4
How Do Living Systems Navigate Parameter Space?
Any attempt at a “realistic” description of biological systems leads immediately to a forest of details. Making quantitative predictions about the behavior of a system seems to require knowing many, many numerical facts: how many kinds of each relevant molecule are inside a cell; how strongly these molecules interact with one another; how cells interact with one another, whether through synapses in the brain or mechanical contacts in a tissue; and more. The enormous number of these parameters encountered in describing living systems is quite unlike what happens in the rest of physics. It is not only that as scientists we find the enormous number of parameters frustrating, but the organism itself must “set” these numbers in order to function effectively. Many different problems in the physics of living systems, from bacteria to brains, revolve around how organisms navigate this parameter space through the processes of adaptation, learning, and evolution.
As will be familiar from examples in previous chapters, the biological physics community has made progress on understanding adaptation, learning, and evolution by engaging with the myriad details of particular examples. But standing behind these details is an approach to living systems more generally. The physicists’ approach to describing any particular functional mechanism invites us to see that mechanism as one possibility drawn from a large space of alternatives. In this view we think not about one system but about a distribution or ensemble of systems, much as in the statistical physics of disordered systems. Crucially, the relevant ensembles of biological mechanisms are neither random nor truly disordered, but
sculpted by the constraints of functionality. The work surveyed in this chapter includes different approaches to characterizing these functional ensembles, and the dynamics through which these ensembles are selected. Table 4.1 provides an overview of these problems.
ADAPTATION
When we step from a dark room into the bright sunlight, we are momentarily blind, but then the cells in our eye “adapt.” More generally, sustained, constant sensory inputs tend to fade away. During adaptation, the concentrations of various molecules in the individual cells of our eye are changing, in effect shifting the parameters that describe the response of these cells to light. Related forms of adaptation happen in all cells as they respond to signals in their environment.
TABLE 4.1 Navigating Parameter Space
Discovery Area | Page Number | Broad Description of Area | Frontier of New Physics in the “Physics of Life” | Potential Application Areas |
---|---|---|---|---|
Adaptation | 159 | Understanding how living systems deal with signal spread across an enormous dynamic range, with changing statistical structure. | Near-perfect adaptation without fine tuning; optimal coding and adaptation to input statistics; from control of gene expression to sloppy models. | Adaptive sensors. |
Learning | 166 | Formalizing and quantifying colloquial ideas of learning in animals, humans, and machines. | Statistical physics of inference; phase transitions and “aha;” connecting molecular complexity to learning algorithms. | Neural networks in artificial intelligence; principles of machine learning; natural language processing. |
Evolution | 170 | Taming the complexity of evolutionary dynamics through targeted experiments and simple models; bringing this understanding back into the real world. | Evolutionary dynamics are driven by the tails of distributions; finding regimes where evolution is predictable; new experiments to track thousands of coexisting strains of microbes; the immune system as evolution in microcosm. | Vaccine design; tracking epidemics. |
Sensory Adaptation
Some of the earliest experiments on the responses of single sensory neurons showed that the perceptual fading of constant inputs has a corollary in a slow decay of the neural response to the same constant inputs. But adaptation is more than subtracting a constant. In many photoreceptor cells, for example, constant background light results in a reduction in the amplitude of the response to each additional photon along with a speeding up of this response. These effects are linked through the molecular cascade that amplifies the single photon response (see Figure 1.11), since the gain of the system depends on the time that individual molecules spend in their active states. This picture of scaling amplitudes and time scales in the adaptation of photoreceptor responses was understood and connected quantitatively to experiment even before the identity of the components in the cascade were known, testimony to the power of phenomenological theories.
Neurons that respond to sensory inputs also adapt to statistical features of these inputs beyond their mean. Single cells at the output of the retina, for example, adapt to the dynamic range over which light intensities are varying, and to the spatial and temporal correlations in these variations. In some cases, these adaptive dynamics can be traced down to the dynamics of particular ion channels, which have long time scales of inactivation after opening during an action potential. Similarly, at different stages of the auditory system neurons adapt to the dynamic range and temporal statistics of incoming sounds. Adaptation to input statistics is predicted by theories of neural coding that maximize the information captured in a limited number of action potentials given that the natural sensory world has intermittent dynamics (Chapter 2), and in some cases it has been possible to show that the form of adaptation really does optimize information transmission, quantitatively.
Although adaptation often is described as a strategy for dealing with slowly changing signals, these changes in response to changing input statistics can be so fast that they are difficult to resolve; reliable changes in the form of the response can occur essentially as soon as the system can reliably infer that the distribution of inputs has changed. More generally, efficiency arguments suggest that the time scales of adaptation can be related to the time scales on which the statistical features of the environment vary. This happens, even in the responses of a single neuron to injected current (see Figure 4.1). In this case, at least, more detailed analyses show that the dynamics are best described not by a single adaptation time scale that changes in response to the inputs, but instead by a near continuum of time scales in parallel. The result is that adaptation discards constants by taking a fractional derivative, or equivalently by comparing signals with a memory of the past that decays only as a power-law.
