8
Connected and Automated Vehicles
8.1 INTRODUCTION
The rapid growth in the application of electronic controls and information and communications technologies in automobiles goes beyond improving internal vehicle operations. Advances in sensing, control, and communication technologies will allow vehicles to respond to external information and to take increasing control of aspects of driving previously handled by the driver.
The primary motivation for the development of vehicle automation to date has been improved safety. In combination with connectivity, it can bring other benefits as well, such as convenience, equity and accessibility, productivity, and commerce/entertainment. If designed with efficiency in mind, automated vehicles could substantially improve fuel efficiency, lowering drivers’ fuel costs and increasing driving range for electric vehicles (EVs) while delivering societal benefits through reduced fuel use and emissions. Table 8.1 lists the benefits that may be realized from increasing automation and connectivity, the vehicle features that enable that benefit, and the primary beneficiaries. The term “autonomous” refers to those highly automated systems that can operate without
TABLE 8.1 Potential Benefits of Connected and Automated Vehicle Technologies
Effect | Automation and Connectivity Features That Enable the Benefit | Primary Beneficiary |
---|---|---|
Enhanced safety | Crash avoidance | Drivers, passengers, other road users |
Greater mobility | Autonomous driving | People unable to drive |
Convenience | Autonomous parking, automation of driving | Drivers, passengers |
Less stressful driving experience | Automation of driving tasks, optimized driving behavior | Drivers, passengers |
Higher fuel efficiency | Optimized driving behavior, powertrain operation, and routing | Drivers, passengers |
Reduced emissions per mile | Optimized driving behavior, powertrain operation, and routing | Society |
NOTE: The vehicle efficiency and emissions benefits that are the focus of this study are highlighted in grey.
a human driver (Society of Automotive Engineers [SAE] Levels 4 and 5; see Figure 8.2, below). Autonomous vehicles are the subject of Chapter 9.
This chapter is concerned with how connected and automated vehicle (CAV) technologies could affect the fuel efficiency of individual vehicles or groups of vehicles in close proximity. The impacts on energy use from fully autonomous vehicles, such as increased travel owing to accessibility for the non-driving population and greater productivity for vehicle occupants, are discussed in Chapter 9.
8.2 CAV TECHNOLOGIES
8.2.1 Automation Technologies and Operating Modes
The push for safer vehicles to reduce the number of road fatalities and injuries has been behind the introduction of electronically controlled systems to enhance human driving capabilities and supplement or replace mechanical- and hydraulic-based vehicular systems. These systems, known as advanced driver assistance systems, or ADAS, use sensors like radio detection and ranging (radar), light detection and ranging (lidar), cameras, sonar, global positioning system (GPS), digital maps, and actuators, along with complex software control systems to warn the driver of potentially dangerous behavior or conditions and assist the driver in case of imminent danger. Figure 8.1 shows some of these sensors and the safety features they are used for. These sensors, described in detail in Section 8.2.1.1, have overlapping capabilities and have their own strength and limitations.
ADAS systems provide the basis for future driving modes involving increased levels of automation up to and including the fully autonomous vehicle. SAE has defined various levels of automation in SAE international standard J3016 (SAE International, 2019). It identifies six distinct levels of driving automation from Level 0 to

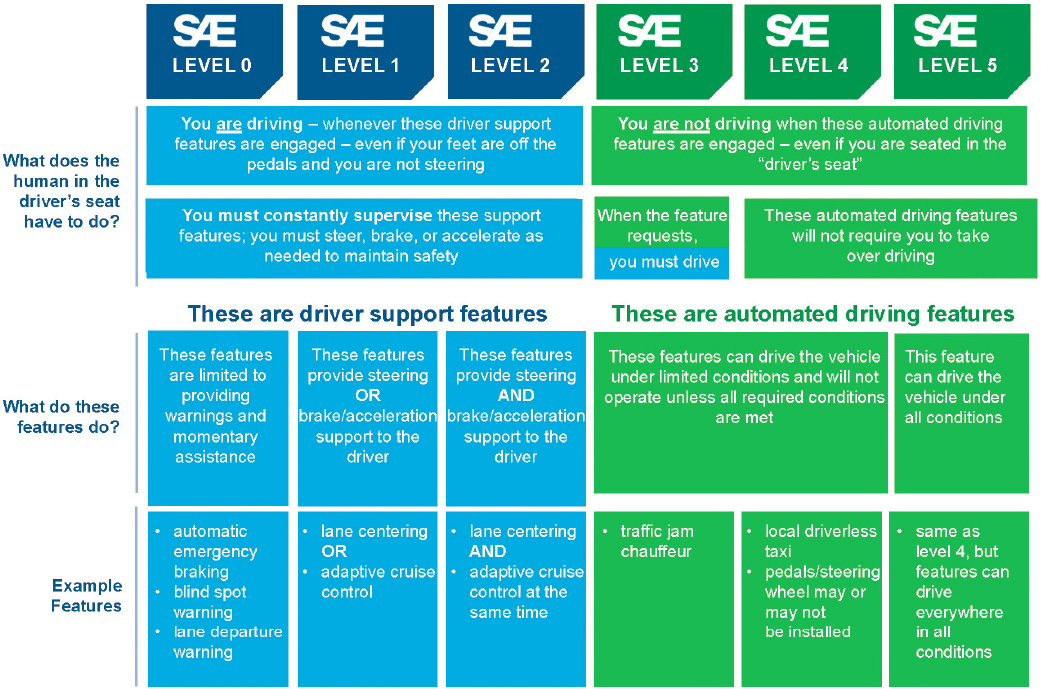
Level 5 and provides classifications and definitions of the functional aspects of the technology and the respective roles of the vehicle and driver in performing a dynamic driving task, as shown in Figure 8.2.
