“The identification of genetic variants from large-scale human population studies is just the first step in what turns out to be a long and often unfortunately unsuccessful process of linking genes to mechanisms of causality,” said John Ngai, director of the National Institutes of Health Brain Research through Advancing Innovative Technologies Initiative (BRAIN Initiative).1 However, a diverse set of new approaches have begun to break down the bottlenecks encountered throughout this process, including the lack of ancestral diversity, said Ngai. These approaches combine cutting-edge experimental technologies with sophisticated computational and statistical methods, he said.
COMPLEX TRAIT FINE MAPPING ACROSS DIVERSE BIOBANKS
Ngai described one of the challenges in interpreting genetic data as “separating the wheat from the chaff.” Hilary Finucane, associate scientist in medical and population genetics at the Broad Institute of MIT and Harvard and associate member of the genetics program at the Stanley Center for Psychiatric Research, uses statistical genetics approaches to address this problem. The use of genome-wide association study (GWAS) has identified hundreds of genome-wide specific loci associated with diseases such as schizophrenia by scanning the genome one variant at a time and then testing each variant for the disease, said Finucane. The challenge she and her colleagues have tackled is how differences at each one of these loci contribute to disease risk. Are there individual genetic variants driving the association or are multiple genetic variants working together?
Finucane said she thinks a very simple pattern of variants underlies this complex pattern of association. Statistical fine mapping enables recovery of simple causal structures from complex association data, she said. These methods assign each variant a value called a PIP (posterior inclusion probability) that indicates the probability that the variant is causal. Fine mapping also enables identification of a group of variants called a “credible
___________________
1 To learn more about the BRAIN Initiative, see https://braininitiative.nih.gov (accessed December 11, 2021).
set” that harbors a single causal variant. By analyzing fine-mapping results from multiple biobanks, Finucane and colleagues have been able to identify putative causal variants and credible sets that are present across multiple populations as well as the genes on which these variants converge.2
Finucane added that the Psychiatric Genomics Consortium3 has performed similar analyses in the most recent schizophrenia GWAS. In this study, also published in medRxiv, they used statistical fine mapping and genetic association data to pinpoint a single, well-known missense variant as the driver of one of the associations.4 This is one example of a powerful analysis that is starting to separate the wheat from the chaff, said Finucane. She added that fine mapping can also help prioritize genes that have been nominated at a GWAS locus through a variety of methods. She and her colleagues recently used fine-mapped coding variants to benchmark and quantify the precision of these different approaches. Their study, published in medRxiv, showed that while none of the methods performed very well alone, combining different families of methods improved precision.5
RELATING RISK VARIANTS TO SPECIFIC CELL TYPES
For psychiatric disorders such as schizophrenia, their genetic complexity has limited the ability of GWAS to identify a few key causative genes, said Jens Hjerling-Leffler, senior researcher in medical biochemistry and biophysics at the Karolinska Institutet. Rather, GWAS have demonstrated the genetic architecture of these disorders. For example, in data aggregated from multiple GWAS, the Schizophrenia Exome Sequencing Meta-Analysis (SCHEMA) Consortium6 demonstrated in a paper published in medRxiv that 95 percent of patients carry many genetic variants with very low effect sizes, while a minority of patients express rare mutations that increase the risk substantially.7
To sort out complexity in the 95 percent of patients with low-effect size variants, Hjerling-Leffler focuses on individual cell types in the brain using single-cell transcriptomics, which informs both genetics and pathol-
___________________
2 Made available in preprint format. To learn more, see https://www.medrxiv.org/content/10.1101/2021.09.03.21262975v1 (accessed December 8, 2021).
3 To learn more about the Psychiatric Genomics Consortium, see https://www.med.unc.edu/pgc (accessed December 8, 2021).
4 Made available in preprint format. To learn more, see https://www.medrxiv.org/content/10.1101/2020.09.12.20192922v1 (accessed December 8, 2021).
5 Made available in preprint format. To learn more, see https://www.medrxiv.org/content/10.1101/2020.09.08.20190561v1 (accessed December 8, 2021).