Adaptation can be seen not only in neural signal processing but also in the sensory responses of single celled organisms, such as bacterial chemotaxis (Chapter 1). In chemotaxis, adaptation makes the cell’s probability of running or tumbling
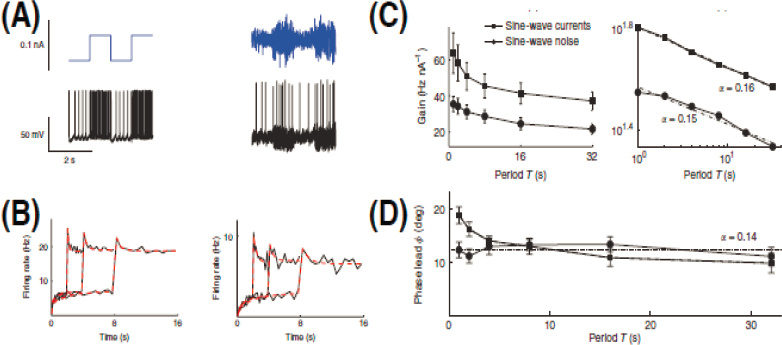
sensitive to the derivative of the concentration of attractive or repellent molecules, ignoring the absolute value. Theory makes clear that this is an essential part of the cell’s strategy for advancing up the concentration gradient, and careful experiments show that the adaptation is nearly exact, so that, for example, after a step increase in concentration the behavior returns precisely to its baseline. Early models for adaptation envisioned two parallel pathways of response to input, one fast and one slow, which are combined with opposite signs to generate the final output. In this broad class of mechanisms, perfect adaptation requires fine-tuning of the responses in the two pathways so that they cancel, which seems implausible. An alternative is adaptation through feedback, which in some limits can ensure that any steady output is at a fixed level independent of inputs. Detailed analysis of the molecular events in Figure 1.12 shows that this in fact is what happens, providing a concrete example of how complex biochemical mechanisms are sculpted by the cell’s need to solve the physics problems involved in climbing the gradient.
Adjusting Gene Expression and Protein Copy Numbers
In chemotaxis the adaptation mechanism involves biochemical reactions, crucially the attachment and detachment of methyl groups on the receptor by the enzymes CheR and CheB. These reactions occur on the time scale of seconds. Cells can also adapt to their environment more slowly by changing the levels of gene expression, or equivalently the number of copies of different proteins. These crucial regulatory processes were discovered by studying the way in which bacteria respond to changes in the available nutrients. For a generation, the focus of these explorations was on the regulation of single genes in bacteria, for example the gene for one crucial enzyme in the consumption of a particular sugar. This work provided the paradigms for how information encoded in regulatory sequences of DNA (Chapter 2) and information encoded in the concentration of transcription (Chapter 2) combine to control the expression of one gene.
In many cases, it is interesting to ask how the expression levels of many genes are coordinated to accomplish functions. Part of the problem is to understand the landscape of performance as a function of the many expression levels. One important example of this is the simple conversion of nutrients into growth or biomass, where the relation between the number of enzyme molecules and the conversion rate is determined by the classical chart of biochemical reactions in core metabolism. A more subtle example is the electrical activity of neurons, which depends on the numbers of different ion channel proteins through equations that are known very precisely.
The human genome, like that of many animals, encodes more than 100 types of channels, and a single neuron chooses perhaps seven different kinds of channels from this large set of possibilities. Each channel type itself is described by many parameters, including the rates at which they open and close, controlling the flow of electric current into the cell, and the dependence of these rates on the voltage across the cell membrane. The result is that just one neuron is described by 40 or even 50 numbers, which can be different in every cell, and there are billions of cells in the brain.
One can measure the properties of individual channel molecules, but it is more difficult to make an independent measurement of the number of channels of each type that are in the cell membrane. Thus, at a minimum, describing the electrical dynamics of neurons requires us to fit these numbers to the overall behavior of the cell. By the 1980s, building these sorts of models was a major activity in the neuroscience community. It was known, but not widely discussed, that inferring the number of each type of channel was a challenging problem.
Our mathematical description of ion channel dynamics rests on a firm foundation, one of the classical chapters in the interaction of physics and biology, as described in Chapter 2. The result of all these developments is that, in contrast to
the typical situation in describing networks of interacting proteins in a cell, the equations that describe the dynamics of ion channels and their interaction with the voltage across the cell membrane are known quite precisely. In the 1990s, theoretical physicists suggested that relying on this knowledge allows a new approach: rather than thinking of these as models tuned to describe the behavior of particular cells, one can take the family of models seriously as a theory of possible cells.
If cells are committed to making particular types of channels, then the universe of possible cells is defined by the number of each channel type that is synthesized and inserted into the membrane to become functional. This theoretical space of possibilities is the same space that real cells explore as they control the expression of ion channel genes; see the discussion of these control mechanisms in Chapter 2. Figure 4.2 shows the behaviors in different slices through the space of possible cells. Importantly the calculations start from combinations of ion channels that occur in particular, well-studied neurons.