8.2.1.1 Sensor Technologies
Radar uses radio waves to detect the distance and velocity of objects in almost all environmental conditions. Radio waves emitted from the radar transmitter reflect off the object and return to the radar receiver, giving information about the object’s location and speed. They have been in use for automotive applications for many years and can be classified based on their operating ranges as follows: short-range radar (SRR) in the 0.2 to 30 m range; medium-range radar (MRR) in the 30 to 80 m range; and long-range radar (LRR) in the 80 m to more than 200 m range. For example, LRR is the key sensor for adaptive cruise control (ACC) and highway automatic emergency braking systems, while MRRs are mainly deployed for blind zone detection and rear cross traffic and SRRs for rear emergency breaking.
Lidar sensors measure distance to a target by illuminating the target with laser light and measuring the return time of the reflected light. Automotive lidar systems typically can provide up to 200 m range in restricted field-of-view (FOV). They cost much more than radar or camera sensors. More recently, solid-state lidar (SSL) technologies that have no moving parts and are therefore more reliable and lower cost are replacing mechanical scanning lidar, which physically rotates the laser and receiver assembly to collect data over 360 degrees. SSLs currently have lower FOV, but their low cost allows for using multiple sensors to expand coverage zone. They are also more compact and can be integrated with the vehicle body, reducing drag.
TABLE 8.2 Comparison of Sensors Used for Vehicle Automation, Strengths and Limitations
Ultrasonic | Camera | Radar | Lidar | |
---|---|---|---|---|
Cost | Low | Low | Medium | High |
Size | Small | Medium | Small–medium | Medium–large |
Speed detection | Low | Low | High | Medium |
Sensitivity to color | No | High | No | No |
Robust to weather | High | Low | High | Medium |
Robust to day and night | High | Low | High | High |
Dimensional resolution | Low (0.01 m) | High (1 Mp) | Medium (0.4, 0.4, 1.8 m) | High (horizontal 0.125 degrees, vertical 0.6 degrees) |
Range (m) | Short (8) | Medium–long (60–250) | Short, medium, long (20, 70, 250) | Long (150–200) |
SOURCE: Michigan Tech Research Institute (2017).
Cameras, in contrast, are passive systems, hence much simpler and lower cost. They are the only sensor technology capable of capturing high levels of detail such as texture, color, and contrast information, and therefore they represent the technology of choice for object classification. They play a key role in many of the ADAS systems in the market today, such as lane departure warning (LDW) and lane keeping systems. On the other hand, cameras are susceptible to adverse weather conditions and variations in lighting.
Ultrasound technology is used to detect objects in a range of a few centimeters to a few meters. It generates sound waves that bounce off objects and return to the car’s sensor, with a delay that is related to the objects’ distance from the vehicle. Ultrasound works in low-light conditions and poor weather, but it cannot classify objects; it only identifies their presence and distance. It also has relatively poor time resolution and is primarily used for slow speed conditions such as slow traffic or parking.
A side-by-side comparison of the four sensor technologies showing their strengths and limitations is given in Table 8.2.
To ensure safety in view of sensor limitations, sensor redundancies are necessary. The use of cameras in combination with radar and/or lidar in what is known as sensor fusion provides for a more reliable situational analysis of the environment around the vehicle. Since camera systems provide the most application coverage they are projected to see the largest volume growth close to 400 million sensor units by 2030. With radar and lidar costs coming down, they too are expected to see large percentage growth and volumes reaching 40–50 million sensor units by 2030 (Ors, 2017).
Last, keeping externally mounted sensors (cameras and lidar) clean is essential for reliable interpretation of the scene. To this end, several technologies for automating the cleaning process, ranging from liquid-jet wiper-based cleaning systems to ultrasonic-based, are at different stages of development (Brooke, 2020b; MS Foster, n.d.).
