8
Tools to Enable the Future of Chemical Engineering
Earlier chapters concentrate on specific aspects of the discipline of chemical engineering (and by extension, of its students, academicians, researchers, and practitioners); this chapter is concerned with the tools and capabilities that will be required in the field’s endeavors in the future. Consequently, the content of this chapter cuts across the topics covered in earlier chapters and is motivated by and organized around a defining question: What existing and anticipated tools and capabilities will drive chemical engineering and chemical engineers of the future?
Some of these tools and capabilities currently exist in some form but will evolve in the future, some are emerging as this report is being written, and yet others will need to be created and will coevolve with chemical engineering over the next few decades. Some of these tools will emerge from current and future trends in technologies, with little or no involvement of chemical engineers in their development even though they will have significant impacts on chemical engineering. In other cases, chemical engineers will be involved directly in the development of tools and technologies that will advance science and engineering more broadly. Some of these tools and capabilities will be evolutionary in the sense that their development and application will be gradual and more predictable; others will be revolutionary in that either their development, their application, or both will alter the landscape of chemical engineering research and practice in ways that may be difficult to predict or anticipate today.
Whether engaging in cutting-edge medicine or manufacturing; revolutionizing the design, optimization, and operation of efficient energy systems that respect environmental constraints; discovering and designing new materials; or innovating in any of the other endeavors discussed in earlier chapters, the chemical engineer of today and tomorrow will, conceptually, need to navigate the interface between the natural world and the
data that describe it. What devices (physical or otherwise), tools, or capabilities will make it possible to turn data into useful information, knowledge, and understanding in the future? While the discussion here could address a virtually endless list of tools and capabilities—many of which, when used in combination, will drive innovation—the focus is on four categories: data science and computational tools, modeling and simulation, novel instruments, and sensors.
DATA SCIENCE AND COMPUTATIONAL TOOLS
Chemical engineering has been a data-intensive field from the very beginning. The chemical industry was built on systematic measurement and cataloging of experimental measurements, including thermodynamic properties, phase diagrams, rate constants, flow rates, and heat- and mass-transfer coefficients. Early steam tables from the 1930s exemplify the importance of data-intensive approaches in chemical engineering. These data led to the development of correlations, equations of state, and process simulators, which have allowed industry to design, optimize, and innovate. Until the late 1990s, chemical engineering datasets were stored primarily in print—notebooks, publications, or books. After a glossary of definitions, the first technical chapter of Perry’s classic chemical engineering handbook is a compilation of physical property data (Green and Southard, 2019). The National Institute of Standards and Technology (formerly the National Bureau of Standards) was entrusted by the federal government with the collection of such data, which were subsequently published in the form of much-sought-after compilations and books.
Chemical engineering is undergoing a transformation driven largely by the way data can be acquired, curated, shared, and utilized. Many labor-intensive measurements traditionally performed by humans—from calorimetry, to composition measurements using chromatographs equipped with autosamplers, to more sophisticated systems capable of carrying out and quantifying chemical reactions—are now routinely automated, making it easier than ever to acquire data in the laboratory (Sanderson, 2019). Open-source databases are now commonplace in such areas as biology (Karsch-Mizrachi et al., 2012); thermophysical properties; materials properties (CHiMaD, 2021; Jain et al., 2013; NIMS, 2021; PRISMS Center, 2021); and climate, water management, and agriculture (University of Hertfordshire, 2021). The venerable steam tables, once available only in print, can now be accessed on the internet (NIST, 2021). These transformations are propelling advances on multiple fronts, but they are just the beginning. For example, the chemical industry only recently began the process of digitizing data previously stored over the course of many decades in laboratory notebooks. However, the infrastructure needed to ingest and organize such data most efficiently is not yet available, limiting the potential use of these vast stores of existing data. The way that infrastructure is developed over the next decade will determine how rapidly and how effectively companies and researchers can benefit from gaining access to vast amounts of information from multiple sources.
Moving forward, industry will develop systems that include not only internal, proprietary data but also data mined from the literature and from publicly accessible and commercial databases. Natural language processing tools are improving at a rapid pace,
now capable of extracting sought-after information, such as experimental data needed to validate computer models, from the published literature (Olivetti et al., 2020). How one uses data and integrates data from multiple sources to generate valuable information is expected to become a major differentiator in highly competitive industries. Recent examples that illustrate this point include the optimization of water and energy use in paper mills (Ahmetović et al., 2021); the optimization of raw materials production from natural sources, such as switchgrass (Galán et al., 2021); and the production of renewable fuels from multiple sources (König et al., 2020). If they are to contribute to the development of such tools, chemical engineers will need to develop skills to exploit new possibilities as they emerge (Rzhetsky et al., 2015).
The growing ubiquity of data, along with increasingly powerful computers, networks, and algorithms, is creating opportunities for integration of data from disparate sources on scales never before imagined. It is now possible to monitor every aspect of a chemical plant through a myriad of sensors capable of collecting data continuously, and it will become increasingly common to couple such “lower-level” process data to other types of “higher-level” data pertaining to global supply chains, raw materials availability and characteristics, failure data, responsible water management (Caballero et al., 2020), or climate conditions throughout the world. This capability will enable chemical engineers to optimize enterprise-wide performance at levels not envisaged a decade ago (Hubbs et al., 2020). The COVID-19 pandemic has revealed the fragility of worldwide supply chains, arising from their inherent interconnectedness and the “just-in-time” operating philosophy of many businesses. Chemical engineers will have enormous opportunities to reimagine and redesign these systems to exploit the new ubiquity of and instantaneous access to data to achieve unprecedented robustness. Modern approaches to the design of all processes and products will revolve around the instant availability of relevant data. Growth in the volume of worldwide data is creating both urgency and opportunity for chemical engineers to develop the tools needed to assess data quality and in turn transform all the data into information and knowledge.
Other opportunities for chemical engineers will arise from combining technical and sociological data to achieve advances that could not have been anticipated just a few years ago. Examples include quantifying the dynamics of failure in scientific endeavors or technology translation (Yin et al., 2019) and reorganizing in real-time teams that are optimal for a given collaborative task (Wang et al., 2020). Combining sociological data with, for example, real-time geographic data on pollution, energy distribution, or water availability will allow chemical engineers to integrate sociological implications into engineering considerations to ensure that technology solutions are equitable and inclusive and do not disadvantage any population groups.
Indeed, the possibilities for integrating data and information from disparate sources and domains of inquiry are limitless. A massive amount of data will be available on climate, pollution, water transport, and the environment; on global and local supplies of raw materials; on how processes operate or perform when materials are altered; on such outcomes as quality and consumer demand for the corresponding products; and on human health (e.g., heart rate, blood pressure, oxygen level), both aggregated and individualized.
What new solutions, technologies, and industries will be possible through clever integration of disparate data? The following are some concrete examples.
Process automation.
Highly automated processes will need to be developed based on vast sensing capabilities that previously did not exist, allowing real-time process management and dynamically optimized performance. This domain will grow rapidly as part of Industry 4.0.1 Given its long history with data-driven approaches, the chemical industry will be quick to benefit from such innovations, while other industries, such as food and biopharmaceuticals, may be slower to benefit because of regulatory issues and relative conservatism in the adoption of new technologies.
Human health.
Chemical engineers will aid in advancing the biomedical sciences to facilitate the practice of personalized medicine by addressing the human body as a system, informed by the massive amounts of data generated by medical devices and fitness trackers. Wearable devices invented by teams of chemical engineers and medical professionals will synthesize a multitude of real-time data streams to enable the instantaneous transmission of health information to physicians and phones. In the future, such information may be used to prevent everything from headaches to heart attacks. With their expertise in microfluidics, nanotechnology, and process control, combined with their deep understanding of biological processes within a systems framework, chemical engineers will drive invention and innovation in this space.
Additive manufacturing.
Additive manufacturing enables on-demand production of materials, metamaterials, and parts. New data science tools will enable ever-more-rapid prototyping and scale-up, real-time assessment of performance in situ, and judicious adjustments based on continuous feedback from finite-element models running in parallel and in real time. New tools may one day make it possible to adapt manufacturing processes autonomously and in real time and to use immediately available raw materials based on local supply chain data.