Relatively small changes in the number of channels can lead to qualitative changes in the pattern of electrical activity, and this is more obvious in the high-dimensional space of possibilities facing the cell. On the other hand, there are directions in this high-dimensional space where variations in behavior are modest. Each time the cell generates an action potential, calcium ions enter the cell, and these ions are pumped back out (or into internal storage spaces) more slowly, so that the calcium concentration provides a record of electrical activity; this is what makes possible the use of calcium-sensitive fluorescent proteins to monitor the electrical activity of neurons, as in Figure 3.13. Of all the ions that contribute to electrical current across the cell membrane, calcium is special because it both carries electrical current and serves as a signaling molecule for various biochemical processes inside the cell. This immediately suggests that cells could tune their pattern of electrical activity by increasing or decreasing the synthesis of particular ion channels in response to changing internal calcium concentration. Real molecular mechanisms will be sensitive to concentration averaged over some limited time, and by using mechanisms that have different averaging windows the cell could achieve even more fine-grained control.
The theoretical proposal that neurons regulate the number of channels in response to their own patterns of electrical activity was confirmed almost immediately by experiments in a variety of systems, from the small networks that generate digestive rhythms in the crab gut to cells in the mammalian cerebral cortex, responsible for our thoughts and actions. The exploration of this phenomenon, and its underlying mechanisms, has become a substantial effort in the mainstream of neurobiology, spreading far from its origins as a theoretical physics problem. There are many potentially general lessons.
First, if there is pressure for the organism to achieve certain dynamical behaviors, but no explicit preference for one molecular implementation over another, then
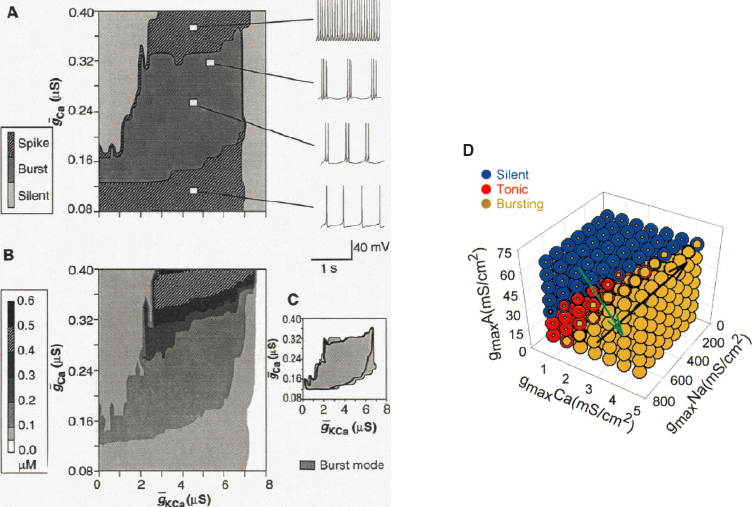
there is no single channel protein whose number needs to be controlled precisely. This is not because cells are incapable of precise control (see, e.g., Chapter 2), but rather because the mapping from molecular mechanisms to macroscopic functions is many-to-one, echoing the ideas of Chapter 3. Second, what needs to be controlled are not the numbers of individual channels, but rather combinations. This predicts that while protein copy numbers are variable, correlations in these fluctuations carry
the signature of functional constraints. Finally, different combinations of protein copy numbers have vastly different impacts on functional behavior.
Sloppy Models and Reduced Dynamics
A different approach asks not about particular settings for parameters of the system, but more explicitly about the distribution or ensemble of parameters that are consistent with the observed behavior. This corresponds to constructing a statistical mechanics in parameter space, and predicts the distributions, for example, of protein copy number variations that are seen in real systems. This distribution has a geometry, being compact along directions that correspond to combinations of parameters whose variation generates big effects, and broad along directions that have small effects (see Figure 4.3). The surprise is that these distances in parameter space are almost uniformly distributed on a logarithmic scale: There is one combination of parameters that is most tightly constrained, another which is allowed to vary twice as much, another four times as much, and so on. It has been suggested that systems with this sort of behavior form a well-defined class of “sloppy models,” neither robust nor finely tuned but with a full spectrum of parameter sensitivities. There is a substantial effort underway to understand the origins of this behavior, its connections to other ideas in statistical physics, and its implications for the dynamics of adaptation. It would be exciting to connect these ideas with other examples in which many-to-one mapping arise, such as the sequence/structure mapping for proteins (Chapter 3).
The theory of dynamical systems provides us with settings in which behaviors become universal, and thus explicitly independent of most underlying parameters. If we think, for example, about models for genetic networks that can describe a developing cell making choices among alternative fates, then in the neighborhood of the decision point the dynamics takes a stereotyped form. Building outward from these decision points allows construction of a geometrical model for the dynamics more globally, in which coordinates are abstract combinations of gene expression levels. As it becomes possible to follow gene expression levels through the steps of cellular differentiation, this approach makes it possible to search for the simplified collective coordinates and to classify the impacts of perturbations, with almost no free parameters.
