this limitation, Kaput’s research team combined results from genotyping arrays and whole-exome data to identify approximately 7,000 SNPs in 90 genes related to vitamin B12, and then determined the genetic ancestry of each SNP using nearby ancestry informative markers from the Human Genome Diversity Project. They then used an analytical approach to identify 36 SNPs within the genetic pathway of vitamin B12 metabolism that could be used for PRS analysis. PRSs of those SNPs explained 42 percent of phenotypes, Kaput reported, with high PRSs correlating with a preponderance of individuals with high baseline vitamin B12 levels (Fuzo et al., 2021). He added that including other factors known to influence health as covariates (age, sex, body mass index [BMI], Healthy Eating Index score, and mean ancestry components) helped achieve the 42 percent result.
Kaput went on to explain that as a proof of concept for use of PRSs in personalized diets, individuals were stratified into tertiles of PRSs that correlated with plasma vitamin B12 levels. He reported that high PRSs were associated with high baseline vitamin B12 levels, and that individuals in this
tercile are therefore at low risk for needing additional vitamin B12, whereas individuals in the middle tercile are at mild risk. Those with the lowest PRSs are at the highest risk for needing additional vitamin B12, a finding that Kaput suggested calls for close monitoring, regular assessment of vitamin B12 levels, and specific dietary recommendations as needed.
In conclusion, Kaput reiterated that assessing multiple genetic variants is more useful than assessing single SNPs for personalizing dietary guidance. It is also important to understand an individual’s genetic architecture, he argued, and to adjust associations by incorporating other factors that may influence metabolite levels (e.g., age, sex, dietary intake) as covariates. He suggested that future efforts to replicate his team’s results should use the same covariates, adding that some of the SNPs they identified may not appear in other studies that draw on other genetic populations. He asserted that similar analyses will gradually lead to the identification of all SNPs in the vitamin B12 metabolic pathway that are valuable to include and can be considered for use on a genetic population–specific basis.
Ahmed El-Sohemy, University of Toronto and Nutrigenomix Inc., was the third speaker to discuss the genetic scale. He began by observing that genetics and gene products such as enzymes, receptors, and transporters can influence various outcomes related to an individual’s response to diet, including sensory perceptions of food, appetite, digestion, absorption, metabolism, and excretion. In El-Sohemy’s view, the evidence base is more robust for genetics than for other omics technologies used to provide personalized dietary guidance, but because the availability and strength of evidence vary by genetic marker, it is important to distinguish where recommendations can and cannot be made.
El-Sohemy stated that his research interest is centered on genes that influence responses to nutrients, not disease-associated genes. As an example of the impact of a single SNP on nutrient response, he referenced a 2-year RCT in which a high-protein diet was found to have a stronger effect on reducing body fat and changing body composition among participants versus those without the AA genotype of the fat mass and obesity-associated (FTO) gene (Zhang et al., 2012). The same effect has been replicated in a number of other studies (Antonio et al., 2019; de Luis et al., 2015), he added, including one conducted in a multiethnic population in Toronto (Merritt et al., 2018).
El-Sohemy next reviewed several controversies in the field by addressing six misconceptions that, he said, are often raised by people skeptical of the value of personalizing nutrition guidance based on genetics (Garcia-Bailo and El-Sohemy, 2021). He responded to the first—that single SNPs are useless—by sharing his belief that this criticism comes from genetic association studies that examine specific phenotypes, such as blood levels of vitamin B12 or 25-hydroxy vitamin D. He pointed out, however, that
predicting baseline blood levels of a nutrient is different from predicting an individual’s response to a dietary intervention based on that nutrient. For example, he said, individuals with low baseline levels of a nutrient might be resistant to increasing dietary intake of that nutrient.
El-Sohemy turned to a second misconception—that people will not change their behaviors—and a third—that more RCT evidence relative to genetics-based dietary guidance is needed. He pointed out that RCT evidence is now available to suggest that giving people certain types of genetic information coupled with actionable recommendations motivates sustainable behavior change (Horne et al., 2020; Nielsen and El-Sohemy, 2014).
El-Sohemy described a fourth misconception—that genetic test results are too complex for people to understand. He agreed that this is likely true if only raw data are provided, but argued that well-designed reports from some testing companies and used by a health care practitioner can help provide practical strategies for needed behavior changes.
El-Sohemy identified as a fifth misconception that family history is more informative than an individual’s genetic makeup. He contended that family history alone is only a crude predictor. Families also share common environments, he pointed out, and without an individual’s specific genetic information, it is not possible to know which offspring have inherited which combinations of particular genotypes that might affect response to a given dietary intervention.
As a final misconception, El-Sohemy identified the belief that people can simply follow recommendations for healthy eating. He pointed out that some aspects of such guidance could be harmful for some people. To illustrate this point, he explained that approximately 50 percent of the population are “slow” metabolizers of caffeine, and for them following the population-based recommendations for coffee consumption will increase their risk of heart attack, hypertension, and prediabetes.
THE PHYSIOLOGICAL/MICROBIOME SCALE
Sarah Berry, King’s College London, was the first of three speakers to discuss innovative methodologies and technologies that focus on matching dietary guidance with an individual at the physiological/microbiome scale. She described the use of big data and novel technologies by PREDICT (Personalized Responses to Dietary Composition Trial) to advance nutrition research and the development of personalized nutrition programs. She explained that PREDICT is an ongoing research collaboration between traditional nutrition academics at King’s College London, Massachusetts General Hospital, Stanford University, Harvard Medical School, and Tufts University and a startup technology company, ZOE, that specializes in machine learning and artificial intelligence (AI).