6 To learn more about SCHEMA, see https://schema.broadinstitute.org (accessed December 10, 2021).
7 Made available in preprint format. To learn more, see https://www.medrxiv.org/content/10.1101/2020.09.18.20192815v1 (accessed December 8, 2021).
ogy, he said, by revealing precise loci with differential expression, which would have been masked by bulk data. For example, he and his colleagues showed, using single cell RNA sequencing data from both mouse and human, that for each cell type, a specific matrix of genes is expressed and that certain neurons in the brain are more enriched for the putative risk genes for schizophrenia, suggesting that these cell types play biologically important roles in schizophrenia (Skene et al., 2018).
Hjerling-Leffler and colleagues did a similar analysis for other diseases, including Parkinson’s disease (Bryois et al., 2020). As expected, they showed downregulated gene expression from dopaminergic neurons, but surprisingly, he said, they also observed a clear signal in the enteric nervous system of the gut and in brain oligodendrocytes, indicating an unexpected role for these cells in disease pathogenesis.
Integrating GWAS and transcriptomics enables prioritization of genes and network modules that are causal, said Hjerling-Leffler. Moreover, as Geschwind and Konopka demonstrated, this network biology approach enables them to leverage variability rather than trying to overcome it, he said (Geschwind and Konopka, 2009). Hjerling-Leffler compared this approach to how one would analyze a problem in an orchestra. Trying to infer which instrument is played wrong by recording the average volume of the instrument during a concert is akin to taking the average of gene expression rather than what individual cells express, he said. In either scenario, inferring where the problem lies is difficult, he said. “Instead, if you see how the different instruments harmonize with each other, you can then start seeing that some of them actually played out of tune or out of sync, and that way it’s much easier,” said Hjerling-Leffler.
To experimentally validate these findings, Hjerling-Leffler advocated the use of induced pluripotent stem cells (iPSC) systems to look at human-specific aspects, and animal models to study mechanisms. Caution and clear objectives are essential when applying these approaches, he said.
FUNCTIONAL EFFECTS OF CODING VARIATION
Determining how genetic variation affects function has been an expanding problem in clinical genetics, said Lea Starita, assistant professor of genome sciences at University of Washington and co-director of the advanced technology lab at the Brotman Baty Institute for Precision Medicine. The conventional wisdom in the field was that as sequencing became more widely used, “we were going to sequence our way out of this problem by linking more variants to disease,” she said. “But this is actually not what’s happening.” Variants of uncertain significance (VUS) have been rising exponentially, outnumbering variants with more definitive interpretations, as shown in Figure 4-1.
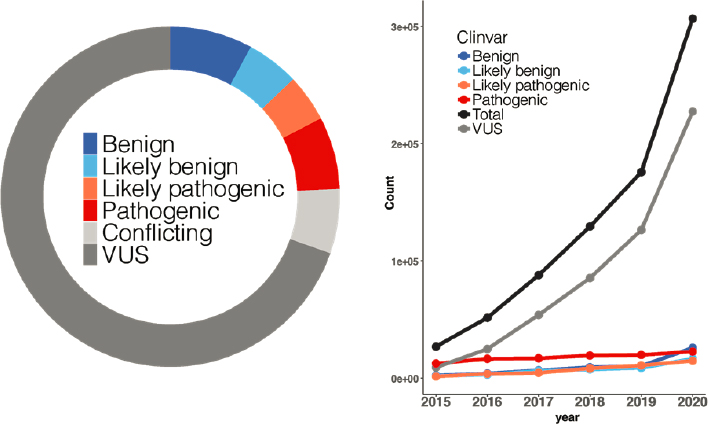
SOURCES: Presented by Lea Starita, October 5, 2021; Fayer et al., 2021.