Perspective
Some form of adaptation occurs in almost every living system, matching its behavior to relatively short-term variations in the demands of the environment. The phenomena range from the gradual fading of constant sensory inputs to the intricate control of gene expression, and more. Even seemingly simple examples
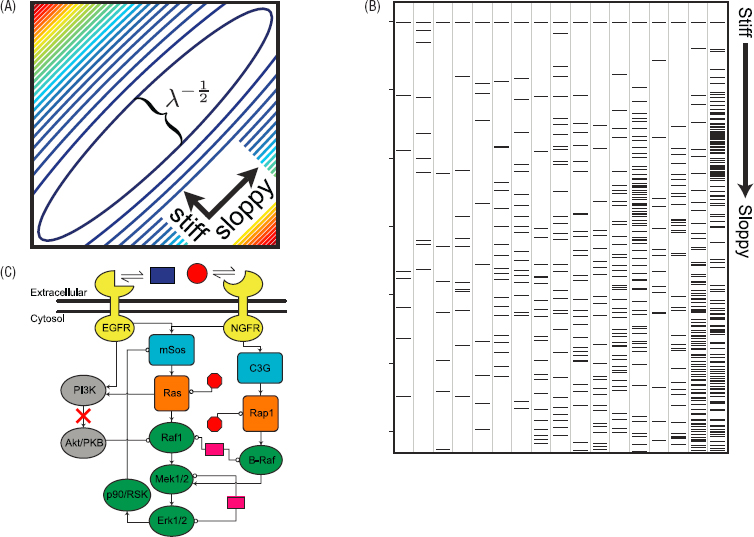
are deeper than they first appear, and new forms of adaptation—often suggested by theory—continue to be discovered. This circle of ideas provides some of the most concrete examples of the idea that real living systems should be seen as examples drawn from a larger set of possible systems. There is a vigorous theoretical effort to understand how and when the high-dimensional parameter spaces of these systems can be collapsed to lower dimensionality, a kind of emergent simplicity. In the coming decade we can hope to understand whether this simplicity is generic, or whether it is itself selected by evolution.
LEARNING
The word learning is used, colloquially, to describe many things—learning a language, learning a rule, learning to play a musical instrument, learning physics.
There is a long history of both psychologists and computer scientists formalizing these colloquial ideas, in the hopes of describing human behavior or learning machines. The biological physics community entered the subject through models for networks of neurons, as described in Chapter 3.
Statistical Physics Approaches to Learning
The functions that are accomplished by a neural network are determined by the strengths of connections or “synaptic weights,” among all the neurons in the network. An important idea, which echoes ideas from many different sections of this report, is that one should think not about particular settings of the synaptic weights, but about a probability distribution over these weights. Given only a limited number of examples of what the network should be doing, such as assigning names to images of faces, then there are many combinations of synaptic weights that are consistent with these examples. There may also be some tolerance for error. If one makes the analogy between error and energy, so that low energy states are close to the correct answer, then a tolerance for error is analogous to temperature.
For real materials, it is natural to plot a phase diagram (e.g., mapping gas, liquid, and solid to different parts of the plane defined by temperature and pressure). Statistical mechanics teaches us that these sharply defined phases emerge from a probability distribution over all the microscopic states of the material in the limit that number of atoms or molecules becomes large. For neural networks, there thus will be sharp phases when the number of neurons or connections becomes large, which surely is relevant for real brains. Natural coordinates for the phase diagram are the tolerance for error and the number of examples that the learner has seen. In the simplest perceptron model—where a single neuron takes many inputs and classifies them into two groups—not only is there a phase transition into the state where the network has learned the correct classification, but in this case the transition is discontinuous (first order) so that the fraction of errors drops abruptly as the network is exposed to more and more examples, as if it experienced an “aha!” moment.
Learning can be thought of as the inference of some underlying parameters (e.g., the synaptic weights) from given data (e.g., examples of correct input/output pairs). Parallel to the statistical physics of learning is the statistical physics of inference. This is interesting as an approach to inference problems solved by the brain, but also as a way of thinking about data analysis and data acquisition strategies such as compressed sensing. Closing the circle are ideas about how olfactory signaling, for example, may instantiate compressed sensing. Statistical physics approaches to the original problem of learning in model neural networks have had a resurgence in response to the deep network revolution in artificial intelligence (Chapter 7), but it is too soon to say how these ideas will influence thinking about the brain itself.
Perhaps the most dramatic example of learning by the human brain is the learning of language. Instead of thinking about a probability distribution over synaptic weights in a network of neurons, we could think of a probability distribution over the parameters of model languages. In particular, if we have grammatical structures that are defined by rules (e.g., replacing nouns by noun phrases), there are probabilities that these rules will be applied in constructing long grammatical statements. Recent work shows that ensembles constructed in this way have phase transitions as a function of the natural parameters in the underlying rules, and that the different phases can be distinguished by their order or correlation. In this approach, then, there is a phase transition between a kind of incoherent babbling and the construction of potentially meaningful sentences. These are first steps in a long and ambitious program.