Materials and molecular design.
The rapid design of molecules or materials for targeted applications will be enabled by the development of widely available “living” databases that are updated automatically with experimental data on newly developed materials, and/or with computational data providing predictions about such materials and incorporating updates as they become available. Similarly, understanding of structure–property relationships ranging from the molecular to the manufacturing scale will enable “inverse design and synthesis,” whereby one specifies the end-use characteristics and performance objectives for materials, and the computational tool determines the molecular components required to achieve them. Such tools will enable precision material design from the nanometer to meter scale.
Quantitative structure–property relationships (QSPR) and quantitative structure–activity relationships (QSAR).
Statistics and probability—the classic tools of data science—also have a long history in chemical engineering, as exemplified by the use
___________________
1 Industry 4.0, or the fourth industrial revolution, denotes the automation of traditional manufacturing and industrial processes.
of statistical methods for QSPR and QSAR—mathematical relationships linking, for example, a particular molecule to its structure or pharmacological activity, respectively (Cherkasov et al., 2014).
Automated/self-driving laboratories.
As discussed further below, a future in which artificial intelligence (AI) is used to power automated laboratories is already taking form. To illustrate this concept, consider a polymer material designed to achieve certain end-use performance objectives. Given the raw materials and laboratory process needed to manufacture this material, a robot produces it by running the predesigned process, subsequently characterizing the polymer, and making any necessary feedback corrections automatically until the desired material performance is achieved. Of course, realizing this scenario in practice will require first developing all the sensors and robotics, along with the chemistries and characterization, necessary to enable high-throughput operation. To carry out syntheses, today’s automated laboratories require extensive human intervention for setup and programming. Methods for automated extraction of chemical synthesis actions from experimental procedures documented in papers and laboratory notebooks have been described that can enable automatic execution of arbitrary reactions using robotic systems (Vaucher et al., 2020). IBM Research has described a system2 in which a dataset of chemical procedures derived from cloud-based literature systems is integrated with cloud-based control of a robotic synthesis machine. In a live demonstration, this system identified a route to synthesis of 3-bromobenzylamine and carried out the process using air-sensitive reagents (Extance, 2020).
The preceding examples represent only a fraction of the ways in which the generation of and access to data will change the way chemical engineers work. Tomorrow’s chemical engineers will need to be capable of using the massive amount of data of all sorts whose ready availability will continue to increase. It is easy to imagine a not-too-distant future characterized by data-on-demand, where data on any subject, at any level of granularity, will be readily and instantly accessible. Such a future suggests profound and exciting opportunities for chemical engineers, who are trained in process integration and systems-level thinking—skills that will be required to synthesize disparate data streams into information and knowledge.
Artificial Intelligence
AI is one of the modern tools of data science that is rapidly transforming all fields of science and engineering, including chemical engineering. Over the next few decades, AI is expected to transform all industry sectors in profound ways; indeed, the ways in which AI is used will differentiate companies with respect to gaining a competitive edge. Whereas prior revolutions in chemical engineering were driven by reductionist models, there is ample evidence that the next revolution surely will be data-driven and powered by AI.
AI is a general term used to describe “machines” (fashioned primarily by models and algorithms) designed to mimic human intelligence. With their unique combination of
___________________
2 See https://rxn.res.ibm.com.
knowledge and skills—in particular in mathematics, statistics, computers, analytical thinking, systems integration, and process control—chemical engineering researchers have been among the first to integrate AI into their toolset, and now into the discipline’s curriculum. Engineering colleges are challenged to keep pace with the increasing demand for courses in AI, and in particular in machine learning (ML), the subfield of AI concerned with the development and use of computer systems to learn adaptively from patterns contained in data, using algorithms and statistical models, without human supervision. At the same time, chemical engineering departments are under pressure to teach data science and ML in the context of their discipline, in part because of changing expectations and needs of employers for those who hold chemical engineering degrees. Each year, an increasing number of chemical engineering graduates take nontraditional jobs as data scientists at such companies as Facebook, Airbnb, Microsoft, Google, Uber, Amazon, and more. In a growing number of instances, these companies are hiring chemical engineers for their data science teams preferentially over computer scientists and even data scientists because they recognize that a large part of “doing” data science lies in asking the right questions using existing AI tools rather than, for example, inventing new AI machines.
While chemical engineering has been data-driven since its inception, what is new to the discipline is the explosive growth in the use of AI—and in particular ML—in making predictions from data. Perhaps the most rapidly growing use of AI by chemical engineers is in the area of deep learning—a subset of ML referring to the use of deep neural networks (DNNs), a class of ML methods whereby multilayered neural networks (NNs) are trained to perform tasks or discover hidden correlations in datasets far too large to be handled any other way. Simply put, NNs use structures motivated by networks of biological neurons to learn, through iteration (training), the relationship between inputs and outputs. Because the outputs are labeled and defined explicitly, the learning is said to be “supervised,” as opposed to “unsupervised learning,” exemplified by clustering, where the output is not predefined explicitly, and the algorithm’s task is to discover groupings within data. In NNs, the network architecture is essential to its success. Examples of NN architectures used in chemical engineering research today include convolutional NNs (CNNs, used, for example, to learn images); generative adversarial networks (GANs); recurrent NNs (RNNs); and long short-term memory networks, where each descriptor is indicative of the unique characteristic of the NN structure in question. DNNs can have millions of parameters (node weights) to train and therefore require massive amounts of data to make useful predictions—a challenge for many research problems. Nevertheless, NNs and DNNs have seen success in a number of application areas within and adjacent to chemical engineering. For example, Shell employed four-layer gated RNNs to predict valve failures a month prior to failure (Gupta, 2018).
While ML concepts have existed for decades, only in recent years have computer chip speeds and architectures become fast enough for ML to become sufficiently practical to realize its promise. In addition to enabling faster computations, advances in computers have made it easier to collect, store, and manage large datasets. Furthermore, significant advances in the theory of statistical learning have led to new ML algorithms. The explosive adoption of ML by nearly every technology sector and research area has been fueled by the development and sharing of powerful, open-source, easily accessible libraries that
simplify many of the most cumbersome tasks. This accessibility and ease of use of libraries is in large part responsible for the rapid adoption of ML by chemical engineers.3 Such libraries encode expertise outside the typical knowledge domain of chemical engineers, providing naturally effective ways of expressing and working with complex computations, and thereby facilitating the implementation of data science and ML pipelines.
The relationship between science and engineering disciplines and AI software development has been mutually beneficial: the aforementioned libraries have seen massive investments and improvements because of the value they have provided to data science as a discipline, while AI’s successes with scientific and engineering problems, among others, are driving continued increases in the use of ML software. Science and engineering generally can expect to continue to reap the benefits of industry investments in AI. A 2020 report from Grand View Research suggests that the AI industry will see a compounded annual growth rate of 40.2 percent between 2021 and 2028 (Grand View Research, 2021).
Today, chemical engineering researchers at universities are already using deep learning alongside experimentation and computer simulation. Examples of the many problems for which chemical engineers are making exciting breakthroughs using ML include mapping equilibrium phase diagrams, predicting system failure well in advance, evaluating metabolic networks, designing new molecules and materials, developing immunotherapies, understanding cellular processes and disease, and controlling nonequilibrium processes (Dobbelaere et al., 2021; Sanchez-Lengelingand Aspuru-Guzik, 2018; Venkatasubramanian, 2019). The swift adoption of AI by chemical engineering researchers is reflected in the steep rise in the number and frequency of American Institute of Chemical Engineers (AIChE) fall meeting presentation titles and abstracts containing AI-related terms. Between 2006 and 2020, the total number of presentations involving work using data science in some form increased from 0 to nearly 400, while the fraction of such presentations at a given meeting increased from 0 percent to nearly 7 percent (Figure 8-1). (In each dataset, an abstract is counted only once if the term of interest appears.) The appearance of no other words or two-word phrases increased as rapidly over this period. For comparison, the two most commonly found technical words in fall meeting AIChE abstracts are “bio” (in ~30 percent of all abstracts) and “nano” (in ~20 percent of all abstracts), frequencies that have not grown substantially since 2006.