According to Berry, the PREDICT studies aimed to move beyond the constraints of traditional nutrition research in which trade-offs are made between quantity and precision (e.g., high-precision but low-quantity RCTs, or low-precision but large-scale epidemiological studies). She added that PREDICT investigators also seek to capitalize on big data opportunities from new technologies such as digital devices, clinical devices, remote clinical testing, and even citizen science, all of which she said provide data at scale, breadth, depth, and precision. She did not have time to describe in detail all of the studies in the PREDICT program, but highlighted that they all use novel technology and remote home testing and measure the many integrated and interrelated, multidirectional pathways that determine individual responses to dietary intake. She elaborated on the types of methodologies using examples from the PREDICT 1 study.
The PREDICT 1 study’s aim, Berry continued, was to use genetic, metabolomic, metagenomic, and meal-context information to predict postprandial responses to food in 1,000 UK participants (main cohort) and 100 U.S. participants (validation cohort). During the baseline clinical visit (study day 1), investigators provided sequential test meals; collected metabolomics, saliva, and urine; conducted genetic testing; took anthropometric measures; monitored blood pressure and heart rate; and administered questionnaires about medical history, lifestyle, and dietary intake. Participants then entered the study’s remote, home-based phase (study days 2–14). During this time, Berry reported, participants consumed standardized meals as well as meals of their own choosing; underwent continuous glucose monitoring and monitoring of sleep and physical activity using digital devices; and provided stool samples for microbiome profiling and dried blood spots for measurement of C-peptide, insulin, and triglycerides. They also logged all foods and beverages consumed along with their corresponding satiety levels in an app that was developed by the research team and monitored in real time by a team of nutrition experts who communicated with participants to ensure the provision of complete, high-quality dietary intake data.
Berry illustrated the scale of the PREDICT 1 study data with a series of statistical results, such as 132,000 meals logged, 750,000 metabolomic measures, more than 2 million continuous glucose monitor readings, and 75 billion metagenomic reads. She noted that the amount of data has increased nearly 10-fold now that the PREDICT 2 and 3 studies are complete and recruiting, respectively, and that the PREDICT studies’ dataset now includes 40,000 different meal configurations, enabling investigators to consider such factors as the food matrix and nutrient/nutrient interactions. According to Berry, this volume of data enhances study investigators’ ability to unravel different exposures related to interindividual variability in outcomes, as indicated by wide variation observed in healthy participants’ responses in triglyceride and glucose levels after consumption of a
standardized meal in a tightly controlled setting, for example (Berry et al., 2020). She listed exposures of interest, including meal composition, meal context (time of day, meal sequence), genetics, microbiome, age and sex, blood pressure, and habitual diet, as well as outcomes of interest, including metabolomics, microbiome composition, glycemic control, inflammation, endothelial dysfunction, and hunger and energy intake. She cited serum measures and anthropometry as two examples of factors that are both exposures and outcomes.
Berry next shared a broad overview of PREDICT 1’s results, emphasizing that meal composition and context are as important as such factors as age and genetics in determining an individual’s response to food intake. For each outcome, the investigators explored the relative contribution of different exposures and found that the importance of a given exposure was different depending on the outcome (Figure 3-2). Berry explained that PREDICT 1 also identified microbiome signatures (i.e., compositions of microbes) that were associated either with healthy dietary habits and favorable health markers or with unhealthy diets and unfavorable health markers.
Berry then described how ZOE used the PREDICT 1 results to create a machine learning model that uses an individual’s results from an at-home test to predict his or her responses to food, and then delivers personalized dietary guidance. The at-home test includes a microbiome assessment, which allows for additional personalized guidance to consume either a gut booster or suppressor. As for the future of the ZOE PREDICT program, Berry stated that the research team is “sitting on a goldmine of data” and has only just scratched the surface of its potential to yield new insights and lead to additional, more stratified, and personalized nutrition solutions.
Michal Rein, Weizmann Institute of Science and University of Haifa (Israel), was the second speaker to discuss the physiologic/microbiome scale. She described an approach to personalized nutrition guidance involving prediction of glycemic responses using clinical and microbiome features. Recounting a study conducted 9 years earlier, Rein explained that researchers continuously tracked glucose levels and multiple other clinical, lifestyle, diet, and microbiome measures among 1,000 healthy individuals. They measured 2-hour postprandial glucose responses (PPGRs) (changes in blood glucose levels during the 2 hours following a meal), a metric that Rein said is associated with risk for such outcomes as metabolic diseases, diabetes, and weight gain.