Connecting with Real Neurons
The introduction of probabilistic ideas into learning also has had implications for the design and analysis of experiments. Songbirds learn their songs, and continue listening to their own songs to stay in tune. If this auditory feedback is disrupted, songs will drift. More systematically, experimentalists can play noise to the bird whenever he sings a note below a certain pitch, and the bird will learn to compensate, driving the pitch upward over a period of hours or days (see Figure 4.4). Under steady conditions, the distribution of pitch across multiple examples of a single note gives a measure of the bird’s own tolerance for errors, in the language of statistical physics models for learning in networks. Experiments show that this distribution has long tails, which means that small deviations from the correct pitch are heavily penalized, but this becomes less steep at larger deviations. Placed in the larger theoretical context of learning, this implies that the bird will have difficulty following cues that would drive larger, immediate changes, but could easily follow repeated small changes over the same total excursion. This sort of behavior is seen in many learning problems, and the songbird experiments agree quantitatively with experimental predictions.
Rather than using theory to understand macroscopic learning behaviors, other groups in the biological physics community have tried to push down to the molecular events at real synapses to understand how learning rules are implemented. Part of what was so exciting about the very first symmetric models for neural networks is that they could learn a new memory by changing the synaptic weight between two neurons in proportion to the correlation between their activities. This is a mathematically precise version of an old idea in the biological and even psychological literature that neurons that “fire together wire together.”
A deeper theoretical examination of synaptic dynamics, however, shows that limits on the number of distinguishable states of individual synapses quickly leads
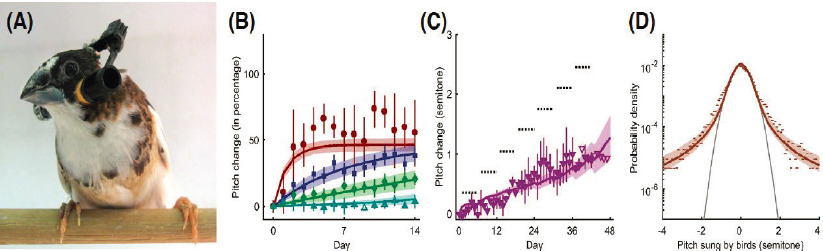
to new memories overwriting the old if the network continues learning over an organism’s long lifetime. This problem can be solved if the synapses themselves have dynamics with multiple time scales, as in Figure 4.5. It is known that molecular mechanisms involved in changing the strengths of synapses have many, many molecular components, and so more complex dynamics are to be expected. What is important is these dynamics are not just more complicated but need to be selected to have properties that solve a physics problem faced by the network. There is an important challenge in connecting more detailed experiments on the microscopic mechanisms of synaptic plasticity to a larger theoretical framework for learning in networks. Experimental studies that have tracked synaptic dynamics in the live mammalian brain suggest that the adult neocortex, which is thought to store some memories for the adult lifetime, has different subsets of synapses with different lifetimes, in the spirit of these theoretical considerations.
Perspective
The study of learning has had many independent lives: in psychology and animal behavior; in mathematics and computer science; and in neurobiology, genetics,
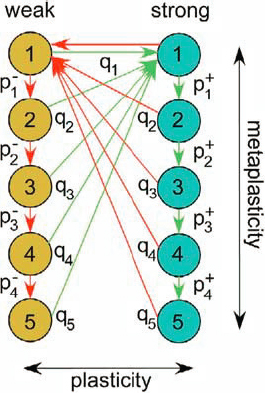
and pharmacology. All these groups have touched different aspects of the problem. The biological physics community is unique because it has engaged with learning at all levels, from the molecular events at synapses to animal behavior, through both theory and experiment. This is important, because while there has been great progress in each of the many directions, major open questions exist about how these different directions are connected: How is molecular complexity at the synapse related to the efficacy of learning? How do networks learn effectively when the number of synaptic connections is much larger than the number of examples that the animal—or the artificial neural network—has seen? The biological physics community is playing a key role in sharpening these questions, and it is reasonable to expect substantial progress over the coming decade.
EVOLUTION
Over longer time scales, evolution can change almost anything in biology, from the rules of the genetic code to the structure of proteins, the logic of gene regulatory networks, and the ways in which organisms learn. The fact that all living systems
have arisen through such an evolutionary process imposes a general constraint that tunes parameter values throughout the rest of biology. This makes evolutionary optimization a key simplifying principle throughout biology: Biological systems have purpose and function to the extent that evolution can measure and select for those properties, and they navigate to highly constrained regions of parameter space to do so. The biological physics community began to engage more deeply with these issues at the start of the 21st century, starting by developing theories for evolutionary dynamics in the simplest possible contexts. Even these simple examples had surprises, and natural formulations as statistical physics problems in which the mean behavior of a population is controlled by the extreme tails of the distribution over individuals. From this has grown a vigorous program of both theory and quantitative experiment, connecting abstract ideas from physics to the detailed behavior of real organisms.