AI has many potential future roles in chemical engineering. The following sections describe two areas in which the impact of AI in chemical engineering is expected to be profound: manufacturing and materials discovery and design.
___________________
3 Examples of such libraries written for the Python program language include NumPy (performs numerical calculations), pandas (enables data cleaning and analysis), Scikit-learn (implements ML models), SciPy (provides numerical routines and solvers common in science and engineering), TensorFlow (provides a scalable platform for performing ML calculations), Matplotlib (creates data visualizations), and Keras (simplifies the definition and training of neural networks for deep learning).
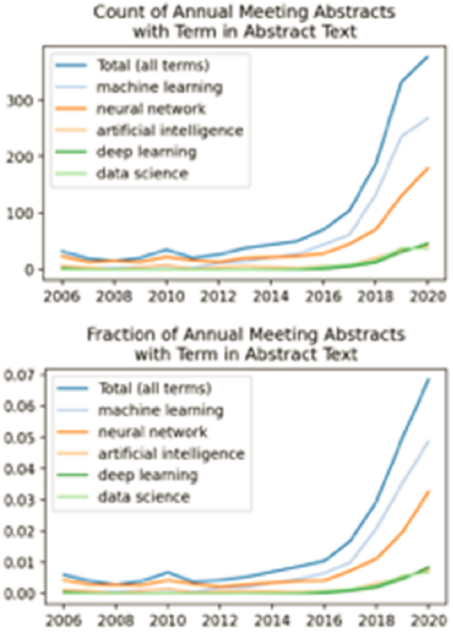
Artificial Intelligence and Data Science Applications in Manufacturing
Enabled by the internet of things (IoT), the availability of and role played by data in large-scale manufacturing have changed dramatically in recent years. It is now reasonable to expect that ubiquitous real-time sensors will be a core part of any future manufacturing operation, and that in general, relevant detailed information will be available at all scales, from the individual plant to the overall supply chain. The result will be a rich set of opportunities not only in the productive use of data but also in the methods for generating high-quality data and for maintaining the security of these processes. In each of these three areas, manufacturing industries are innovating rapidly without waiting for academic research to lead.
Productive Use of Data with Artificial Intelligence
An immediate implication of the above trends is the existence of a “data flood” in many manufacturing settings. Numerous AI tools have been developed to use such data effectively. A major challenge for chemical engineers, however, is that most data science methods originated in fields in which the implications of “off-spec” predictions are less
dramatic than would be the case at a large-scale chemical plant. A related challenge is that many of these methods are not transparent, with components that have no recognizable connection to the physical characteristics of the process in question. Their “black box” predictions are therefore not readily interpretable, a challenge extending beyond manufacturing. These challenges highlight important research and development (R&D) directions for chemical engineers in combining data science methods judiciously with the best aspects of traditional, physics-based models. An underappreciated aspect of data science with particular importance in manufacturing is data quality, curation, and provenance. Important R&D challenges lie in automated assessment of data quality—for example, in the detection of sensor faults in large networks of sensors.
Innovative Modes of Obtaining High-Quality Data
Some aspects of introducing the IoT into manufacturing are as conceptually simple as measuring a process variable, such as temperature, at hundreds of points in a plant instead of at a handful of points. However, there are important areas in which R&D could enable collection of critical data that are currently challenging or impossible to obtain. In a traditional facility, sensors are placed in fixed, predetermined locations. Efforts are already underway in industry to collect data from a variety of locations using mobile sources, such as robots or autonomous vehicles. Chemical engineers have opportunities to collaborate with adjacent disciplines to explore the full implications of these concepts for manufacturing operations. They have opportunities as well in the development of imaging methods that provide low-cost, real-time insight into conditions inside inaccessible spaces (e.g., in reactor vessels) or at nanometer scales, perhaps by leveraging insights from associated areas, such as medical imaging.
Cybersecurity Implications in Manufacturing
Even as the IoT opens up unprecedented avenues for control and management of manufacturing facilities, these same avenues create enormous cybersecurity challenges. Well-publicized incidents of cyber-enabled attacks on physical resources in chemical manufacturing have already taken place. Significant needs for R&D include adapting general principles from the field of cybersecurity to the specific challenges of large-scale manufacturing. Just as data science methods can be used for fault detection, similar methods could be used to detect possible breaches of cybersecurity. It is notable that, as is the case with more familiar aspects of process safety, cybersecurity is a need shared by all parties in the chemical industry, suggesting that public–private consortia focused on precompetitive R&D would be well suited to addressing this challenge.
Artificial Intelligence Applications in Materials Discovery and Design
The nascent fourth paradigm of science has evolved rapidly over the past decade, enabling the use of big data and ML for the design and realization of new, better materials
(Tolle et al., 2011). In the semiconductor industry, for example, ML is enabling the identification of property patterns and anomalies for new materials. Chemical engineers are using ML to predict “sweet spots” for synthesis of new semiconductors, alloys, polymer materials, and nanomaterials through training on previously obtained experimental and simulation data. Force fields—necessary for molecular-dynamics simulations of materials—are well known to fail to predict some material properties (e.g., dynamic properties) accurately while reproducing others (e.g., thermodynamic properties) successfully. Chemical engineers are using ML to generate ab initio and phenomenological force fields that can accurately describe multiple material properties simultaneously, thereby enabling more complex simulation studies of phase transformations in materials, for example. They are designing new catalysts using a combination of electronic structure calculations and ML. Nonequilibrium materials processes (such as self-assembly and crystallization) are challenging to study for many reasons. Combining ML with molecular-dynamics simulations of nanoparticle self-assembly into complex structures, chemical engineering researchers are discovering microscopic details of assembly pathways that can be used to engineer products with fewer defects. In the future, both reaction engineering and assembly engineering will benefit from deep learning and other AI tools.
Scientific inquiry typically begins with a hypothesis generated by a researcher. Using AI successfully for hypothesis generation requires a high-quality training dataset. Depending on the maturity of the field, the exercise may produce a large set of well-characterized existing materials, or the result may be no more than a potential solution space with gaps. Fortunately, these gaps can be partially filled through modeling and simulation, using, for example, ab initio computational methods. Examples of areas in which high-throughput computational screening of materials have been demonstrated include optoelectronic semiconductors (Luo et al., 2020), thermoelectric materials (Sarikurt et al., 2020), and metal organic frameworks (Daglar and Keskin, 2020). In many cases, such AI-based hypothesis generation can be computationally expensive. In the future, the emerging field of quantum computing could greatly reduce the cost of these calculations or enable solutions to problems that would otherwise be intractable using classic computing architectures (Cao et al., 2019). However, technical and programming challenges need to be overcome before quantum computing can become a tool in the chemical engineering toolbox (Almudever et al., 2017).
Once a dataset of relevant materials and their properties (measured or calculated) is assembled as a training set for ML, generative AI models can be used to fill out the design space and suggest optimized synthesis targets, even if they were not part of the original training set. While AI-based discriminative learning (that is, the ability to discriminate among different kinds of data instances) is now commonplace (Ng, 2016), recent improvements in generative modeling (the ability to generate new data instances) are now allowing the generation of hypothetical new materials (Elton et al., 2019; Maziarka et al., 2020). Improved generative algorithms can restrict the output of hypothetical materials to those that are physically possible as manufacturable product (Dan et al., 2020). In the near term, human experts will be required to select potential, viable synthesis candidates from the set of predicted materials.
In the future, the testing phase of the research process also may be enhanced by AI. Standardized machine-readable representations of chemical species (simplified molecular-input line-entry system [SMILES]) and reactions (SMILES arbitrary target specification [SMARTS]) have been developed. These representations have been recognized as analogues to a context-free language (Sidorova and Anisimova, 2014). Atoms and molecules (SMILES) are the letters and words, while reactions (SMARTS) are sentences. Thus, state-of-the-art computer processing for language may be used to improve models for predicting chemical reactions and properties (Whiteside, 2019). Earlier approaches to predicting chemical reactions relied on expert-crafted reaction rules and heuristics to describe potential retrosynthetic disconnections (Socorro et al., 2005), with mixed results. More recent approaches are taking advantage of modern AI algorithms and the large reaction corpora, such as the U.S. Patents and Reaxys databases, to construct purely data-driven prediction engines (Coley et al., 2018). Beside retrosynthetic approaches, AI algorithms for solving the forward problem of predicting products given a set of reactants and reagents have been described (Schwaller et al., 2019).