The study produced data on approximately 2 million PPGRs for 50,000 meals consumed by participants, Rein reported, and revealed high interindividual variation in PPGR to the same meal. Responses were reproducible within participants, she elaborated, indicating that they are highly personalized and can be predicted accurately. She described how the researchers used these results along with participants’ clinical and microbiome data to
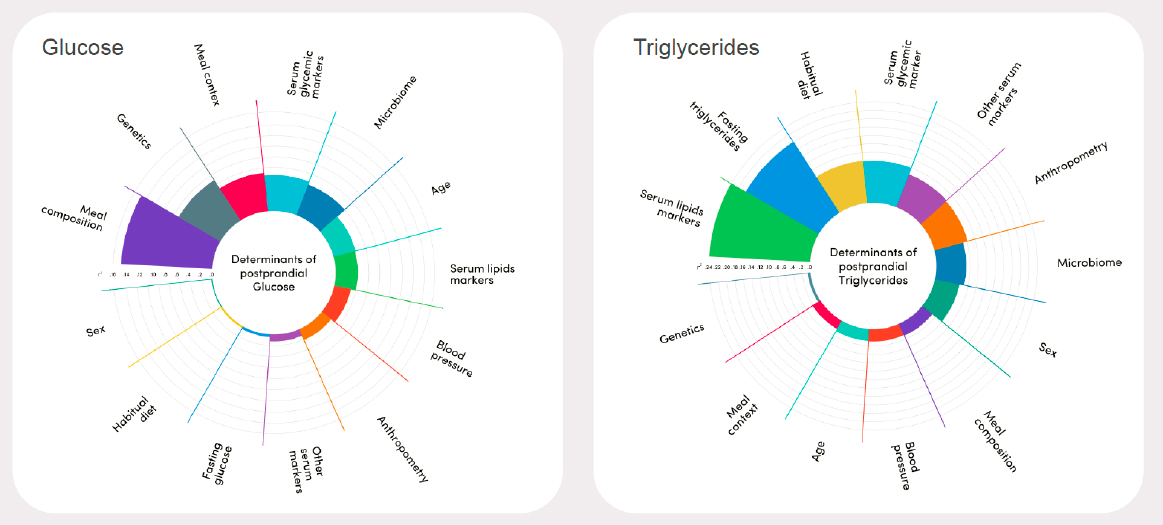
SOURCE: Presented by Sarah Berry on August 11, 2021.
develop a machine learning algorithm that accurately predicted personalized PPGRs to any food combination. A short-term intervention tested the effects of personalized dietary intervention based on the algorithm, she added, and indicated that the personalized diets lowered PPGR in people with prediabetes (Zeevi et al., 2015).
Rein discussed the next phase of this work, which was to assess the clinical impact of a personalized postprandial targeting (PPT) diet. She shared three interventions that tested a PPT diet, beginning with a study conducted in participants with impaired glucose tolerance (i.e., prediabetes). The 225 participants were randomly assigned to a PPT diet or a Mediterranean diet, the commonly recommended diet for prediabetes, and instructed to follow the diet (without restrictions on calories consumed) for 6 months and to undergo additional follow-up for another 6 months. Continuous glucose monitoring and recording of dietary intake continued throughout the 6-month intervention. Rein reported that the PPT diet decreased daily time with high glucose levels and reduced hemoglobin A1c levels compared with the Mediterranean diet, and the researchers concluded that the PPT diet improves glycemic control and metabolic parameters in individuals with prediabetes (Ben-Yacov et al., 2021).
Next, Rein continued, the researchers conducted a pilot study in which 16 participants with newly diagnosed type 2 diabetes who were not taking any glucose-lowering medications were instructed to follow a PPT diet for 6 months. They observed changes in gut microbiome composition associated with improved fasting blood glucose levels, as well as a reduction in hemoglobin A1c levels. Finally, Rein showed that the PPT diet induced diabetes remission in more than 60 percent of participants.
Rein then presented a third, ongoing intervention performed by the company DayTwo, which is testing an algorithm-based dietary intervention in a multicenter dietary modification project among 251 participants with type 2 diabetes taking glucose-lowering medications. She reported that following the diet decreased hemoglobin A1c in more than 80 percent of the participants, and also reduced body weight an average of 4 percent. Thirty percent of participants also self-reported reduced use of glucose-lowering medication after 3–4 months of intervention.
The results of these three interventions, Rein said in summary, support the use of personalized dietary interventions for improving glycemic control and metabolic health.
Guru Banavar, Viome, was the panel’s third speaker. Building on the prior speakers’ discussion of genetics as the basis for understanding glycemic response and other metabolic effects on biology, he stated that gene expression (i.e., the RNA molecules that are produced and transcribed by one’s genome and ultimately control the development of chronic disease) can also be modulated with diet and lifestyle. To illustrate this concept, he
explained that C. diff colitis, an inflammation of the colon caused by Clostridioides bacteria, does not necessarily develop simply when those bacteria are present; rather, the infection begins when the host organism starts to express certain genes that result in illness.
Banavar cited two key technologies that Viome uses to create personalized food and supplement recommendations based on the premise of dietary modulation of gene expression. He described the first, metatranscriptomics, as measurement and detection of RNA molecules across a range of organisms, from microbes to humans. Viome has a scalable platform for extracting RNA data from a user’s stool sample, he said, which are then fed into the second key technology, AI and machine learning, to generate personalized recommendations.
Banavar contended that, based on Viome’s research, genetic expression as measured by RNA molecules is superior to genetics for making dietary recommendations. He has used these data to predict differences in individuals’ glycemic response to foods such that single foods can be classified as “enjoy” or “minimize” for a given individual. Furthermore, he continued, Viome has layered gut microbiome pathway analysis onto the glycemic response data to further classify specific foods as “super,” “enjoy,” “minimize,” or “avoid” for a given individual. Banavar explained that the gut microbiome is responsible for producing metabolites (e.g., butyrate) that influence an individual’s response to foods and for eliminating compounds (e.g., oxalate) that are not beneficial.