8.2.1.2 Data and Mapping Technology
In addition to onboard sensors, automakers are acquiring and using digital map, traffic, and other data to help their vehicles operate safely and efficiently. Such information can be used for routing and navigation, for predictive optimization of the powertrain operation, and for safe operation of the vehicle. High-definition (HD) digital mapping is an important addition to vehicle automation technology at all levels. While map accuracy for navigation purposes is 10 m and 1–2 m for ADAS purposes, HD maps have an accuracy of 10 cm. The market potential for using such maps particularly for Level 2 and 3 automation is substantial and provides the driving force behind this costly development (Markets and Markets, 2019). HD maps complement information from other localization sensors like GPS, lidar, and vehicle-to-infrastructure (V2I) communication and allow a vehicle to adjust to upcoming conditions in advance. It is important to note that HD maps do not require high accuracy GPS to provide the
cm-level localization. High accuracy GPS systems (Differential GPS-DGPS or Real-Time Kinematic-RTK) using a fixed ground station to provide error correction present other alternatives for providing cm-level localization but are considered more expensive and less accurate options.
Data for digital maps come from third-party sources, such as HERE, Google Maps, Waze, or TomTom, as well as from the fleet of vehicles produced by the automaker itself, if equipped with the ability to share data collected by the vehicles with the automaker. Data about routes, infrastructure, and traffic may be stored on board the vehicle or transmitted to the vehicle as needed. Vehicles’ use of HD maps is not expected to be power-intensive. While maps contain a huge amount of data, what is delivered to the client for use is a small subset of the total and requires limited onboard storage. Computation needs are also modest; predictive analytics requires less computing power than real-time processing of sensor data.
Developing these maps is both labor- and equipment-intensive, and hence costly. Mapping may cost hundreds of dollars per lane mile for data acquisition, and the data must be updated frequently. Moreover, this cost does not include map production, which requires the extraction of features with high accuracy. Costs are expected to decline over the next decade, however, as cars themselves increasingly contribute to the collection of data and artificial intelligence (AI) allows machine reading of lidar data, replacing manual work. Despite the high costs of creating HD maps, automakers thus far have worked independently to create or acquire these capabilities. There may be a role for a federal agency such as the Federal Highway Administration to oversee and/or host the data collection effort and perhaps develop a national specification. This could greatly improve the efficiency of map creation and ensure consistency across mapping products.
FINDING 8.1: High-definition maps are increasingly important elements of automation packages that complement information from sensors. By allowing the vehicle to anticipate and adjust to roadway features well in advance, they can improve safety and efficiency. However, they are expensive to produce and maintain and there has been little collaboration among automakers to establish common map specifications or to reduce their cost.
8.2.2 Connectivity Technologies
Vehicle connectivity is emerging as an important feature, alone or as a complement to automation, to promote greater safety through advanced knowledge of other vehicles’ sudden stopping and other potential hazards. It can bring multiple other benefits as well, including better fuel efficiency. Connected vehicles can use a combination of different technologies to communicate with their surroundings including other road users, roadside infrastructure, and other devices, which may be near or far.
8.2.2.1 Vehicle-to-Everything Connectivity
The capability for direct data exchange between a vehicle and other objects, called vehicle-to-everything or V2X connectivity, includes vehicle-to-vehicle (V2V) and V2I communications. V2V enables the exchange of information from any onboard device such as the brake system, steering, and so on that is operated by a controller area network (CAN bus) or other electronic system. Each vehicle broadcasts its location, heading, and speed in a secure and anonymous manner. For dedicated short-range communications (DSRC) this information must be transmitted 10 times per second in uncongested conditions (NHTSA, 2016). All surrounding vehicles, provided they are suitably equipped, receive the message, and each estimates the risk imposed by the transmitting vehicle. V2I allows equipped vehicles to share information with similarly equipped components that support a road system and operate on the same frequency, which may include traffic lights, lane markers, streetlights, signage, or parking meters, for example.
V2X communication can be viewed as a sensor capable of 360-degree coverage and of providing high-level data that require only simple decoding to process rather than complex signal processing techniques required of other sensors (V2X Core Technical Committee, 2020). V2X enables bidirectional information flow and operates well in poor weather and lighting. It also has a range of 300 m or more, which exceeds that of sensors in ADAS systems (Table 8.2).
TABLE 8.3 Per Vehicle Cost of Onboard DSRC Equipment Cost—Delphi Survey Average
2017 | 2022 | |
---|---|---|
Direct manufacturer cost of embedded DSRC | $175 | $75 |
Total cost (direct + indirect) of embedded DSRC | $350 | $300 |
Consumer cost of aftermarket DSRC | $200 | $75 |
SOURCE: CAR (2012).
V2X is especially useful for safety-related communications because it can support very low-latency, secure transmissions (DOT, 2020a). It also provides the connectivity for efficiency opportunities such as vehicle platooning. Signal phase and timing (SPaT) communications from traffic signals can be leveraged to generate safety (red light violation warning), mobility (traffic optimization, bus priority), and vehicle efficiency (acceleration optimization) benefits. V2X is not intended to provide the long range of cellular networks that is needed for other types of communication. V2X requires installation of roadside equipment, which could hinder implementation across the vast areas needed to allow vehicle communication in all parts of the United States.