Computing
From mainframes, to desktops, to cloud computing, to graphics processing units (GPUs), chemical engineers have been among the first to exploit the most powerful computer architectures available. The first uses of computers in chemical engineering can be traced back to the early 1950s (Wilkes, 2002). Since the early 1990s, chip speed has doubled roughly every 18 months. As a result, computing ability—in terms of both time to solution and complexity of problems that can be handled—has reliably increased by roughly three orders of magnitude every decade. What took months to compute in 2000 takes only minutes today and will likely take only a small fraction of a second in 2030. At the same time, the cost of computing has plummeted, democratizing and increasing access to supercomputing resources. The implications of this increase in computing capability and concomitant decrease in cost are profound. It now costs next to nothing to store the output of large simulations for subsequent interrogation offline, or it may now be faster to rerun a simulation when new calculations are desired or to perform real-time calculations as the simulation is running. This flexibility afforded by the broad availability and speed of both computing and data storage means that it is becoming faster and easier to explore vast, multidimensional design spaces; predict phase behavior in complex multicomponent or reactive systems more accurately; or apply ML to large, heterogeneous datasets. It also means that for many chemical engineering problems, simulation and experimental length and time scales coincide. This makes it possible for experiments to inform model development directly and for computation to be a true equal partner with experiment in R&D.
Several relatively recent game changers have emerged in the computing tools available to chemical engineers. An example is the GPU. Driven largely by the multibillion-dollar computer gaming industry, GPUs are now ubiquitous throughout scientific computing and are today a key component of modern supercomputers. Central processing units (CPUs)—the original main processors in personal computers—have a handful of
compute “cores,” exhibit low latency, and excel at serial tasks, but can handle only a few operations at a time. By grouping tens of thousands of moderately fast CPUs or hundreds of powerful CPUs together in a single supercomputer, computations can be carried out in parallel. Since the first parallel computers appeared in the early 1990s, chemical engineers have exploited their capabilities to great effect.
CPU-based parallel computing was the cutting-edge tool in scientific computing until about 2010, when GPUs emerged in personal and business computers. Since the invention of the GPU by NVIDIA in 1999, every desktop or laptop computer has contained a GPU to process graphics displayed on the screen more quickly than was possible with the CPU, which now handles all remaining tasks. In contrast to CPUs, GPUs contain many cores, are high-throughput, excel at parallel processing, and can handle many thousands of operations simultaneously. Game developers and professional graphics designers exploited and drove R&D to increase on-chip parallelism to render ever-more-realistic images in real time. NVIDIA’s public release of the parallel computing language CUDA in 2007 made the power of GPUs accessible to the science and engineering communities, which then could take advantage of GPUs by inserting a few simple commands into their existing codes.
Today, nearly all major scientific software has been or is being ported to or rewritten for GPUs and GPU/CPU hybrid supercomputer architectures, which are expected to be the primary architectures for at least the next decade. In molecular simulation, for example, open-source software such as LAMMPS,4 HOOMD-Blue,5 GROMACS,6 and NAMD (Kass et al., 2011) runs on GPUs, enabling operations up to several orders of magnitude faster than those using CPU-based codes. Together with standards provided, for example, by Dockers,7 computational science and engineering applications can be developed and tested on low-cost laptop and desktop computers; scaled up to run on local clusters, on federally funded computer facilities (e.g., XCEDE),8 or on cloud-based service providers; and then scaled up again to run on the fastest, most powerful supercomputers (including the current fastest open-science supercomputer, Summit,9 at Oak Ridge National Laboratory). The growing use of software “containers”10 greatly facilitates porting simulation code from one architecture to another, making it easier for chemical engineering researchers to use state-of-the-art software without having to know the nuts and bolts of the computer they are using.
Another commercial driver of GPU technology is AI, with GPUs enabling the use of deep learning. The ubiquity of GPUs for deep learning has been accelerated by Tensor Cores, which not only speed up the linear algebra and large matrix operations at the heart of NNs but also carry out, as a single operation, multiple operations common in gaming. By enabling faster graphics and faster, more powerful AI algorithms, the video games
___________________
4 See https://www.lammps.org.
5 See https://hoomd-blue.readthedocs.io/en/latest/#.
6 See http://www.gromacs.org.
7 See www.docker.com.
8 See www.xsede.org.
industry, the financial sector, and nearly every major industrial sector are today indirectly driving breakthroughs in computing for science and engineering. GPUs are also game changers for processing of imaging data, fueling everything from improved computed tomography (CT) scans to self-driving cars.
The continued applicability of Moore’s law—achieved by still-decreasing nanometer-scale chip features, continued densification and thus multiplication of transistors on single chips, continued densification of chips on computer boards, faster networking and switch speeds for faster communications and input/output, new and faster computing algorithms, declining costs, and ever-increasing accessibility—will continue to enable chemical engineers to tackle bigger, more difficult, and more complex problems. Yet while today’s computing power continues to increase, advances in AI are now pushing the limits of what is currently available, motivating the search for alternative options with even greater computing capability (Olson et al., 2016). The holy grail of computing is quantum computing, which until recently has been somewhat of a laboratory experiment but is now becoming more mainstream as an option available through some cloud providers. In traditional computing, a transistor holds the information as a 1 or 0 depending on whether there is a current applied (1) or not (0). In the case of quantum computing, because the qubits are in a superposition of states, they can hold a continuum of values between 0 and 1. Thus, in contrast to traditional computing’s binary system, quantum computers can theoretically hold much more information within each qubit. Given the reliance on managing quantum states to control the computation, the quantum computer’s surrounding environment needs to be tightly controlled against vibration and temperature variation. This is one of the fundamental challenges in quantum computing today, responsible for its high error rates and limiting its adoption. Accordingly, scientists across the globe are working to reduce error rates and foster confidence in quantum computer results. Today’s approaches to this error-reduction problem have been based on the use of several materials, including superconducting materials and ionic fluids, but the expectation is that mainstream implementation may require a material not yet developed. It is widely expected that the requirements for strict environmental controls and high-purity materials for fabricating today’s computer chips will become even more stringent as the computer industry moves toward exascale and quantum computers. These challenges offer additional opportunities for chemical engineers.
MODELING AND SIMULATION
Mathematical modeling—the development and use of mathematical equations as a convenient surrogate for studying, understanding, and even designing a physical entity—has been an indispensable tool for the modern chemical engineer. In general, mathematical models range from simple equations based on material and energy balances of large-scale industrial equipment or empirical correlations connecting one observed phenomenon to another to complex multiscale models that span the entire length scale, capturing atomistic molecular interactions and integrating up to macroscale phenomena.
Traditionally, modeling has almost always been connected to simulation in which a computer is used to solve the set of modeling equations representing a physical system
so the behavior of that system can be explored under various conditions. However, simulation has evolved to be understood in the more general sense of a computer representation of a physical entity with or without the use of an explicit mathematical model. As a consequence, any computational representation of a physical system, whether via explicit mathematical equations or a computer simulation, is understood to be a “model” of the system in question.
Simulation also refers to systems in which interaction potentials between particles are developed to describe many interacting species, and emergent collective behavior is revealed. These approaches are leveraged to understand particles ranging from the quantum to colloidal scale, with detailed or approximate potentials. The results of these simulations often inspire reductionist modeling in terms of mesoscale mathematical models and can be used to understand or predict the parameters in these mesoscale models. Chemical engineers have developed and applied these approaches to understand biomolecular interactions essential to drug design and particle interactions and assembly at the heart of nanotechnology. This cross-talk between simulation to reveal emergent behavior and to inform model development can be further developed to embrace data-driven and equation-free approaches to simulating interactions across scales in complex, far-from-equilibrium systems.