Banavar then illustrated Viome’s use of glycemic response data plus microbiome pathway analysis to develop personalized dietary guidance, taking spinach as an example. Although spinach likely evokes a low glycemic response and is typically recommended as a healthy food for everyone, he observed, it is also high in oxalates. He explained that for individuals with high microbiome pathway activity for oxalate elimination, spinach could be a “super” food, but that it could be less beneficial and even raise risk for negative health outcomes (e.g., kidney stones) among individuals with low microbiome pathway activity for oxalate elimination.
Banavar next highlighted Viome’s efforts to feed phenotypic and gene expression data from its hundreds of thousands of customers into its AI engine not only to generate insights for personalized nutrition recommendations but also to detect certain key initial signals of chronic disease. The company has launched a discovery program around this concept, he explained, and is considering a variety of therapeutic areas, including metabolic, gastrointestinal, and oncologic. As an example of the power of RNA-based discovery using large-scale data, Banavar noted that Viome recently received a U.S. Food and Drug Administration (FDA) breakthrough device designation for a biomarker that is useful for diagnosing early-stage oral and throat cancer.
Looking forward, Banavar envisioned the potential to use RNA detection combined with AI analysis to examine host/microbiome interactions. This systems biology approach has the potential to detect specific molecular signatures associated with defined pathogenic processes, he explained, which can lead to predictive, diagnostic, prognostic, and therapeutic clinical applications.
THE INDIVIDUAL SCALE
Andres Acosta, Mayo Clinic, was the first of three speakers to discuss innovative methodologies and technologies for developing personalized nutrition approaches tailored to an individual in terms of both physiology and behavior. He discussed the use of pathophysiological and behavioral phenotypes for guiding obesity management to enhance weight loss.
Forty percent of U.S. adults have obesity, Acosta said, and obesity is a risk factor for numerous chronic diseases, as well as premature death. He reported that, despite U.S. spending of $480 billion in direct annual health care costs on obesity (Waters and Graf, 2018) and the availability of five FDA-approved medications, five devices, three bariatric surgery options, and countless lifestyle programs for weight management, the prevalence of adult obesity continues to rise. Furthermore, he contended, these “one-size-fits-all” obesity treatment approaches are not working, and he proposed that a “precision obesity” approach (to include a precision nutrition component) is essential.
Acosta then elaborated on the shortcomings of existing obesity treatment approaches, pointing to the considerable heterogeneity among patients in how much weight they lose in response to a given treatment, whether lifestyle-, drug-, device-, or surgery-based (Figure 3-3).
Similar heterogeneity exists among patients in traits related to energy balance, he reported, highlighting as an example the wide variation in the number of calories consumed by individual patients before they reached maximal fullness. He added that patients show similar variability in postprandial fullness (i.e., how full a patient feels 2 hours after a meal) and resting energy expenditure (Acosta et al., 2015). Current obesity classifications are based on a patient’s risk of obesity-associated comorbidities (Lewis et al., 2009; Lonardo et al., 2020), Acosta pointed out, instead of stratifying patients with obesity based on prediction of their response to various treatments.
The way obesity is traditionally viewed, Acosta continued, is that phenotypes drive the disease, for which drugs are then developed, and outcomes are highly variable among patients. In contrast, he said, a precision medicine approach starts with the disease and then segments it into various phenotypes with their own unique developmental pathways, an approach
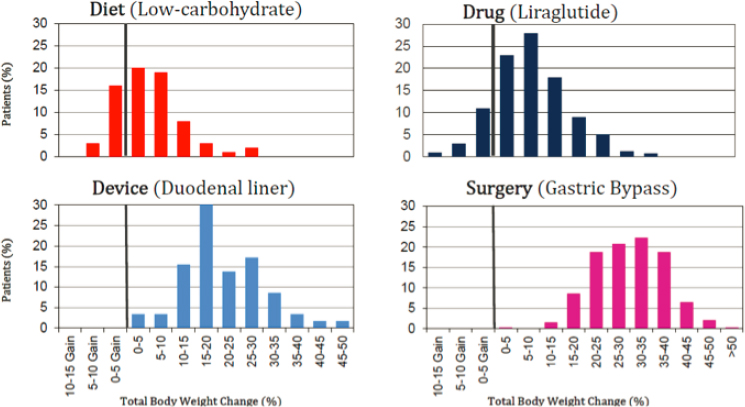
SOURCES: Presented by Andres Acosta on August 11, 2021; Kaplan, 2017. Reprinted with permission from Lee M. Kaplan.
that leads to targeted treatments that result in better patient outcomes. He noted that an individual’s phenotype is determined by the interaction of genomics, which indicates diseases to which the person may be predisposed, with such environmental and lifestyle factors as diet, physical activity, and other exposures.
Acosta next explained that obesity phenotypes have been proposed on the basis of their pathophysiology. For context, he explained that obesity results from an imbalance between an individual’s energy intake and energy expenditure. Intake is driven mainly by hunger, satiation, satiety, and emotional eating, he elaborated, whereas expenditure is driven by resting energy expenditure, exercise, and nonexercise activity thermogenesis. The Mayo Clinic phenotypes patients with obesity based on their results on a series of tests measuring domains that influence energy intake and expenditure. Specifically, Acosta explained, patients arrive for testing after an overnight fast and undergo measurement of body composition and resting energy expenditure. Next, they consume a standardized meal with a radiolabeled isotope that enables assessment of their gastric emptying, and they are also assessed in terms of appetite, hunger, satiation, satisfaction, and emotional eating. Four hours later, patients are presented with an ad libitum meal and instructed to consume as much as they wish. The number of calories they consume before feeling full is measured, and they are followed for another
2 hours to assess satisfaction and fullness. In addition, Acosta said, patients record the number of steps they take and their exercise levels (Acosta et al., 2021).