Two wireless communication standards for V2X connectivity are DSRC and cellular-based communications (C-V2X), both for radio communications.1 The two systems are not interoperable, although both can be used on the same vehicle. In the United States, the Federal Communications Commission (FCC) allocated a dedicated spectrum of 75 MHz in the 5.9 GHz band for Intelligent Transportation Systems (ITS) specifically for DSRC technology (FCC, 2019a). While few vehicle models have been sold with V2X capability to date, at least 25 state transportation departments are already using this dedicated band for a variety of safety projects involving vehicles equipped with aftermarket connectivity devices. Projects include V2V and pedestrian collision avoidance, transit priority, and emergency vehicle traffic signal preemption (DOT, 2020b). In December 2019, the FCC issued a Notice of Proposed Rulemaking to propose that the lower 45 MHz of the band be repurposed for unlicensed uses including Wi-Fi and the upper 20 MHz of the band be dedicated to C-V2X, which currently has no spectrum allocation. The FCC also sought comment on whether the remaining 10 MHz should also be dedicated to C-V2X or be retained for DSRC (FCC, 2019b). Many automotive industry groups commented on the proposed rulemaking, noting that if frequencies were removed from the original 75 MHz band, ITS communications would face greater likelihood of congestion and disruption. The U.S. Department of Transportation expressed concern that this action would choose a winning technology (C-V2X) prematurely and would have adverse safety implications, and requested that the FCC pause its consideration of the proposal (DOT, 2020c). Nonetheless, on November 18, 2020, the FCC voted to adopt its proposal to release the lower 45 MHz of the band, retaining the upper 30 MHz for ITS uses utilizing the C-V2X protocol (FCC, 2020a).
DSRC equipment comprises onboard and roadside transceivers. A 2012 report of the Center for Automotive Research reported DSRC per vehicle cost projections from a connected vehicle technology industry Delphi study, as shown in Table 8.3 (CAR, 2012).
C-V2X, now under development, offers an alternative to DSRC for direct communication between vehicles and other road users, vehicles, and infrastructure. While C-V2X equipment is currently somewhat more expensive (ABI Research, 2018), proponents claim a cost advantage for C-V2X over DSRC based on private sector expertise and integration of road-side units with the cellular network (Qualcom, 2017).
General Motors (GM) is the only automaker to have equipped any vehicles in the United States with DSRC, and deployment was limited to CTS sedans, which were discontinued following model year (MY) 2019 (Abuelsamid, 2019; Lopez, 2019). Similarly, Europe initially planned to adopt DSRC but is now moving toward C-V2X. China adopted C-V2X at the outset and is now the world leader in testing and implementation of the technology. Multiple pilots are now under way. Ford has announced plans to launch C-V2X in China by 2021 and in all vehicles sold in the United States starting in 2022 (News Wheel, 2019).
___________________
1 Here “cellular” refers not to the use of cellular networks but to the electronics used in cellular radios.
8.2.2.2 Cellular Network Connectivity
While direct, short-range connectivity is essential for safety-critical communications, connectivity through cellular networks is used for a rapidly expanding universe of other vehicle capabilities. Most new vehicles today offer internet connectivity for infotainment and in-vehicle apps. They also include telematics, which provides detailed in-use vehicle operating data to automakers.
Connectivity through cellular networks can also add to the safety and efficiency benefits of short-range V2X connectivity by providing wide-area road condition information, real-time map updates, and routing alternatives. When combined with C-V2X short-range connectivity, it can produce cost savings as well as new business opportunities in the realm of ecommerce. Such activity could help manufacturers fund the development of advanced sharing programs, which is key for automakers and others entering the shared mobility space.
Until very recently, cellular devices in the United States used 4G-LTE (Long-Term Evolution), the fourth-generation standard for wireless cellular communications. 4G-LTE was designed to provide up to 10 times the speeds of 3G networks for mobile devices using the existing cellular infrastructure. LTE is the most modern standard that is readily available in most areas of the United States. The latency of LTE is variable depending on many factors that can decrease speed of communication on the network. Signal interference from obstructions can make usage in cities unpredictable.
5G is the fifth generation of cellular mobile communications. It succeeds the 4G, 3G, and 2G systems. 5G performance targets high data rate, reduced latency, energy saving, cost reduction, higher system capacity, and massive device connectivity. Major carriers have now reached nationwide 5G coverage in the United States. 5G makes possible peer-to-peer communications through the network using mobile “edge computing,” providing the necessary improvements in bandwidth and latency as well as the ability to separate safety-critical and non-safety critical messages (ABI Research, 2018; Qualcomm, 2019). Additional capabilities for connected vehicles will include the ability to share sensor data and planned trajectory and to build local HD maps in real time (Green Car Congress, 2020). However, to achieve such benefits 5G requires many more towers than its predecessors. A recent study forecasts 41 million 5G C-V2X vehicles globally by 2030 and 83 million by 2035 (Green Car Congress, 2020).
8.2.3 Specifications of CAV System Components
Table 8.4 shows representative specifications for current (Level 2) automated vehicle system components.