Chemical engineers continue to innovate and drive the field of molecular dynamics (MD) simulation, which was invented in 1957 at Argonne National Laboratory and further developed over the next several decades, primarily in the United States and United Kingdom, by chemists and chemical engineers. Today, the most widely used MD codes—including LAMMPS, HOOMD-Blue, NAMD, and GROMACS, all led by U.S. researchers—are open source. Chemical engineers have developed some of the most popular molecular simulation algorithms, such as free-energy methods (Frenkel and Ladd, 1984), thermodynamic integration (Kofke, 1993), enhanced sampling methods, ensemble simulation techniques (Panagiotopoulos, 1987; Yan and de Pablo, 1999), and acceleration algorithms (e.g., bond-boost; Pal and Fichthorn, 1999). New generalized ensemble simulation techniques, such as digital alchemy (van Anders et al., 2015), use MD and Monte Carlo simulation for inverse design of materials building blocks for targeted structures and properties.
Rapid advances in computer technology and an unprecedented increase in computing power and data storage, combined with the ready availability of user-friendly modeling and simulation software packages powered by extremely efficient computational methodology, have facilitated modern modeling and simulation in chemical engineering. It is now possible, for example, to begin with simple rules for interactions among particles (electrons, protons, atoms) and simulate emergent behavior. On the other hand, such emergent phenomena may be so complex that the computer model itself is an “equation-free” model developed to capture the behavior on the basis of large volumes of experimental observations (using such data analysis tools as ML algorithms; Kevrekidis and Samaey, 2009). It is possible, therefore, that in the future, the distinction between first principles–based and data-based modeling/simulation will become sufficiently blurred as to become almost irrelevant. The following sections describe the role that the dual tools
of modeling and simulation will play in chemical engineering in the future in the specific areas of education, research, and industrial applications.
Education Applications
Since computers were first introduced into chemical engineering, (Katz, 1966), simulation has been an integral part of chemical engineering education; this trend will continue and will likely accelerate in line with the advent of faster and more sophisticated computer hardware and software and transformative approaches (such as equation-free models). The three traditional central components of simulation are (1) the mathematical/computational model or interaction potentials upon which the simulation is based, (2) the algorithms used to carry out the simulation and/or solve the equations, and (3) the computer hardware and software used to carry out the necessary computations and display the results.
The chemical engineering classroom of the future will leverage modeling and simulation to expand student understanding. In the past, student intuition was developed in advanced undergraduate courses using simple canonical examples of chemical processes that permit analytical closed-form solutions. Advances in simulation are already making it possible to complement and expand these pedagogical approaches. For example, molecular-scale phenomena, phase transitions, and fluid flow can all be taught effectively using computer simulations. With virtually limitless computing power, the challenge is to convey this information in consumable form so that students understand the limitations of simple models; develop intuition; and become adept at leveraging this information to design, control, and exploit the systems of interest. Simulation allows students to learn through concrete examples how electronic interactions give rise to interatomic and intermolecular interactions; how these interactions produce structure, properties, and behavior; and how changes at the nanometer scale manifest at the mesoscopic and macroscopic scales.
The future will see improvements not only in the knowledge base upon which to build the models but also in the ability to compile, validate, and deploy such information rapidly and efficiently. Achieving the goal of conveying the information from simulations in “consumable form” will be facilitated by synergistic integration of model development and computation within unified simulation software, coupled with efficient graphical output capabilities and user-friendly application programming interfaces (APIs). Different parts of the chemical engineering curriculum will require different suites of modeling and simulation tools (e.g., MATLAB/SIMULINK for process control, ASPEN for process design, computational fluid dynamics [CFD] tools for fluid dynamics, MD and Monte Carlo simulation for thermodynamics). There is no question as to the increasing role of modeling and simulation tools in the education of chemical engineers of the future. The committee imagines a future in which modeling and simulation tools, in combination with AI and virtual reality technology, will afford students the experience of operating an entire chemical manufacturing plant virtually, in a manner that transcends even that which flight simulators provide to pilots in training today.
Research Applications
Chemical engineers have used simulation to contribute significantly to understanding of systems in which interaction potentials are developed to describe interacting atoms, molecules, polymers, nanoparticles, and larger particles, and emergent collective behavior is revealed. These approaches make it possible to predict the behavior of matter, whether a catalyst for a chemical reaction or a material for a specific application. Electronic structure calculations are used at the smallest level, which can inform classic force fields to access longer length and time scales. Chemical engineers have made leading contributions using such approaches in a wide variety of systems. Examples in biomolecular systems include protein folding and aggregation, hydration, and ligand–receptor interactions; self-assembly of lipids; and the role of hydration in ion complexation. In surfactant systems, examples include prediction of equilibrium mesostructures used to formulate personal care products or to template advanced materials. In nanotechnology, the same simulation methods are used to predict the self-assembly of “colloidal molecules” and patchy particles to form aggregates and assemblies for next-generation soft materials or their directed assembly in the presence of biasing fields.
Mesoscale models, which exploit coarse-grained potentials or even continuum free-energy functionals, are also used to access emergent behaviors (e.g., for protein aggregation in lipid bilayers, or colloidal assembly in complex fluids at length and time scales inaccessible to the most detailed simulations). The challenges for the future include better connecting simulation from one scale to another and developing interoperable simulation tools to inform model development for the systems scale. The coarse-grained or continuum-scale descriptions are valuable in that they include descriptors and parameters that may be related to experimental observations or may be exploited in systems-level mathematical models. Close coupling of these simulations with experiments can form a virtuous circle of simulation, prediction, and identification/determination of mesoscale parameters and experiments to improve understanding of complex systems.
Significant challenges remain in simulation of systems that are far from equilibrium. For example, it is currently challenging to move from the molecular scale, to the mesoscale, to the cellular or tissue level in biomolecular engineering; to relate surfactant mesostructure to rheology in systems under flow; to understand reacting systems with changes in mesostructure and physicochemistry over prolonged time scales; or to understand driven assembly of colloidal suspensions in complex soft matter. As simulation costs decline and data-driven approaches mature, cross-talk between simulation to reveal emergent behavior, mesoscale description, and mathematical models will advance. Such advances will facilitate, for example, environmental modeling, making it possible to start from detailed chemical reactions at the quantum level and integrate up to represent large-scale, macrolevel behavior, which in turn will enable high-fidelity climate prediction over periods of years.
Other rapidly developing tools that will be important for chemical engineering include CFD, computational quantum chemistry, and predictive reaction kinetics. Improved CFD models will contribute to understanding of turbulence. Direct numerical simulation (DNS) and large-eddy simulation (LES) for fluids and methods for two-phase
flows, combined with faster computers, are yielding new insights. Computational quantum chemistry has become invaluable to chemical engineers for modeling force fields, electronic materials, catalysts, thermochemistry, and safety analyses. Chemical engineers are deeply involved in developing methods and codes that leverage these calculations with ML. Finally, tools of predictive reaction kinetics are advancing rapidly. Reactive force fields such as ReaxFF and QMDFF and improvements in ab initio molecular dynamics promise to enable model-based chemical experiments.
Chemical engineers can also meet the challenge of capturing and manipulating the information from simulation and experiment in forms that provide mechanistic insight to inform the design, control, and exploitation of the systems of interest. As research advances on this front, so will pedagogy, as these new approaches and capabilities will be integrated into the classroom.
One other tool that could revolutionize chemical engineering research is the development of interoperable models and software. Currently, there exist software for predicting properties, other software for materials design that use the properties, and yet different software for simulating the material and predicting its performance in end use. Integrating these disparate systems within a framework that facilitates interoperability would greatly speed up materials discovery and design.
With the growing power of AI and its adoption in chemical engineering, chemistry, physics, and materials science, molecular simulation is poised for a revolution. Standard approaches to developing interatomic force fields may become a thing of the past, replaced by the use of ML to develop force fields (Chmiela et al., 2018; Noé et al., 2020). Already there are a growing number of success stories. A recent study (Batzner et al., 2021) shows that with the use of equivariant neural networks, massive datasets are not required for deep learning, and that high accuracy can be achieved with far less computational effort than previously thought.