Statistical Dynamics in Fitness Landscapes
In the extreme, it is possible that evolution selects for functional performance close to the relevant physical limits. Examples of near optimal performance include the diffraction-limited optics of insect eyes, photon counting in vision and molecule counting in chemotaxis (see Figures 1.11 and 1.12), and more. There are many efforts in the biological physics community to turn these observations into theoretical principles from which aspects of system behavior and mechanism can be derived, as described above. It is even possible to imagine optimization principles for evolutionary dynamics itself. The mutation rate in copying DNA from one generation to the next is reduced by proofreading mechanisms, analogous to Maxwell’s demons (Chapter 2), but this comes at an energetic cost. The rate of evolution itself is subject to evolutionary change, and this leads to the regular appearance of “mutator” strains of bacteria that can adapt more rapidly to novel and stressful environments.
A corollary of ideas about optimization is that the parameters of living systems should not be more constrained than is required to reach some criterion level of function. In large families of proteins, for example, patterns of conservation and diversity allow us to identify regulatory elements and highlight proteins and protein domains that have particular functional roles. Correlated patterns of amino acid substitutions within a protein family can be a signature of natural selection to restore physical interactions between residues that are in contact in the three-dimensional structure of the protein. As discussed in Chapter 3, researchers from the biological physics community have used methods from statistical physics to describe the distribution of sequences as being as variable as possible consistent with the observed correlations. This leads first to the possibility of drawing new sequences from the distribution, circumventing the sequential nature of normal
evolution and synthesizing new proteins; a strong confirmation of this theoretical framework is that a large fraction of these new proteins fold and function. Analysis of the models allows a disentangling of direct physical interactions from indirect correlations, and this information can be used to predict the three-dimensional structure of the corresponding proteins.
The conditions under which evolutionary dynamics allow for optimization are much less clear: Evolution does not itself have a direction or purpose. Instead, macroevolutionary processes are the collective outcome of an enormous number of individual cell divisions, each of which can introduce errors due to random mutations, combined with the effects of natural selection and genetic drift that act on this novel variation. The nature of available genetic variation does not necessarily allow for full exploration of the relevant parameter space, and even in cases where such variation exists, evolution cannot always favor it. For example, in small populations there are important limits to the efficiency of natural selection, and evolution can actually in some circumstances lead to a degradation in function. The biological physics community has played a key role in recent efforts to understand these dynamics of stochastic evolutionary processes and the limits they place on optimization arguments in living systems.
An important contribution from the biological physics community has been the calibration of ideas about evolutionary dynamics through the study of simplified models. Perhaps the simplest evolutionary problem is a finite population of organisms that can always mutate to achieve slightly higher fitness. But as mutations arise and compete with one another, beneficial mutations can go extinct before reaching sufficient population size to take over the population as a whole. At the start of the 21st century it was realized that in these problems the evolution of the population as a whole is driven by the tail of the distribution of high-fitness individuals, connecting to other statistical physics problems; even the overall rate of evolutionary progress toward higher growth rates has a subtle dependence on population size, mutation rates, and the selective advantage of each mutation. More recently it has been possible to bring similar rigor to the analysis of much more complex evolutionary scenarios; examples include spatial structure, fluctuating environments, and perhaps most importantly the interactions between evolutionary and ecological dynamics that create and maintain diverse communities of organisms.
The statistical physics approach to evolutionary dynamics identifies multiple regimes for these dynamics, two examples of which are illustrated in Figure 4.6. Although the engine of evolution is random mutation, there are regimes in which the future trajectory of evolutionary change can be predicted. There is evidence that viruses which infect humans, such as those that cause COVID-19 and the seasonal flu, are in this regime. In an unexpected turn, the biological physics community’s approach to evolution thus has clear practical implications, in particular for the design of vaccines, as discussed in Chapter 7.
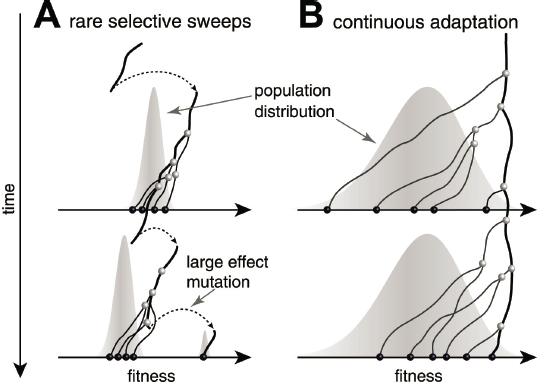
Evolution as an Experimental Science
The explosion in genome sequencing has turned observations of evolution in the wild into a data rich, quantitative enterprise. It is now relatively straightforward to sequence near-complete genomes from thousands of individuals from essentially any species, including humans, and use the observed patterns of genetic variation within the population to infer key aspects of evolutionary history. This enterprise has helped us understand the demographic history of humans, leading to productive exchange between evolutionary biologists and archaeologists, and has shed light on adaptation in response to pathogens such as malaria and the plague. In other species, genome-based investigations of evolutionary history have led to insights on population structure, local adaptation, and speciation.
In microbial and viral populations, we can track evolutionary changes by sequencing populations as they adapt to the human gut or as viral epidemics spread across the world. This allows us to go beyond inferences of evolutionary history from sequence data in the present, and to observe evolution acting in real time. Technology for analyzing ancient DNA offers the promise to directly observe change through time in populations where this would otherwise be impossible, such as humans.