After conducting this series of tests with more than 500 patients, Acosta explained, the Mayo Clinic applied machine learning to the resulting data to identify four obesity phenotypes (Acosta et al., 2015). The first, hungry brain, occurs when a patient consumes excessive amounts of calories on a given eating occasion. The problem is abnormal satiation, which Acosta described as a problem in the brain. The second phenotype is hungry gut, where the problem is abnormal postprandial satiety. Acosta noted that these patients feel full after consuming a reasonable number of calories, but feel hungry again relatively soon thereafter. The third type is emotional hunger, in which people eat in response to positive and/or negative emotions, and the fourth type is slow burn, characterized by an abnormal metabolism. When distribution of the four obesity phenotypes was measured in 450 new patients (Figure 3-4), Acosta reported, around one-quarter of patients had more than one phenotype, and about 15 percent had none of the four.
Acosta stated that, since identifying the four main obesity phenotypes in 2015, the Mayo Clinic has completed multiple proof-of-concept, placebo-controlled trials to demonstrate that phenotypes can help enhance weight loss in patients with obesity. The trials have evaluated response to
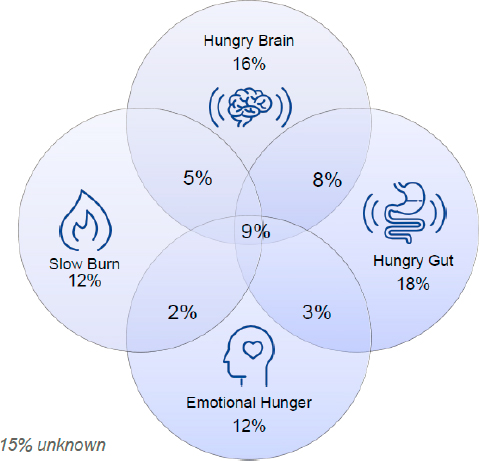
SOURCES: Presented by Andres Acosta on August 11, 2021; modified from Acosta, et al., 2021. Reprinted with permission from John Wiley and Sons.
obesity pharmacotherapy and endoscopic devices, he explained, adding that phenotype-guided pharmacotherapy in clinical practice can almost double the amount of weight loss at 12 months from baseline compared with standard use of pharmacotherapy (Acosta et al., 2021).
Going forward, Acosta said, the researchers will continue to examine the deeper phenotypes underlying an individual’s primary obesity phenotype, using multiomics in an effort to predict phenotypes and identify their underlying causes. He predicted that additional research will also enhance understanding of whether the four phenotypes are causes or consequences of obesity, and indicate whether additional obesity phenotypes may exist or even whether fewer than four phenotypes are necessary for finding the right intervention for the right patient. He predicted further that in the future, it will be possible to measure phenotypes with a simple blood test, and that this will become a routine companion diagnosis helping to improve treatment outcomes for people with obesity.
Samantha Kleinberg, Stevens Institute of Technology, discussed technology-based monitoring of individual dietary intake. She began by stating that current approaches to tracking dietary intake require active input, in contrast to passive approaches that are common for measuring such biometrics as sleep and physical activity. Even with digital approaches to tracking dietary intake, she pointed out, individuals still must remember to take photos and/or complete questionnaires about meals consumed, for example, and are often limited by the challenges associated with complete and accurate recall of food intake. Furthermore, she observed, existing approaches to tracking dietary intake are not typically structured to provide individuals with real-time feedback, which would be particularly useful for those who use diet as a primary tool for managing disease.
Kleinberg described her team’s work on developing passive methods for tracking individual dietary intake, clarifying that perfect accuracy is neither attainable nor the end goal. Even active approaches to tracking dietary intake are associated with error, she said, arguing that passive approaches that were approximately as good as active, human-driven approaches would represent a valuable contribution. She added that efforts to develop passive tracking methods have focused on monitoring when meals occur and what is consumed.
In terms of tracking meal timing, Kleinberg reported that audio sensors (e.g., earbuds that track chewing sounds), motion sensors (e.g., other wearables that measure head motion and arm- and wrist-to-mouth motions), and multimodal sensors that combine audio and motion have been tested. She explained that multimodal sensors can help overcome the limitations of sensors that pick up only sounds or motions, but she acknowledged that even multimodal tools for tracking meal timing provide insufficient information for tracking specific intake, let alone developing personalized guidance.
With respect to efforts to track the specific foods people eat, Kleinberg pointed out that until recently most of this work has occurred in laboratory settings using individual sensors to measure audio or motion or to record images of meals consumed. Multimodal sensors provide more complete data relative to single-mode sensors for tracking foods consumed, she affirmed, but said that her lab’s data suggest that combining multiple types of single-mode sensors is not necessary; rather, one type of motion plus one type of audio sensor is sufficient. In lab settings, she continued, each chew and swallow is recorded and labeled, whereas this level of assessment is not possible in free-living environments. But by combining data from lab and free-living environments, she suggested, it may be possible to identify types of foods consumed in an automated way. She reported that in the lab setting, researchers in one study correctly identified each bite of food by type (e.g., steak, potato, or salad) with 83 percent accuracy (Mirtchouk et al., 2019). A similar degree of accuracy was measured in free-living environments, she noted, and incorporating these data also improved the accuracy (to 88 percent) of lab-collected data (Mirtchouk et al., 2019).