The increase in VUS has real-world clinical consequences, said Starita. For example, although the detection of pathogenic variants in the BRCA1 gene can lead to a reduction in breast and ovarian cancer risk down to population levels through the use of prophylactic surgery, most variants sequenced so far have uncertain significance, leaving clinicians with little guidance. Using a biochemical or cell-based assay to measure the effects of each variant individually would require “many, many postdoc years,” said Starita. Alternatively, multiplexed assays that tie a functional phenotype (e.g., cell death) to sequencing could enable analysis of thousands of variants simultaneously, she said.
Starita and colleagues are using a technique called saturation genome editing to insert all possible single-nucleotide variants into key regions of the BRCA1 gene. The BRCA1 gene is essential to the human haploid cell line, and to determine which variants were functional or nonfunctional, they sequenced the gene at day 5 and day 11 to see which variants become depleted in the population, said Starita (Findlay et al., 2018). This assay enables them to distinguish pathogenic and benign variants with very high specificity, she said.
In a collaboration with Ambry Genetics, Starita and colleagues are also using this assay to validate the significance of other BRCA1 variants using the American College of Medical Genetics interpretation framework. To scale up this and other technologies being developed to correlate genetic variation with function, the National Human Genome Research Institute recently launched a Consortium for the Impact of Genetic Variants on Function.8 Starita’s lab is also developing more complicated cell models with variants edited into iPSC lines; they and others have launched the Atlas of Variant Effects Alliance to democratize this work and spur others to interrogate more genes and variants across phenotypes using multiplex assays.9
INTERPRETING GENETIC DATA IN NEUROLOGICAL AND PSYCHIATRIC DISORDERS
The challenge of translating GWAS findings into mechanistic insight requires overcoming several additional complexities beyond those already discussed, said Danielle Posthuma, professor and head of the Department of Complex Trait Genetics at VU University Amsterdam and Amsterdam University Medical Center. Among these is the difficulty of pinpointing the actual causal genes within the genetic risk loci identified, she said. Strategies she described to address this challenge include functional annotation, where all variants in a risk locus are annotated with biological information gleaned through several tools; statistical fine mapping described earlier by Finucane; and local genetic correlation analysis where one looks to see whether the genetic variant of interest is also associated with other traits. A second major complexity is the fact that most of the trait studies are polygenic, with small effects sizes at each risk locus, said Posthuma. To overcome this, she said scientists utilized a variety of methods to identify convergence in biological pathways, shared expression of genes, and shared cellular or synaptic functions.
Posthuma’s lab has focused on developing three GWAS interpretational tools—FUMA, MAGMA, and LAVA. FUMA is a web-based platform that facilitates functional mapping and annotation of genetic associations by integrating multiple types of biological information (Watanabe et al., 2017). Analyses are initiated by simply uploading GWAS summary statistics to the platform, said Posthuma. MAGMA provides gene and gene-set
___________________
8 To learn more about the Impact of Genetic Variants on Function Consortium, see https://www.genome.gov/Funded-Programs-Projects/Impact-of-Genomic-Variation-on-Function-Consortium (accessed November 11, 2021).
9 To learn more about the Atlas of Variant Effects Alliance, see https://www.varianteffect.org (accessed November 11, 2021).
analysis and statistical methods that look for convergence (de Leeuw et al., 2015); LAVA analyzes genetic correlations among multiple traits including multivariate and bivariate traits (Werme et al., 2021), she said.
These tools are helping Posthuma and colleagues try to understand why so many loci are implicated in disorders such as depression, what is common among these different loci, and what are the most likely causal variants for each locus. Working in close collaboration with neuroscientists, Posthuma is refining her hypotheses to go beyond just identifying which gene is involved to understanding in what way. For example, which variant is involved with a particular allele to increase risk of disease and the expression of a particular gene in specific cell types across certain stages of development. “The more specific our hypotheses are, the better the functional follow-up experiments can be,” she said.
REGULATORY MAPS, GENE NETWORKS, AND HIGH-THROUGHPUT FUNCTIONAL VALIDATION
As discussed by previous speakers, major challenges facing genetic studies of disease include determining which variants are causal, what genes they regulate, and the functional consequence of those variants, said Daniel Geschwind, professor of neurology, psychiatry, and human genetics and director of the Institute for Precision Health at the University of California, Los Angeles. Nothing trumps functional data, he said, but given that functional studies are resource intensive and expensive, computational methods are essential and complementary.