However, all of these studies of natural populations are inherently observational. While we sometimes have the opportunity to track multiple populations—observing intra-patient evolution of HIV across many infected individuals, or tracking the evolution of Pseudomonas infections across multiple cystic fibrosis patients—it is impossible to precisely control parameters or conduct replicate studies. Thus to make evolution an experimental science, there have been parallel efforts to bring evolution into the laboratory.
Laboratory evolution experiments have been conducted in a wide range of organisms, including obligately sexual eukaryotic organisms such as the fruit fly Drosophila melanogaster and the nematode Caenorhabditis elegans. However, microbial and viral populations are in many ways the ideal model systems for these studies. In these organisms, we can conduct hundreds or thousands of evolution experiments in parallel and we can freeze clones or whole-population samples to create a “fossil record” for future study. Unlike the actual fossil record, these frozen samples can actually be resurrected later and compared directly to their descendants. Microbes and viruses have manageable genome sizes that make it possible to sequence many individuals from many replicate populations through time at a reasonable cost, and in many of these organisms, genetic tools allow manipulations such as inserting fluorescent markers or elevating mutation rates. Finally, we can leverage our deep understanding of cell biology and genetics in some of these organisms to interpret results in a functional context.
The most well-known and deeply studied evolution experiment is the long-term evolution experiment (LTEE). As discussed in Chapter 6, this study has propagated 12 initially identical independent replicate populations of the bacterium Escherichia coli in the same environment since 1988, a total of nearly 75,000 generations to date. This experiment originated in the evolutionary biology community, and has been used as the basis for important insights into the evolution of novelty, the interactions between ecological and evolutionary dynamics, the evolution of mutation rates, and many other questions. It has also generated a rich data set (including a fossil record of samples frozen every 500 generations) for the broader community, and biological physicists have played a key role in analyzing these data and making connections to theory.
While the LTEE is a remarkably rich resource, it involves only 12 replicate populations of a single organism evolving in response to a single selective pressure. Because evolution is an inherently stochastic process, numerous groups have made efforts to expand the scale of replication in order to quantify the probabilistic outcomes of adaptation, and have explored the generality of conclusions in other organisms and environments. The physics of living systems community has played a central role in many of these efforts, both in developing theoretical predictions and in conducting more highly replicated experiments that allow for closer quantitative comparison between theory and experiment.
Guided by theoretical studies showing that microbial populations are often in a regime where small fractions of the population can play a critical role in driving evolution of the population as a whole, and that large changes can result from the accumulation of many small advantages, one important experimental effort has been to push for higher resolution and more precise phenotypic measurements. This has included developing new methods to detect strains of single celled organisms that constitute only one ten-thousandth of a large population, and measuring the relative fitness of mutants to within one part in a thousand. As in other areas of physics, profound tests of our understanding come from precision experiments, but not so long ago, it would have been difficult to imagine such precision measurements on the dynamics of evolution itself.
Theorists from the physics of living systems community have also worked to extend models of evolutionary dynamics to include important additional features, such as the role of recombination within linear genomes, the emergence of ecological interactions, and the effects of spatial structure. These same theorists have established connections with experimental groups to test these theoretical ideas (or, in several cases, conducted experiments themselves). For example, this has led to major advances in our understanding of adaptation in spatially expanding populations, with implications both for microbial populations and for evolution more generally (see Figure 4.7). This combination of theory and experiment in the study of evolution is an example of how the intellectual scope of biological physics has expanded in the 21st century.
The Immune System
Many of the conceptual issues in evolutionary dynamics are illustrated in microcosm by the adaptive immune system. To respond to the enormous range of challenges from our environment, the immune system synthesizes billions of distinct types of antibody molecules. In contrast to other protein molecules, the genome does not contain the precise instructions for making these antibodies. Instead, the genome has multiple sequences for separate V, D, and J segments of an antibody, and individual cells in the immune system edit their own DNA to combine one of each segment into a full sequence. This recombination process is an important example of chromosomal dynamics (Chapter 3), and in this process random lengths can be deleted from the ends of the V, D, and J segments, and random nucleotides can be inserted. Further steps in the development of the antibody repertoire include removing those sequences that encode antibodies against the body’s own molecules, as well as hypermutation in some cells to further diversify the population. The many different antibodies can bind to different kinds of molecules and molecular fragments from invading viruses or bacteria, and those cells making antibodies that engage in these binding events generally reproduce more
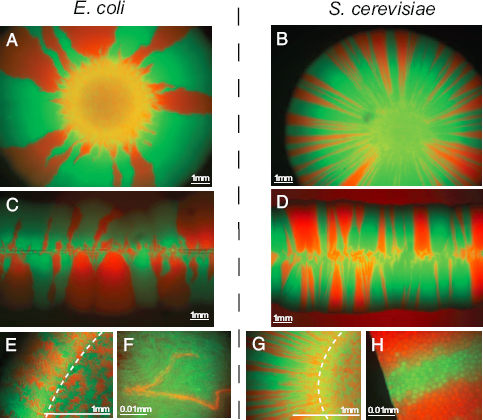
quickly. Thus, the antibody repertoire evolves over the lifetime of the organism, with diversity being generated at random and subject to both positive and negative selection pressures.