Kleinberg observed that major gaps remain despite advances in passive methods for tracking meal timing and type. She explained that these methods do not provide information about why, when, and what people eat; their levels of hunger and fullness; or the social and emotional context in which eating occurs. She also identified this as another major limitation on the generalizability of the information obtained, because although passive methods can reliably classify intake for 40 or 50 different types of foods (e.g., salad, soup, cookies), the same types of food may vary considerably in composition across cultures and countries.
Looking ahead, Kleinberg predicted that more reasonably priced wearables for audio and motion tracking of food intake will become available in the next 5 years. She added that the level of specificity needed for categorizing foods using these tools depends on the intended use of the data. For example, she said, if the goal is to understand food sensitivities without using an elimination diet, specific foods need to be identified, something that could be achieved by combining self-reported dietary intake with data from wearables. On the other hand, if the goal is to track macronutrient intake, less specificity and contrast will be required, and only one type of sensor (audio or motion) may need to be worn.
Diana Thomas, United States Military Academy at West Point, was the panel’s third speaker. Thomas emphasized the richness of data collected via open-ended, free-form survey questions and analyzed with natural language processing software. She noted that nutrition surveys typically ask multiple-choice or Likert-style questions whereby participants select from a continuum of responses, for reasons related mainly
to perceived ease of analysis. According to Thomas, it is not necessarily easier to analyze the latter responses, and current statistical software is equipped to assess and extract a wealth of information from free-form text survey response data.
Thomas illustrated the value of such data with an example from a survey on weight bias. Of 26 survey questions, only one requested a free-form text response: “In your opinion, what does the American public think about people with obesity?” Assessment of sentiment is one of the first approaches statisticians take to analyze free-form text, Thomas explained, with support from statistical software packages that have built-in dictionaries that assign various levels of positive or negative sentiment levels to different words and classify words according to emotions (Thomas et al., 2020). In this example survey, mostly negative sentiments were detected in response to the above question, Thomas reported, and more people responded with words that fell into the category of disgust relative to any other emotion (Figure 3-5).
When the sentiment data were combined with the survey’s Likert-style question asking respondents to classify their self-perceived weight status, sentiment became more negative as the respondent’s self-perceived weight status grew heavier, Thomas reported. She reiterated that this combination of data from Likert-style, multiple-choice questions and free-form text data provided a depth of insight beyond that gained from either type of question alone.
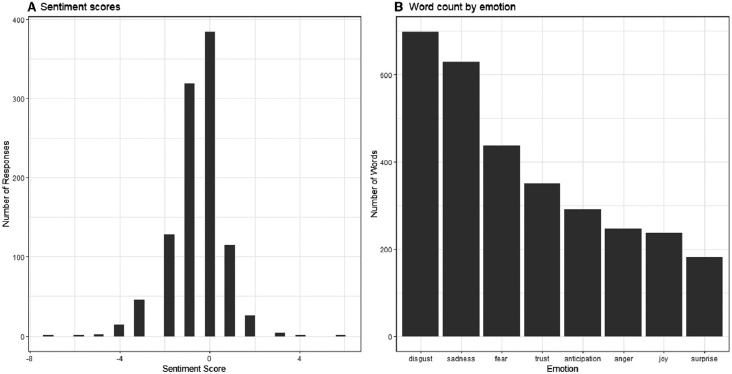
SOURCES: Presented by Diana Thomas on August 11, 2021; Thomas et al., 2020. Reprinted with permission from John Wiley and Sons.
THE SOCIAL-ECOLOGIC SCALE
Kayla de la Haye, University of Southern California, discussed innovations in research on the social and ecological settings in which people access and consume food. Eating behaviors are particularly susceptible to social and environmental influences, de la Haye began, because they are habitual and often shaped unconsciously by proximal cues. She pointed out, for example, that eating companions encode social rules and social resources that can enable or constrain the food choices of those eating with them (Delormier et al., 2009). She maintained that achieving dietary behavior change depends on both personal agency and supportive social and environmental structures. When people exert personal choice and effort to change eating behaviors, she asserted, those new healthful habits will be difficult to sustain if they are embedded in unsupportive structures. She stressed further that people with low incomes and those in communities of color are unequally exposed to structural influences that do not support healthy eating.
de la Haye discussed the use of new sources of social data and network science to understand more precisely how social exposures and social networks influence what people eat. Findings indicate that people are eating in increasingly diverse settings with diverse eating partners, she reported, as they eat food away from home more often, have fewer family meals, and eat with a greater variety of people in their social networks (Koehly and Loscalzo, 2009). Reflecting on her ongoing research, she stated that people tend to eat with about one-quarter of the approximately 20 people closest to them in their social network, although those eating networks vary across people and populations. Longitudinal social network research using network analytic methods has shown that nutritional health and people’s social networks are interdependent, she continued, because people tend to select and form social ties with people who are similar to themselves (Centola, 2011; de la Haye et al., 2011; McPherson et al., 2001; Valente et al., 2009). She added that research has linked these patterns to several contributing factors, including homophily, propinquity, and stigma: homophily is a phenomenon whereby people connect with others who have similar characteristics, such as ethnicity, socioeconomic status, or health risks and inequalities; propinquity is the tendency to connect with others who are geographically close; and stigma drives the exclusion of individuals and groups based on stigmatized traits.