Industrial Applications
Chemical Manufacturing
Modeling and simulation have jointly played a significant role in the modern chemical and biomanufacturing industries, although applications have focused primarily on large-scale processes and equipment, mainly for operator training and for the design and implementation of advanced control systems. The increasing operational complexities and shrinking economic margins in the petrochemical industry, coupled with stringent environmental and quality demands on the manufacture of specialty chemicals and polymers, have spurred increased use of modeling and simulation. While the pharmaceutical industry currently lags behind the chemical industry, fundamental changes in regulatory requirements are motivating greater use of mathematical models and simulation in that industry, especially in the rapidly growing biomanufacturing sector. With the advent of such Food and Drug Administration initiatives as process analytical technologies and quality by design, an increasing number of leading pharmaceutical companies are incorporating process modeling and simulation systems into their manufacturing enterprises.
One concept penetrating pharmaceutical manufacturing is that of the “digital twin,” described most simply as a virtual representation that serves as the real-time digital counterpart of a physical object or process. Park and colleagues (2021) provide a brief review of potential bioprocess applications.
With increasing capacity for collecting, curating, warehousing, and visualizing massive quantities of process data and with easy access to relevant ancillary data, the next challenge is to develop systems that will integrate process operating data seamlessly with ancillary data from the supply chain, policy, economic trends, global markets, and climate predictions to optimize production planning, scheduling, and operation. This capability will become especially indispensable for enterprises with multiple manufacturing facilities distributed across the globe. Such modeling is also important for scaling up, especially for large-scale plants that represent a large capital investment. The computer hardware technology necessary to facilitate the implementation of such systems currently exists and is advancing rapidly; the necessary software is sure to follow in the coming years.
Systems are currently being developed to allow the industrial practitioner to connect molecular-level models to large-scale process models and insert process models within the context of a facility. Consider as an illustrative example a dynamic model of a CO2 capture unit within a power plant that is capable of predicting the plant’s performance as the electricity output changes dynamically. Imagine that the systems-level model of the power plant is integrated with the market to enable the user to determine optimal plant configurations and product slates in a given market. Such a simulation system will, among other things, allow users to explore options for how best to design and optimize energy production/conversion systems. A beta version of such a system currently exists and offers a glimpse into what is possible in the future (Arent et al., 2021).
To be most useful, however, such systems need to provide different kinds of interfaces for different categories of users, making customized information available in real time as appropriate for each user: technical information for the research engineer and the practicing engineer, and high-level economic and business information and policy implications for decision makers. The committee envisages such systems also possessing the capability to incorporate past experiences (e.g., failures, safety incidents). Chemical engineers already feature prominently in, and in some cases have founded, companies that are commercial vendors of process modeling, simulation, control, and operations software, hardware, and services (e.g., the pioneering process design software ASPEN was developed and the company ASPENTECH founded by Larry Evans, a professor of chemical engineering at the Massachusetts Institute of Technology). Chemical engineers are likely to contribute significantly to the development, implementation, and commercialization of these systems of the future.
Life-Cycle and Technoeconomic Analyses
Life-cycle assessment (LCA) and technoeconomic analysis (TEA) are systems-level analysis tools used by chemical engineering primarily for new chemical technologies; they represent a completely different category of industrial applications of modeling and simulation. As mentioned throughout earlier chapters, the ability to use these tools
will enable chemical engineers to ensure that their innovations have a net lower environmental impact relative to alternatives. LCA is fundamentally about the mass and energy balances of processes and is a standardized methodology for assessing the environmental impact of a product over its entire life cycle (i.e., production, use, and disposal) (Billiet and Trenor, 2020; Muralikrishna and Manickam, 2017; Nuss, 2015; Weisbrod et al., 2016; Zhang et al., 2018b); it can also be used to compare two or more products objectively. LCA has a fixed structure and follows the standards in International Organization for Standardization (ISO) 14040:2006 (ISO, 2006). It can be carried out as a
- cradle-to-grave analysis, which provides the full LCA from the raw material extraction step, through the use step, to the disposal step (grave);
- cradle-to-gate analysis, which provides a partial product LCA from the raw material extraction step to the factory gate (i.e., before the product is transported to the consumer); or
- cradle-to-cradle analysis, in which the end-of-life disposal step is a recycling process.
TEA, a methodology for analyzing the technical and economic performance of a process or product, combines engineering design, process modeling, and economic evaluation. It depends heavily on the technology readiness level (TRL) and includes goal, scope, and scenario definition; cost estimation; market analysis; profitability analysis; and results interpretation. Specifics on TRL titles, descriptions, tangible work results, and workplaces for the chemical industry can be found in Buchner et al. (2018). As the TRL increases, data availability and accuracy increase, and the TEA becomes more reliable.
Integrating LCA with TEA yields what is called environmental technoeconomic assessment (Thomassen et al., 2019), most useful when further combined with energy and mass balances, thermodynamics (Banholzer and Jones, 2013), government policies (e.g., potential carbon tax, packaging recycling content targets, greenhouse gas [GHG] ceilings), social acceptance assessment, and project control structure. This overall integrated assessment structure affects technology directions and needs to be incorporated into the thinking of chemical engineers as they develop new products and processes in the circular economy, discussed in Chapter 6 (see the example discussed in Zimmermann and Schomäcker, 2017). Appropriate modeling, simulation, and data assimilation tools tailored specifically to facilitate such large-scale systems analysis do not currently exist, creating an area of opportunity for chemical engineers as they facilitate the transition from the linear to the circular economy. AI will play an important enabling role in this regard (McKinsey Sustainability, 2019), allowing chemical engineers to harness information contained in large datasets to learn faster and more efficiently from highly complex systems. Specifically, AI can enable chemical engineers to
- design circular products and materials (via ML-assisted design processes for rapid prototyping and testing),
- operate circular business models (via AI’s predictive capabilities from historical datasets), and
- optimize circular infrastructure (via improving the processes for sorting and disassembling products and recycling materials).
Medical Applications
Chemical engineers have contributed significantly to understanding of the molecular systems and mechanisms underlying many of the body’s physiological functions. From signal transduction in cells (how signals are passed from the surrounding environment into the nucleus of a cell) to changes in gene expression in response to these signals, ultimately resulting in the regulation of various biological processes, chemical engineers have developed models that have facilitated the development of systems biology (Kinney et al., 2019). In an earlier generation, Bischoff and his coworkers pioneered the use of compartmental models to study the macroscale distribution of drugs in the human body (Bischoff, 2015). Mathematical models of metabolic pathways combined with novel measurement techniques have facilitated unprecedented high-fidelity analysis of human metabolism (Lachance et al., 2021). At the physiological level, the recent development of the artificial pancreas, an insulin delivery system for those with type 1 diabetes, is based on modeling, simulation, and control mechanisms developed primarily by chemical engineers in conjunction with clinicians (Dassau et al., 2017).
Understanding of human physiology is rapidly approaching a sufficiently quantitative level to permit the development of multiscale simulators of the entire body, from single cells, to tissues, to organs and organ systems, to the entire organism. Such systems can then be used for a limitless number of applications, including clinical trials. These so-called in silico trials will save a significant amount of money and time by allowing prediction of the clinical outcomes of drugs at various stages of development on the one hand, and on the other, facilitating the design and synthesis of complex biological drugs and predicting the behavior in vivo. Such simulation systems can also be used to implement personalized medicine, with the potential to revolutionize the practice of medicine and drug development (Ogunnaike, 2019) (see Chapter 5).
The building blocks necessary to actualize this class of simulators already exist in the form of single-cell models, multiscale biological system information, metabolic analyses, carbon labeling, flux analysis, single-cell genomics, high-throughput screening, and so on. With rapid advances in biological/medical knowledge, data-collection capabilities, and computer hardware and software technologies, the development of such medical simulation systems may occur sooner rather than later.