Much progress has been made in annotating regulatory variants in non-coding regions of the genome that alter the expression and splicing of target genes, said Geschwind, yet even having all that information does not predict functionality fully nor necessarily identify the target gene. As Hjerling-Leffler noted, other functional genetic and biochemical methods done in a cell type, tissue, and developmental stage-specific manner are required to assess functional consequences. Yet there are challenges even to these approaches because, as Geschwind said, “what you look at is what you’re going to find and if you’re not looking broadly, you’re only going to find something narrow that may not be really that relevant.” He added that predictive algorithms also perform poorly and may not correlate with one another very well.
Geschwind and colleagues have been using a massively parallel reporter assay (MPRA) that enables high-throughput experimental testing of the regulatory effects of thousands of variants in parallel. Variants are cloned and transfected into cells, and the effects are assessed by sequencing, he said. In one prepublication study from the Geschwind lab, assaying of more than 5,000 Alzheimer’s disease and progressive supranuclear palsy
(PSP) GWAS variants at 20 dementia-risk loci genome-wide found 320 functional variants.10
Looking genome-wide rather than locus by locus and using actual functional data to build tissue or cell-typed relevant regulatory maps provides a different and more relevant view of disease mechanisms, said Geschwind. In the case of PSP, for example, they found a non-random disruption of a set of transcription factors expressed primarily in neurons, which are known to form a protein/protein interaction network.
Geschwind has been working with the PsychENCODE consortium11 to apply MPRA and other technologies to build regulatory network maps for other neuropsychiatric disorders such as autism and schizophrenia. He and others have shown that network structure is robust and reproducible; that a gene’s network position is biologically meaningful; and that network structure serves as a basis for biologically meaningful insights. Moreover, he said, gene network analysis provides an organizing framework to define the molecular pathology of psychiatric disorders, serves as a quantitative phenotype for cross-disorder comparisons, enables genetic enrichment analyses, and permits the assessment of reliability and relevance of in vitro models.
He further suggested that these genome-wide tools are facilitating a move toward more effective treatments by reverse engineering disease networks, as illustrated in Figure 4-2.
EMBRACING THE COMPLEXITY OF LINKING GENES TO CAUSALITY
The approaches described by Finucane, Hjerling-Leffler, Starita, Posthuma, and Geschwind all embrace the complexity of linking genes to causality, said Ngai. Moreover, integrating these approaches may offer even more power. For example, Geschwind suggested that integrating Finucane’s statistic fine-mapping computational methods and machine learning with functional data could enable the construction of optimized computational tools.
Indeed, Finucane said that such approaches, what she called functionally informed fine mapping, are in development. “Just showing that a variant does something doesn’t mean it’s the causal variant for the disease, so I think that coming up with orthogonal ways of validating and orthogonal types of gold standards is definitely worth thinking about,” she said. She
___________________
10 Made available in preprint format. To learn more, see http://biorxiv.org/lookup/doi/10.1101/2021.06.14.448395 (accessed December 10, 2021).