In addition to these social selection processes, de la Haye cited strong evidence that social networks influence people’s health behaviors, including eating behaviors, through such mechanisms of social influence as mimicry and normative influence, as well as by providing social support (Aral and Nicolaides, 2017; Fletcher et al., 2011; Trogdon et al., 2008; Valente et al., 2009; Zhang et al., 2018). She gave the example of a study in which
adolescents with friends who frequently ate energy-dense, low-nutrient junk foods were found to be likely to increase their intake of these foods over the course of one school year. She added that this effect of social network influence was significant after controlling for whom the teens selected as friends, their own beliefs about junk foods, and their demographics (de la Haye et al., 2013).
Overall, de la Haye summarized, these dynamics of social selection, social influence, and corresponding shared exposures influence diet and health such that people with the greatest nutritional health risks are more likely to find themselves in social networks that also confer health risks. She emphasized that these social exposures reinforce and influence nutritional health behaviors, and can be barriers to behavior change or targeted in network interventions.
de la Haye next described two types of intervention approaches that use social networks to promote health behavior change. The first is segmentation, which targets at-risk social groups (e.g., family or peer groups) rather than individuals as promoters of positive social influence, norms, and support for healthy habits (Valente, 2012). A second strategy is alteration, in which an intervention is crafted to change people’s social networks by building new, adjacent health network ties (e.g., through intentional group or buddy activities) that can increase access to positive social influences, norms, and support (Valente, 2012).
Lastly, de la Haye described as another opportunity to improve eating habits the use of new methods and data to better understand the food environments to which people are exposed. Her group is currently using big data from mobility traces (smartphone-captured information about geolocation) to analyze the daily movements of large populations over time, including patterns of exposure to specific food environments and visits to food outlets. In the future, de la Hayes predicted, this type of data will be able to inform tailored interventions that, for example, might intervene in real time to help people make healthy choices in certain food environments to which they are exposed.
Concluding, de la Haye summarized two opportunities to pursue personal and precision nutrition for all populations. She described first the use of innovations in data, data science, and behavioral and social science to gain both more precise insights into the social and built environments where people acquire and eat food and richer knowledge of how social-ecological mechanisms influence diets. As the second opportunity, she identified the application of these findings to develop precision nutrition knowledge and interventions that will be effective, actionable, and equitable.
Sean Duffy, Omada Health, shared examples of his company’s insights illustrating how virtual care can be used to improve health behaviors. As a first example, he explained that when members join Omada, they are asked
what challenges they expect to face as they try to change their health behaviors, such as eating and physical activity. The answers they give, he said, provide helpful context as members begin to develop a relationship with their Omada coach and care team. He added that the verb tenses used in the responses provide additional insight. Responses in the present tense—for example, “I hate to cook” or “I don’t enjoy many vegetables”—are correlated with less weight loss, whereas responses in the past tense—for example, “I used to eat extra portions of sweets”—are correlated with more weight loss. Omada characterized the latter responses as framing a “growth mindset” in which intelligence and talent are viewed as more flexible and changeable.
A second insight, Duffy continued, is that the combination of technology and human support appears to enhance accountability and influence subtle changes in behavior. As an example, he described how when coaches engaged directly with members after noticing that they exhibited unconscious behavioral patterns, the behavior of meal tracking increased to almost the same level as that obtained through automatic nudges, and more weight loss was achieved. Duffy attributed the improvement to the human touchpoint and outreach of care and support.
Based on that insight, Duffy said, Omada developed a strategy for sending members personalized nudges that it calls “coach plays,” in which the program’s coaches identify patterns of member behavior and respond with suggestions for improvements. He described two food-related examples. In one, a coach notices a member tracking the same breakfast several days in a row and suggests ideas for small substitutions that maintain similar flavors but increase the nutritional quality of the meal. In the other, the coach notices that a member has not mentioned a particular type of food in the past month of meal logs and suggests how the member might enjoy that food in future meals. Omada has observed modifications in user behaviors and improved member–coach rapport, Duffy reported, within 2 weeks of these types of coach plays.
Michael Howell, Google, reviewed three categories of publicly available, anonymized, and aggregated datasets that are useful for public health research. The first is aggregated, anonymized search queries, which, he explained can provide insights into people’s information needs and how those needs change over time. As an example, he showed how the Google Trends tool illustrated a sharp increase in interest in COVID-19 between January and March 2020. As the pandemic evolved, Google made available two additional search query datasets: COVID-19 Search Symptoms (geographic trends in searches for specific health symptoms) and COVID-19 Vaccine Search Insights (trends from searches for topics related to COVID-19 vaccines, such as safety and side effects).
As a second category of Google datasets to support public health efforts, Howell described maps that can aid in understanding distance and
how it relates to travel time, which he explained can provide information about built environments. As an example, he shared a research article comparing global differences in travel time to health care facilities by car and by walking, which included a color-coded map to quickly convey differences among regions (Weiss et al., 2020). Another example of the use of distance and travel time data is the COVID-19 Vaccination Access dataset, which Howell noted has been used by Boston Children’s Hospital and Ariadne Labs to identify “vaccine deserts” and to calculate travel time to vaccine locations from various starting points.1
The third category of anonymized, aggregated datasets described by Howell is community mobility. An example of this dataset’s application is research examining how many people traveled from one zip code to another during a given period of time, which he said was used to develop a hierarchical organization of urban mobility and its connection with city livability (Bassolas et al., 2019). He noted that community mobility data have also been used to assess trends in movement to various destinations during the COVID-19 pandemic. He illustrated this function with a real-time example showing that at the time of the workshop, Santa Clara County (California) was 51 percent below baseline for movement to workplaces but only 10 percent below baseline for movement to grocery and pharmacy locations.