NOVEL INSTRUMENTS
Chemical engineers have had a transformative impact on instrument development, especially in the establishment of fundamentals underpinning measurement and characterization, in the development of hardware, and in early adoption. Analytical methods and visualization tools are two particular areas that have benefited from the participation of chemical engineers. These methods and tools have had an impact on chemical engineering research and practice in two distinct ways. First, in some cases (e.g., super-
high-resolution microscopy and single-molecule detection), the tools have improved signal-to-noise ratios and improved accuracy and/or precision, thereby making higher-fidelity characterization possible. Similarly, advances in atomic-force microscopy and transmission electron microscopy have provided new information about synthetic as well as biological objects with unprecedented precision and accuracy. Second, improved speed in genomics, proteomics tools, and flow cytometry has allowed more rapid and more comprehensive explorations of the parameter spaces of interest to the researcher. The impact on biology and medicine has been particularly significant, since the high-throughput capabilities of these tools now make the routine collection of massive amounts of data a practical means of addressing biological complexity.
The field of tool development offers opportunities for chemical engineers to contribute to the development of next-generation instruments that will provide both fundamental and practical insights not possible today. For example, methods that track protein abundance dynamically inside a cell will provide unprecedented information about cellular function. In contrast, current protein analysis methods are rather simplistic, with capabilities limited to quantifying specific, predetermined proteins one at a time by antibody-based methods or mass spectrometry. Even if current proteomic methods were to be enhanced to become high-throughput, the technological basis would still be such that analysis could be performed only ex situ, requiring the removal of proteins from their native environment, with a two-fold undesirable consequence: some of the key information about in situ characteristics would be altered, and some of the essential features of protein–protein interactions would be lost. For proteins for which information on dynamic behavior is accessible, advances in visualization of such dynamic characteristics have yielded unique insights into cellular transport and cytoskeletal networks. Another example of tools that will have a significant impact on chemical engineering is dynamic in situ super-high-resolution microscopy, especially electron microscopy. Current transmission electron microscopy methods can provide only temporally averaged information.
The ability to track individual cells in real time in the body represents another opportunity to obtain potentially game-changing insight into the body’s metabolism in both healthy and diseased states. Current methods for assessing cellular composition can provide only infrequent snapshots of macroscopic information at discrete points in time. Histology remains the primary clinical mode of assessing the cellular composition of tissues. Such assessment is rather limiting because it provides only a two-dimensional static view of cellular composition. The ability to visualize cellular motion in the body in real time will provide useful information about the immune response to infections, sepsis, and metastasis, all of which involve the complex, coordinated motion of multiple cell types.
Tools for building materials with molecular-scale precision will open up new opportunities in nearly all fields associated with chemical engineering. With such tools, unique structural features that are currently infeasible will be possible because the tools will offer the precision and control of 3-D printing and allow building of materials at the individual-molecule scale, making it possible to control functional attributes and identify and address manufacturing defects in real time.
“On-chip” systems offer a cost-effective means of generating information, synthesizing materials, and screening them for their important interactions. Examples of such
systems include a chemical-plant-on-a-chip, human-on-a-chip, organ-on-a-chip, or colloidal-particle-generator-on-a-chip. (Opportunities for cell-, organ-, and organism-on-a-chip are discussed in Chapter 5.) Such chip-based methods offer many key advantages. From a synthesis point of view, they offer the advantage of small volumes and subsequently reduced costs. This attribute is helpful especially for research operations that aim to generate large libraries of materials in small quantities (for example, chemical libraries). Reduced scales are especially significant if the chemicals involved are toxic, since the on-chip systems use very low volumes of material. Such on-chip systems are also beneficial for colloidal synthesis. Traditional macroscopic methods of colloidal synthesis are often subject to a high degree of heterogeneity associated with interfacial phenomena. On-chip methods are very precise and have been used to synthesize colloids with more uniform dimensions. Such on-chip systems have also played an important role in the deployment of sensors that provide finer control over the flow and interactions of samples and reagents. Human-body-on-chips, which include systems comprising cellular chips representing one or more organs, are also poised to make a strong impact on drug development.
All on-chip systems share some common features and opportunities for impact from chemical engineering. First, microfluidics is a central component of all on-chip systems, and many of the fluids of practical interest (e.g., blood in the case of body-on-chips, or colloidal suspensions in the case of nanoparticle synthesis) are complex. Consequently, an understanding the flow of complex fluids in confined spaces is of prime importance. Second, all on-chip systems consist of a complex interconnection of a number of individual components, each complex in its own right. The design, analysis, and effective deployment of these systems offer opportunities for chemical engineers to contribute their expertise in fluid dynamics, reaction engineering, tissue and cellular engineering, and process systems engineering.
SENSORS
Sensors for Process Monitoring
Sensors play an important role in the design, development, and monitoring of processes and systems in all areas of chemical engineering. Development of “smart sensors,” “ubiquitous sensors” (tool or enabling technology depending on specific use), “smart actuators,” and “smart transmitters” hold the potential to transform a wide variety of manufacturing applications in the future. Such sensors can be classified broadly into those that measure physical parameters (e.g., temperature or volume), chemicals (e.g., reactants or cell culture media), or states (e.g., cell viability or particle sizes).
Physical Parameters
Sensors that measure such physical parameters as temperature, pressure, or flow rate have long been central to process monitoring in the chemical industry. With the advent of inexpensive, lightweight, and wearable types enabled by the development of flexible electronics, sensors that monitor physical parameters have found widespread applications. For the most part, sensors that monitor physical parameters are sufficiently mature and have already been incorporated into many practical applications. A key remaining opportunity moving forward lies in real-time analysis of data from such sensors for advanced process and product optimization. An emerging opportunity is in the adoption of these sensors and sensor networks for remote operation and remote laboratories. While remote operations have been used in the past largely for processes with safety concerns or with inherently limited access, the practical limitations imposed by COVID-19, which made remote operations inevitable, have brought this technology to the forefront not only for manufacturing processes but also for instruction in laboratory courses, representing an area of potential future impact for chemical engineers.
Chemicals
Sensors with which to measure the composition of chemicals play an important role in collecting molecular-scale information about a monitored system. For example, chemical analysis of reactants/products has long been used to assess reaction progress. Decades of advances in analytical tools have provided an array of methods (e.g., nuclear magnetic resonance [NMR], Raman spectroscopy, high-performance liquid chromatography, gel permeation chromatography, mass spectrometry [MS]) for assessing the molecular composition of complex chemical mixtures. Two key opportunities exist for the future. First, while the ability to measure (sense) chemicals in large laboratory equipment is practically unlimited, capabilities to do so using small sensors that can be readily inserted into the system for continuous monitoring are limited. Such sensors often require the development of new sensing mechanisms, robust hardware to implement those mechanisms, and novel technologies to enable continuous measurements in a seamless way. In addition, sensors for specific molecules (e.g., protein glycan groups) may require the development of molecularly and/or biologically based sensors interfaced with an appropriate device to generate the required measurement in the form of an electronic signal.
A second opportunity is the use of AI and ML to extract more meaningful information from complex data. Measurements offered by analytical tools such as NMR and MS are rich in information, and the information extracted from these measurements is heavily dependent on the question posed. The availability of new AI/ML algorithms can facilitate the extraction of new information from existing data, offering the opportunity to develop means by which new tools can extract information from preexisting data.
State
Another emerging opportunity lies in the capability to sense a state, which is in a relatively early stage of development. In this context, the “state” of a process or system refers in general to characteristics of the process or system that otherwise cannot be captured in a simple single parameter—for example, the viability of a phenotype of cells for application in protein or cell manufacturing, or particle-size distribution in a granulation or agglomeration process. Sensors capable of going beyond the current acquisition of physical parameter measurements have played and will continue to play a major role in the monitoring of chemical and biochemical processes. Future opportunities lie in the development of sensors for measuring the abundance of specific molecules and the establishment of a network of such sensors supported by advanced data analytics tools to enable optimum extraction of information contained in the massive quantities of acquired data. The development of self-learning, automated, self-monitored reactors is yet another opportunity that will depend critically on the availability of smart sensors.