TABLE 8.4 Onboard Technologies That Enable Automation and Connectivity and Their Capabilities as Implemented in 2020 Vehicles
Technology | Specs in 2020 | |||
---|---|---|---|---|
Number per Vehicle | Weight per Unit (kg) | Power Draw per Unit (W) | Cost per Unit (US$) | |
Stereo camera | 7–11 | 0.06 | 1–2 | 45 |
Lidara | 0–2 | 0.83–12.25 (small–large) | 8–60 | 2,000 |
Radar | 1–5 | 0.25 | 4–18 | 55 |
Ultrasonic sensor | 0–12 | 0.04 | 0.1 | 10 |
DSRC onboard unit | 1 | 2.7 | 6 | 75 |
C-V2X radio/chipset | 1 | 90 | ||
Inertial measurement unit w/GNSS b | 1 | 1–7 | ||
Computer | 1–2 | 3.13–5.075 | 80–96 | 800 |
a Lidar not typically included in Level 2 systems but shown here for completeness.
b Global Navigation Satellite System.
SOURCE: Gawron et al. (2018); Baxter et al. (2018); CAR (2012); ABI Research (2018); committee estimates.
Cost and power draw of these individual components are generally decreasing. Automative lidar costs in particular are projected to fall rapidly, from about $2,000 in 2020 to hundreds of dollars within a period of 3 to 5 years. Some lidar suppliers have already commercialized units at well under $1,000, although with limited range and resolution. For example, Valeo’s SCALA is available at high volumes at a cost of $600 (Rangwala, 2020). Velodyne, which demonstrated a compact, solid-state $100 lidar for applications such as drones and robots at CES 2020, has announced that its solid-state, automotive grade Velarray H800 has a target price of less than $500 at high-volume production (Krok, 2020; Velodyne Lidar, 2020). At the same time, as automation advances, the number and sophistication of devices will increase. Tesla’s Autopilot V3 computer uses chips capable of 36 trillion operations per second (Abuelsamid, 2019); by comparison, chipmaker Nvidia reports that its next-generation chip will be able to process 200 trillion operations per second. The trajectories of total cost and power consumption for automation systems are not yet clear, and are discussed further in Section 8.3.8.1.
In addition to these onboard technologies, CAVs rely to varying degrees upon cloud computing, traffic and map data, infrastructure sensors, and security systems. These offboard systems will be costly and will consume considerable amounts of energy, although on a per-vehicle basis requirements may be low in the future if most vehicles on the road are using their services. For both on- and offboard systems, sophisticated algorithms, models, and data acquisition are required, at high cost to automakers and suppliers.
8.2.4 Onboard Computing
Vehicle automation systems require substantial onboard computing. The continued expansion of their functionality to systems with greater vehicle driving authority requires dramatic increases in electronic content and interconnected, embedded systems. These are complex systems, in contrast to the relatively simple, stand-alone computing systems that once controlled basic engine and vehicle functions. These systems are also evolving to become highly cyber-physical in nature, and perhaps represent some of the most sophisticated, widely distributed cyber-physical systems that exist today. These are characterized by the following:
- Deep physical interactions (sensors, actuators, controllers);
- Deeply embedded electronics (nonlinear multilayered interactions);
- High degrees of computation (hundreds of trillions of operations per second);
- Rich needs to communicate (high speed, rich data communication is a must for time critical decisions);
- Pervasive integration (cyber and physical); and
- High degree of coupling with driver behavior.
Because of these systems’ complexity and their need for tight control of multiple interconnected, embedded systems with high levels of security, accuracy, and reliability, there are many challenges facing the designer and developer of such systems. For example, components and subsystems can no longer be designed and developed in isolation and then integrated into the vehicle. Now, complete systems have to be integrated at the outset of the design process and in setting system requirements, in order to allow mutual interactions at deeper and deeper levels. Also, efforts are ongoing to centralize and integrate some control functions in order to optimize the number of the electronic control units wiring and connectors involved, reducing cost. In addition to the high cost and large power demand—more than 2 kilowatts (kW) reported for prototypes—for intensive computing, this complex cyber-physical system requires new computing hardware and software architectures and new system engineering and design tools to be integrated into the vehicle. Examples include the following:
- The integration of sophisticated control algorithms involving a large number of software code lines makes it increasingly difficult to verify and validate these algorithms and their interaction under a plurality of inputs and conditions (states) using conventional trial and error approaches. The use of emerging, systematic, and automated “formal methods” techniques for requirements checking of model and software generations are becoming essential for the design of reliable software. Furthermore, the integration of algorithms developed by multiple sources requires strict adherence to software architecture standards such as AUTOSAR.
- Advanced control strategies and architectures are used to ensure “fail-soft” and “fail-operational” requirements, needed for Level 2–Level 5 automated driving.
- Information security is critical with the increasing usage of wireless communications involving data transfer, which also interacts with vehicle control devices (throttle, steering, and braking). Therefore, a strong emphasis on automotive cybersecurity is key to the success of advanced safety critical systems.