PANEL DISCUSSION
Promoting Health Equity
Responding to a question about how the personalized nutrition industry can avoid targeting a narrow segment of consumers at the cost of equity, Abrahams asserted that companies with values centered on improving the health of all consumers tend to partner with like-minded companies. They also seek inputs from health care professionals, she added, about incorporating equity at the front end of product and service designs.
Replying to a question about how to ensure the collection of adequately diverse data for the development of personalized advice across a broad spectrum of the population, Berry offered two comments. First, she proposed the use of novel approaches to recruiting people for such research, aimed at making participation as accessible as possible for diverse populations. Second, she argued that personalized nutrition is at a transition point at which it is creating large-scale, high-depth data and can apply those data in a stratified way that can be more broadly accessible. With continued
___________________
1 See Vaccine Equity Planner, available at https://vaccineplanner.org (accessed October 11, 2021).
learning, she predicted, it will be possible to identify key dietary factors that are important for an individual based on a few simple attributes.
Banavar noted that Viome has made a breakthrough in reducing the cost of RNA sequencing to less than $100, which he estimated to be affordable for most U.S. consumers. He predicted that in the next few years, further advances could make this technology affordable for middle-class populations in developing countries, and that eventually the costs could decrease even more. He proposed shifting the focus from the cost of the technology to its value in terms of health advantages it can provide.
Reimbursement for Personalized Nutrition Solutions
According to Kaput, it is necessary to apply machine learning to integrate more data across multiple scales (e.g., genetic, microbiome) in order to grow the evidence base to a point that it can support reimbursement for more personalized nutrition solutions. Payers will want to see a return on their investment, El-Sohemy added, and he suggested that it would be helpful to have economic analyses demonstrating that investments in personalized nutrition solutions can be cost-saving in the longer term.
Duffy explained that Omada members do not self-pay; the company approaches payers to request that they cover the Omada solution based on its demonstrated value in clinical and economic studies. He noted that for nearly 10 percent of commercially insured U.S. adults, at least some part of Omada membership is a fully covered benefit, coded as a preventive benefit and bypassing deductible and coinsurance payments. Omada has focused on the commercial insurance market to date, he added, but in the future wants its services to be covered by all types of health insurance policies.
Addressing Potential Biases in Computational Methods Used to Develop Personalized Solutions
Howell commented that although technologies such as machine learning are powerful, they have limitations, such as the inability to fully address the different types of bias associated with their multiscale data inputs. In Banavar’s view, a key approach to addressing bias is increasing a study’s sample size and ensuring that its design incorporates participants with diverse phenotypes. He suggested that sample sizes exceeding 100,000 and capturing phenotypes from 70 or 80 countries are representative across the human population and fundamentally help to reduce bias. Berry and Rein agreed, and Berry added that a challenge is recruiting participants from a broader demographic than the “worried well” population that tends to volunteer for nutrition research studies and/or purchase personalized nutrition products.
Banavar also maintained that collecting real-world evidence is critical for improving the usefulness of computational methods. Initial training of an AI or machine learning system is constrained by the training dataset he observed, but it is important that continuous learning and updates to the model occur as the dataset grows with the addition of real-world evidence. Berry added that as datasets grow, researchers would do well to evolve their processes and algorithms accordingly, and to announce that their advice has also evolved based on the availability of additional information.
Acosta urged testing of processes in unsupervised as well as supervised settings (i.e., clinical trials), so that both critical reasoning and empirical validation can be applied. Thomas pointed out that a limitation of natural language processing is that sentiment dictionaries are human-made and do not necessarily capture all nuances of current trends in the use of certain words and phrases; therefore, researchers must review the data manually and ask whether the results of applying natural language processing make sense in the overall context of a participant’s responses. Machine learning cannot indicate definitively what is going on, she stressed, reiterating the importance of applying a human element to data analysis.
Using Technology to Provide Insight into Social-Ecological Contexts
de la Haye observed that the suite of data sources now available, such as those in Google, provides the opportunity to gain understanding of populations and their exposures across various levels of social factors and built environments. The ability to analyze these kinds of data at the large-population scale is particularly exciting, she argued, as it allows for identification of differences in exposures and disease risk across populations. Such insights may become apparent only after repeated exposures over months or even years, she noted, giving these data sources advantages over smaller, shorter-term studies that would be less likely to pick up those signals.
Involving Social Networks and Communities in Research Designs
de la Haye observed that community-based participatory research approaches are increasingly being used for social network and ecological-focused interventions. These approaches, she elaborated, draw on local community organizations and families as key networks of people who are open to helping with intervention planning and creating social and community-level change. She stated that the availability of stakeholder and participant networks is critical in setting the stage for policy and environmental change interventions to be successful and sustainable.
Duffy described his vision for Omada’s ability to help answer the research community’s questions regarding personalized nutrition, which
includes enrolling a large-enough membership base to serve as the source for recruiting participants for large-scale RCTs. He also stressed that personalized nutrition guidance must be aligned with an individual’s ability and willingness to change behavior, and suggested that technology could provide “digital trails” to facilitate behavior change.
This page intentionally left blank.