Although significant progress has been made in real-time monitoring for quality control during production in dissolution, crystallization, drying, and other important unit operations, the implementation of similar monitoring during cell growth and protein production is lagging. For example, most antibodies and other therapeutic proteins require posttranslational modifications, such as glycosylation and disulfide bond formation. The product profile approved by the regulatory agency includes a range of allowed modifications typically not determined until postpurification. In addition, the extent of other, undesired modifications—such as oxidation, aggregation, charge variants, and truncation—needs to be determined and quantified because these modifications affect drug performance in end use. Two approaches for addressing these product characterization needs are (1) modifying existing analytical methods and using data science methods to determine the composition of complex mixtures (e.g., cell culture supernatant), and (2) developing altogether novel analytical optical and bioelectronic sensors. For example, infrared (IR)–based sensors are often used for measuring chemical composition, primarily because they can be used in situ for the most part. However, using Fourier transform infrared spectroscopy or near IR requires offline sensing and spectral deconvolution. Determining the level of contaminants, such as heavy metals, in a bioreactor feed is an important aspect of biomanufacturing. The development of sensors capable of providing these critical measurements requires not only development of the actual hardware of the sensor but also establishment of the fundamental parameters that define the state. Correct placement of sensors is another practical challenge that needs to be addressed. As new sensors are developed, proper placement within a network and the connectivity within the configuration will be central to successful implementation. The availability of transmitters to transmit the necessary data within the network with the requisite resolution will be critical. The development of such tools will require advances in hardware related to wireless transmission, as well as data analytics tools for efficient and effective information extraction.
Sensors for Health Monitoring
Design for Biological Applications
In the field of health monitoring, care for patients with diabetes has been impacted by sensor innovation through the development of continuous glucose monitoring technology. Monitoring of blood glucose levels, which, decades ago, depended on infrequent laboratory-based sampled measurements, is now carried out virtually continuously with wearable sensors (Lipani et al., 2018). This development has been made possible by significant advances in glucose sensing chemistries, a deeper understanding of the biocompatibility of sensors, and the development of electronics that enable a user-friendly patient interface. Such developments have transformed the sensing paradigm from “sample to the sensor,” to “sensor to the sample,” to “sensor on the patient.” Sensing technology was once based on large analytical equipment, requiring that the patient sample (e.g., blood) be withdrawn and shipped to a central analytical laboratory (e.g., laboratory-based glucose measurements). As sensors became smaller and portable, they went where the sample was (e.g., home-based glucose tests), which eliminated the sample shipment process. As sensors get even smaller, they can go directly on the patient (e.g., wearable glucose sensors), thus eliminating the step of collecting the sample and putting it into the sensor. These continuous sensors utilize a needle-based sensor inserted subcutaneously to measure the glucose concentration in the extracellular space just under the skin. Efforts have also been made to develop completely noninvasive means of measuring glucose concentrations using spectroscopic methods or noninvasive collection of tissue fluid through the skin, including sweat-based sensors. Sweat-based sensors measure the composition of various analytes present in the sweat (including such small molecules as glucose and hormones, and such large molecules as proteins) and use these measurements to assess the patient’s physiological state (Chung et al., 2019). Recent advances in sweat-based sensors are fueling optimism that the development of means of measuring additional analytes, including hormones, toxins, and allergic responses in the body, will likely have a similar transformative impact on health.
In addition to molecule-based diagnosis, wearable sensors now routinely perform a variety of other diagnostic measurements, such as temperature, blood oxygenation, and pulse (Gao et al., 2019). The ability to measure these key vitals through a wearable sensor has already transformed the collection of human physiological data, facilitating unprecedented acquisition of information about human responses in real-life situations in real time and continuously. Traditionally, these measurements were possible only using large, bulky sensors often connected to stationary electronic processors and displays.
Therapeutic drug monitoring is another area in which the impact of novel sensors can be transformative. Current drug-dosing regimens are rudimentary, based largely on average male body mass and drug trials that ignore the effects of race, ethnicity, gender, and many other patient-specific characteristics. The ability to measure drug concentrations in the body in real time can allow clinicians to achieve tighter control over
pharmacokinetics, thus potentially reducing harmful variability in drug distribution and preventing adverse events due to large excursions from desired concentration levels. Successful implementation of such technology will require the development of drug-specific, nonfouling, long-term implantable sensors that provide real-time feedback to clinicians. Significant progress is already being made in this regard. For example, an aptamer-based sensor that can measure certain chemotherapeutic agents has been developed and tested at the preclinical level. The challenges associated with expanding the scope of such technologies for a broad range of drugs offer future opportunities for chemical engineers.
Data Handling and Processing
The ability to extract new, better information from existing data promises to have significant impacts on health and medicine. As one example, consider medical diagnosis, which has long relied on qualitative analysis of images derived from magnetic resonance imaging (MRI), CT scans, radiology, and histology. Tools that enable better image processing can extract better information and yield more accurate diagnoses compared with qualitative human analysis. Studies have already demonstrated that AI-based analysis of histology is likely to be more accurate and less likely to miss a rare event. At the same time, AI-based analyses and diagnoses can help eliminate human bias in such procedures (although if not implemented correctly with appropriate training data, AI can itself amplify biases).
Extrapolation of current trends into the future suggests an ever-increasing capability of wearable sensors to collect even more massive amounts of data about human behavior in healthy and diseased conditions. These data will certainly include such basic vitals as temperature, pulse, and oxygenation, which in themselves are sufficient for certain applications. However, the available data could contain real-time measurements of such quantities as blood glucose, hormones as an indication of stress, and alcohol as a measure of intoxication, among others. Some of the technical challenges to overcome in realizing such capabilities include development of sensors that can work efficiently with small amounts of analytes that are available in sweat and determine compositions with appropriate accuracy.
A challenge also exists in correlating these measurements accurately with blood concentrations, in particular with respect to the transport of analytes from the blood into the interstitial fluid. An opportunity exists to collect massive amounts of data and establish correlations across the population in a way that is simply not possible through the collection of small amounts of data. Can model-based analysis be used to predict catastrophic physiological events from these data? Can drug reactions be better understood or predicted through appropriate analysis of such massive amounts of data? Given the well-documented challenge posed for drug design and development by the heterogeneity of drug response across a population, the prospect of personalization of drug therapies presents an exciting opportunity for reducing adverse events without compromising therapeutic efficacy. However, actualizing this concept will require having adequately informative data available to support such personalization at the design stage and the
reduction of adverse events at the follow-up stage. Continuous physiological monitoring offers the potential for producing precisely this sort of data.
CHALLENGES AND OPPORTUNITIES
The development of tools that synthesize available data in real time and frameworks or models that transform data into information and actionable knowledge could become one of the key contributions of chemical engineering to society over the next decades. It is easy to imagine a not-too-distant future characterized by data-on-demand—where data on anything, at any level of granularity, will be readily and instantly accessible. Such a future suggests profound and exciting opportunities for chemical engineers, who are trained in process integration and systems-level thinking—skills that will be required to synthesize disparate data streams into information and knowledge.
The systems thinking, analytical approaches, and creative problem-solving skills of today’s chemical engineering graduates give them a distinct advantage in using AI in real-world contexts. The evolution of AI in the next decade will have enormous implications not only for the types of problems chemical engineers will be able to solve but also for how they will develop those solutions. Chemical engineers are poised to contribute significantly to the development of modeling and simulation tools that will influence education, research, and industry. They will continue developing and disseminating methods, algorithms, techniques, and open-source codes, making it easier for nonexperts to use computing tools for scientific research.
The increasing operational complexities and shrinking economic margins in the petrochemical industry, coupled with stringent environmental and quality demands on the manufacture of specialty chemicals and polymers, will continue to drive the increased use of modeling and simulation. While the pharmaceutical industry currently lags behind the chemical industry in its use of simulation tools, fundamental changes in regulatory requirements are motivating its greater use of mathematical models and simulation, especially in the rapidly growing biomanufacturing sector.
Recommendation 8-1: Federal and industry research investments should be directed to advancing the use of artificial intelligence, machine learning, and other data science tools; improving modeling and simulation and life-cycle assessment capabilities; and developing novel instruments and sensors. Such investments should focus on applications in basic chemical engineering research and materials development, as well as on accelerating the transition to a low-carbon energy system; improving the sustainability of food production, water management, and manufacturing; and increasing the accessibility of health care.