- To achieve the control accuracy and reliability required for advanced active safety and autonomous driving systems there is an increasing need for fast, reliable, onboard communications, and computational approaches at affordable cost. These typically involve modern field programmable gate array capable of parallel processing high volumes of data on a single chip utilizing sophisticated error correction algorithms.
- Diagnostics and prognostics of cyber-physical systems present a challenge owing to their complexity, but at the same time they are considered key enablers for systems service and repair and customer peace of mind.
The sophisticated technologies addressing the above challenges have already found their way into the design and development process of automakers and suppliers as a means of ensuring the safety and integrity of these new complex products. Furthermore, the large electric power demanded by onboard computing experienced by prototype autonomous vehicles, as well as its cost, is expected to greatly diminish by the time autonomous vehicles are more widely available (2030) owing to intensive efforts by chip manufacturers (Stewart, 2018).
8.2.5 CAV Systems in Production
Several vehicle models with Level 2 CAV systems are in production and many more, as well as some Level 3 systems, are in the preproduction phase or in planning in the United States and other locations. Table 8.5 details several of the available and upcoming models.
8.3 IMPACTS OF CAV TECHNOLOGIES ON VEHICLE EFFICIENCY
Vehicle automation can increase or decrease fuel consumption owing to a variety of factors. Even at low levels (Level 1 to Level 2), automated driving can adjust vehicle operation (speed, acceleration, deceleration) to achieve an eco-driving mode. Existing electronic vehicle controls will then minimize unnecessary or unexpected braking and high acceleration (unless there is a safety need), reducing high-torque and fuel-inefficient maneuvers.
Even during human driving (Level 0), the integration of short-term (5–20 second) load preview (prediction of upcoming need for acceleration and deceleration) using onboard sensing and long-term (1–20 minute) forecast of driving patterns (route estimated time of arrival, traffic ahead, signal lights, signs, intersection congestion, accidents, etc.), through historic data or rough real-time traffic information available from popular navigation tools, will provide opportunities for further optimizing powertrain efficiency. As will be discussed below, anticipating the load demand offers unique opportunities to improve powertrain efficiency while satisfying these load demands by calibrations that judiciously manage torque (power) and energy reserves.
Connectivity can complement automation to help a vehicle run more efficiently. Automation does not require connectivity, as the car can replicate the driver via AI and algorithms. However, if an algorithm is short sighted in optimizing (short horizon), has poor perception (noisy or delayed signals), or causes aggressivity (short time headway), it may increase fuel consumption (Zhu et al., 2019; Prakash et al., 2016). The full benefits of vehicle automation can be achieved only with connectivity and there are many benefits that connectivity can provide sooner, and perhaps at lower cost, than can be provided through automation. Particularly in the early years, using both automation algorithms and connectivity will allow for the best decisions by the computer control module. Optimization of powertrain operation for efficiency will be enhanced by information on upcoming activity from other vehicles, traffic lights, and other infrastructure. The ability to engage in more co-operative driving scenarios or to form tightly controlled clusters of vehicles as in platooning requires connectivity and automation features.
TABLE 8.5 Example Automated Vehicles Deployed, Including Their Level of Automation, Technology, Automation Features, and Details on the Year Introduced and the Technology Penetration
Make and Model | Level of Automation | Technology | Automation Features | Year Introduced (MSRP + Additional Cost of Automated Tech) | Technology Penetration |
---|---|---|---|---|---|
Mercedes-Benza | 2 (Driver Assistance Package) |
|
|
Introduced 2013 (updated 2016, 2017) (+$2,250) | Available on most Mercedes Benz models |
Nissan ProPilot Assist Altima, Rogue, Leaf | 2 (Pro Pilot Assist with Navi-link) |
|
|
MY 2016 (Serena, Japan) ($22,000–$32,000) | High for both luxury and economy models (Japan) |
MY 2018 (Rogue, United States) ($25,000–$33,000 + $0–$2,500) | Luxury models in the United States | ||||
Cadillac CT6 | 2 (Super Cruise) |
|
|
MY 2018 ($75,000 + $2,500)b | CT6 discontinued in 2020 |
Audi e-tron | 2 |
|
|
MY 2019 ($74,000 + $3,500) |
Make and Model | Level of Automation | Technology | Automation Features | Year Introduced (MSRP + Additional Cost of Automated Tech) | Technology Penetration |
---|---|---|---|---|---|
BMW X5 with Extended Traffic Jam Assistantb | 2 (Extended Traffic Jam Assistant) |
|
|
MY 2019 ($59,000–$82,000 + $0–$1,700) |
Debuted on 2019 X5. Available as standard or optional on these 2020 models:
|
Hyundai Ioniq, Sonata, Nexo, Palisade | 2 (SmartSense with Highway Driving Assist) |
|
|
MY 2019 ($23,000–$60,000 + $0–$3,100) | Standard in limited trims, available in add-on packages for lower-tier trims |