11 To learn more about PsychENCODE, see https://www.nimhgenetics.org/resources/psychencode (accessed November 14, 2021).
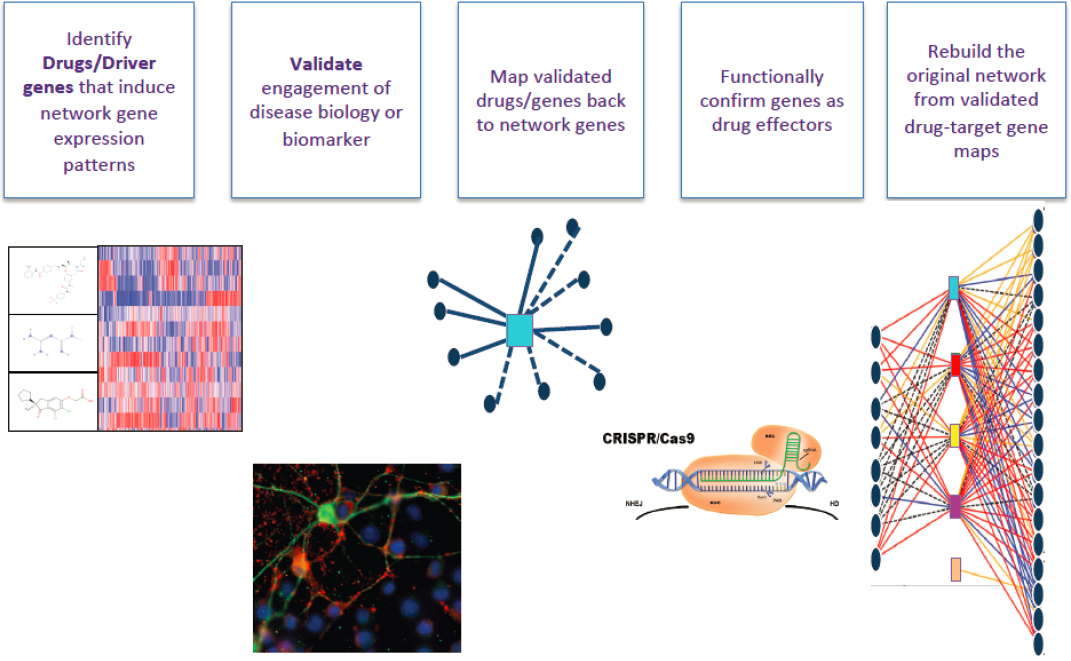
SOURCES: Presented by Daniel Geschwind, October 5, 2021. Co-created by Jessica Rexach in the Geschwind Lab.
added that her lab has also developed a polygenic priority score method for gene prioritization that aims to use polygenic enrichments of pathways and network data, building off work from Posthuma’s lab.
Posthuma noted that having an atlas of genetic variants, as well as GWAS data and disease-specific differential expression data, available in a central database is essential to advance mapping efforts in neurological and neuropsychiatric disorders. Hjerling-Leffler agreed that scale is key, commenting that with the exception of Alzheimer’s there have so far been only small studies with single cell resolution to define and demonstrate the importance of differential gene expression and network biology.
Cell-based assays, including high-throughput single-cell sequencing, have transformed the field’s understanding of the biology of these disorders and enabled the interrogation of disease and genetic variants, said Ngai. Obtaining multiomic data from single samples may also offer deep insight, but adds the challenge of integrating data streams together, he said. Moreover, there is still value in bulk tissue analysis, said Geschwind. “There are things that are missed in single-cell data, especially large genes, membrane-spanning genes, and being able to look at cell–cell interactions,” he said. Hjerling-Leffler added that sequencing of isolated nuclei versus whole cells produces different results. He suggested that certain classes of genes can be under sampled in nuclei preparations such as synaptic genes, which are especially relevant for psychiatric disorders (Skene et al., 2018).
Finucane added that one variant can regulate multiple genes or different genes in different tissues. “So while there are a lot of practical considerations about tissue and cell type, there’s also this kind of scary caveat that if we’ve got the wrong one, we might get the wrong answer,” she said. Ngai agreed, noting that “At the end of the day, it still requires a good understanding of biology to really know under which streetlamp to look more carefully for these effects.”
Moreover, understanding the biology of a disorder can help in identifying the appropriate functional assays to use. For example, cell death is not terribly informative for many genes, said Starita. She said that Douglas Fowler, her colleague at University of Washington, is using high-content imaging and visual cell sorting to identify morphological phenotypes and link them to specific variants. Geschwind added that while in vitro phenotypes such as cell death or axon growth may be relevant for disorders such as dementia, much less is known about the appropriate in vitro phenotype for autism, schizophrenia, depression, and other neuropsychiatric disorders. “Once we know a little bit more about what we’re trying to preserve or enhance, like blocking cell death, I think it will make these in vitro assays much more powerful,” he said.