In the late 2010s, the exploration of antibody diversity was revolutionized by the possibility of using DNA sequencing to make deep surveys of antibody diversity in single organisms, first in model systems such as zebrafish and then in humans. The biological physics community pioneered these experiments, along with the theoretical analysis that followed. Over the course of a decade, precise probabilistic models were developed for the generation of diversity, providing a framework for inferring the parameters of recombination, deletion, and insertion events (see Figure 4.8). This theoretical work shows that the total entropy of antibody sequences is large (nearly 50 bits in humans), so that the actual repertoire at any moment is only a small sampling from the set of possible antibody molecules. Furthermore, only ∼20 percent of this entropy arises from the combinatorial choices of V, D, and
J regions from the genome; the overwhelming majority of the diversity comes from the random insertion and deletion events. This is important because the enzymes that catalyze insertion and deletion can be controlled, and indeed there are clear connections between these controls and changing patterns of antibody diversity from embryonic to adult life.
Quantitative understanding of how diversity is generated provides a foundation for measuring selection in response to infections and leads to the surprising conclusion that the mechanisms for generating diversity already are biased strongly toward sequences that turn out to be functionally relevant in fighting infections. There are interesting theoretical questions about what it means for the antibody repertoire to be well matched to the distribution of possible challenges from the environment, with connections to other living systems that must represent information about the world with limited physical resources (Chapter 2). The enormous entropy of sequences means that most will be unique to individual members of a species even if they share the same genome. But the distribution is predicted to have anomalously large fluctuations in the probability itself, so that some sequences are overwhelmingly more likely than others. A crucial test of this prediction is to predict the probability that different individuals will share the same sequences. As shown in Figure 4.8B, in a group of hundreds of people, most sequences will be unique to each individual, but hundreds of sequences are shared among a large fraction of the group. This pattern is in excellent agreement with theoretical predictions. This is an especially interesting example because it makes explicit how the biological physics community has been able to tame the variability across organisms—in this case, humans—even to the point of showing how parameter-free predictions for the distribution of this variability arise from more fundamental theoretical considerations.
The examples of evolution in the immune system discussed so far involve the analysis of snapshots of the system at single moments in time. It is more challenging to get at the dynamics. An important test case for these ideas is also an important human health problem, HIV infection. As discussed in Chapter 7, input from the biological physics community played an important role in realizing that HIV evolves rapidly, even over the lifetime of a single patient, and that effective treatments should take this into account. More recently, it has become possible to sequence samples from the population of viruses over time in many individual patients, sometimes in conjunction with surveys of the patient’s antibody repertoire. These experiments in the clinic provide an unusual opportunity to observe fundamental dynamics of co-evolution between the virus and the immune system. Ideas from statistical physics have been used to make clear that this process is far from equilibrium, and to disentangle the flow of information from the virus into the immune system versus the reverse process where the immune system is driving evolution of the virus.
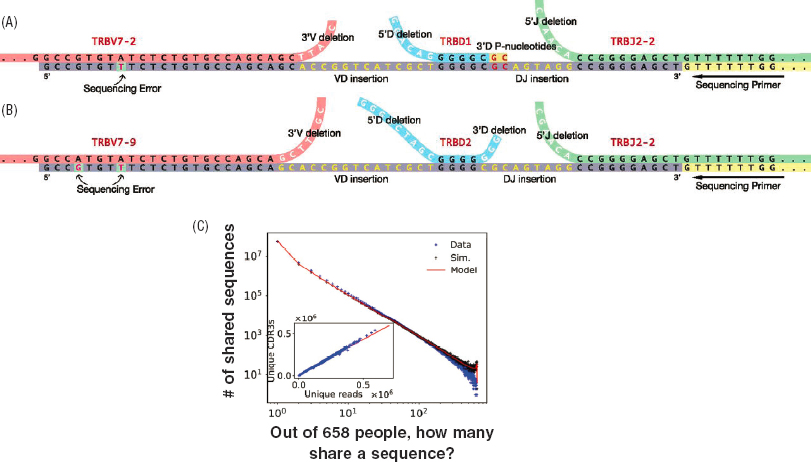
Perspective
The evolutionary relatedness of all living systems is a unifying theoretical principle on which physicists and biologists can agree. The biological physics community has engaged with evolution, both theoretically and experimentally, in several ways. Ideas of optimization, discussed in many sections of this report, represent an attempt to predict the outcome of evolution while circumventing its dynamics. In the opposite direction, many theoretical and experimental observations are concerned with the imprint that evolutionary history leaves on the diverse collection of current organisms and individuals. Biological physicists also made important
contributions to understanding the evolutionary process itself, particularly in recent years. There is a growing body of both theoretical and experimental work that aims to predict and measure, in quantitative detail, how populations create and maintain genetic variation, how efficiently natural selection can operate, and how predictable and repeatable adaptation will be. These advances are now contributing to understanding the somatic evolution of cancers, the operation of adaptive immune systems, the spread of viral epidemics, and more (Chapters 6 and 7).