Tesla (Model S, 3, X) | 2 |
|
|
MY 2019 Model S ($89,200) | Available worldwide |
Model 3 ($35,000) | |||||
Model X ($81,000) | |||||
Lincoln Corsair, Aviator, Nautilus | 2 (Lincoln CoPilot360 Plus) |
|
|
MY 2020 ($42,000–$55,000 + $4,150–$4,950) | Available as an add-on in luxury trims |
Toyota Mobility Teammate | 2 (Mobility Teammate) |
|
|
MY 2020 (not for sale) | LS1 Concept in Japan |
Cadillac CT5, CT4, Escalade | 2+ (Enhanced Super Cruise) |
|
|
MY 2021 ($40,000–$75,000 + $2,500–$6,150)c | MY 2021 triples number of models offering Super Cruise, along with additional capabilities |
Nissan ProPilot 2.0 Ariya | 3 (Pro Pilot 2.0) |
|
|
MY 2020 (Skyline, Japan, $40,000) | Standard with hybrid Skyline in Japan |
Will be available in United States with MY 2021 | |||||
BMW 2021 iNext | 3 |
|
|
2021 (highway pilot) | |
Mercedes-Benzd | 3 (Drive Pilot) 4 (Intelligent Parking Pilot) |
|
|
Late 2021 (Germany only) | |
Cruise Origine (GM-Honda) | 4 |
|
|
2022 (not for sale) | Purpose-built for “automated taxi” and mobility as a service pilot deployments, beginning in San Francisco |
Argo AI (Ford-Volkswagen)f | 4 |
|
2022 (not for sale) | Commercial service expected in 2022, test Fusion Hybrids and Escape Hybrids in six U.S. cities |
NOTE: AVM = around-view monitor; MSRP = manufacturer’s suggested retail price. MSRP and technology package costs sourced from automaker websites and online catalog.
a Mercedes-Benz USA (2017); Autotrader (2020).
c But must already have Driver Assist and Technology Package, $3,650. Patel (2020); Cadillac Pressroom (2020).
f Korosec (2020); Wayland (2020); Ford Motor Company (2020).
CAV technologies may also bring indirect energy savings through system effects. For example, safety improvements from automated emergency braking or lane-keeping assist can save fuel by reducing the congestion associated with vehicle crashes. On the other hand, automation technologies can increase vehicle miles traveled: in a 2018 survey conducted by University of California, Davis researchers, Tesla buyers reported large increases in weekend travel and travel under congested conditions owing to availability of the Autopilot system (Hardman et al., 2019). Fully autonomous vehicles could further increase or decrease fuel consumption overall by changing vehicle miles traveled and vehicle size and weight, as discussed in Chapter 9. This section focuses on automation technologies’ direct impacts on individual vehicles’ fuel efficiency. They may vary substantially across implementations of a given technology and can depend strongly on driving conditions and adoption levels. Understanding of these factors is improving as a result of modeling and real-world experience, however.
8.3.1 Velocity Optimization
Using automation to optimize vehicle speed can produce substantial fuel savings. For most powertrains, minimization of tractive energy to transport a vehicle from a point A to point B corresponds to a “pulse and glide” operation which involves three phases—namely, short but high accelerations to a cruising speed, followed by a fixed cruising speed, and finalized by short, sharp braking. The resulting change in acceleration, also known as jerk, associated with this energy-optimum pattern increases passenger discomfort and is limited by the automakers. Instead, they aim to deliver smooth velocity transitions and minimization of accelerations that exemplifies eco-driving style.
ACC provides an opportunity for velocity optimization. ACC was primarily designed for convenience (cruising) and safety (maintaining a safe headway from proceeding vehicles) and not for fuel efficiency. The ACC functionality involves low-level automation and could be adjusted with a short preview for fuel efficiency creating the commonly known eco-ACC functionality. The optimal velocity trajectory maintains a distance from the vehicle ahead that minimizes acceleration events and aerodynamic drag and is short enough to prevent cut-ins from other lanes while ensuring a safe distance from the lead vehicle. Prediction of the velocity of the vehicle in front of the subject vehicle is necessary since optimal decisions depend on future acceleration demands. When the subject vehicle is on an open road, the eco-driving approach may use the speed limit for that road segment based on existing navigation tools.
To assess the benefit of these optimal following velocity profiles in a laboratory setting, a lead vehicle is typically represented by a federal testing drive cycle. Implementing the optimization in the laboratory on an Escape 1.6 L Ecoboost engine with full drive cycle preview showed up to a 17% increase in fuel efficiency (Prakash et al., 2016). Real-time implementation with model predictive control achieves improvements in fuel efficiency proportional to the prediction horizon. When the prediction horizon reaches 20 seconds, most of the fuel efficiency benefits of full preview are achieved. A preview of less than 5 seconds, however, may eliminate most of the benefits and can even make the automated driving efficiency worse than that of a human-driven vehicle unless special robustification measures are applied. With those more robust algorithms, some increase in fuel efficiency was obtained with as little as 1.5 seconds’ preview of the lead vehicle velocity (Hellstrom et al., 2018).
8.3.2 Added Benefit of Connectivity
In a vehicle without connectivity, eco-ACC strategies assume a constant acceleration or constant velocity up to a predefined prediction horizon (Guanetti et al., 2018). These methods result in large errors in expected velocity at the prediction horizon that can degrade the eco-ACC performance (He and Orosz, 2017; Vajedi and Azad, 2016). Connectivity enables a preview of the trajectory of a vehicle in front of the subject vehicle or the distance to a stop sign or an upcoming traffic light. Traffic signal information would be available through transmission of SPaT messages.
V2V communication can dramatically improve the accuracy at the prediction horizon used for fuel efficiency optimization, as shown in Figure 8.3 (Hyeon et al., 2019).
If an automated vehicle using eco-driving algorithms is tested for fuel economy by “following” a federal testing drive cycle, it will exceed the allowable deviation from the standard drive cycles for fuel consumption testing.

Hence the automated vehicle cannot use eco-driving during testing, so the fuel savings go undetected, removing an incentive for automakers to adopt the technology.
FINDING 8.2: Connectivity technologies can add substantially to the fuel savings benefits of automation technologies.
8.3.3 Efficiency in Internal Combustion Engine-Based Powertrains
The preceding sections discuss how automation can be used to optimize a vehicle’s velocity profile to save fuel. Connectivity that enables the prediction of upcoming acceleration or braking (load preview) will also enable the full realization of the benefits of many powertrain technologies whose calibration involves significant tradeoffs between efficiency and drivability by reserving torque for unexpected accelerations. These two efficiency opportunities in co-optimizing velocity profiles and powertrain control enabled by connectivity and automation can achieve benefits that are nearly additive.
Forseeing the need for acceleration or braking will enable currently automated powertrain technologies such as high dilution, advanced ignition, and turbocharging to be calibrated in their most efficient value without drivability compromises. Nazari et al. (2017) showed a 4% reduction in fuel consumption from high exhaust gas recirculation on the US06 drive cycle with full load preview compared to a strategy that reserves boost pressure for fast transients. This significant fuel efficiency gain can be attained with low-level (Level 1 to Level 2) automated driving that plans load transitions; much of the benefit can be achieved with even 1-second load preview. The benefit is much smaller (0.6% reduction in fuel consumption) if there is load anticipation of only 300 milliseconds, however
(Hellstrom et al., 2018). The loss in fuel savings is indicative of the sensitivity of these benefits to the extent of unanticipated load transients.
Connected and automated driving can also attain the full benefit of engine and powertrain technologies with discrete operating modes such as cylinder deactivation and gear switching (Olin et al., 2019; Sciarretta and Vahidi, 2020). Automation can provide benefits in two ways: (1) by keeping operation as much as possible inside the most efficient mode and (2) by minimizing the number of switches and hence reducing the switching losses and wear. The mode-switching process is in general inefficient, and having a preview of upcoming load allows the powertrain controller to decide if it is worth switching based on how long the load will stay within a given mode and how long it takes to recover the switching losses.
While anticipating the load can benefit high efficiency powertrains, automakers do not currently take advantage of this opportunity for added efficiency because the acceleration/deceleration anticipation will not be accurate in traffic with low penetration of automated and/or connected vehicles. Such calibrations can be applied if the vehicle recognizes that it is driving in a lane or an environment with low uncertainty, as in the case of certification testing. However, such adjustments in calibration based on drive cycle would need to be well documented to declare and explain their legitimate need and benefits to regulators. Some automakers recently applied cycle-dependent adjustments to improve emissions test results that did not carry over to real-world reductions and accordingly incurred “cycle-beating” fines. Being unable to claim the benefits in certification testing, automakers may find that adjusting calibration using load anticipation to improve fuel efficiency is not worthwhile, at least until automated vehicles are more prevalent in the fleet.
Furthermore, while connectivity and automation permit optimal operation of many powertrain efficiency technologies, high penetration of CAVs would lessen the need for complex technologies such as engines with turbocharging, lean combustion, or dynamic cylinder deactivation, or high-gear number transmissions. That is because when traffic is largely comprised of automated vehicles, eco-driving (minimization of acceleration) will be prevalent, greatly reducing the need for high torque. Right-sized, therefore small, naturally aspirated engines will be adequate. While downsized engines and overall powertrains will have poor drivability and fuel efficiency when they are used in abrupt or uncertain traffic, the deterministic traffic associated with a high penetration of automated driving would enable aggressive powertrain downsizing for vehicles not requiring towing or load-carrying capability.
At least in the near term, automakers will be reluctant to design general purpose light-duty vehicles that can function well only under automated driving conditions. This creates a near-term barrier to their taking advantage of this opportunity to employ low-cost, fuel-efficient, downsized powertrains in CAVs. Dedicated vehicles for use in enclosed and low speed areas, such as the shuttles being used in various closed-campus services, are good candidates for such aggressive downsizing, however.
FINDING 8.3: Connected and automated driving can allow some engine and powertrain efficiency technologies to achieve their full savings potential. However, high penetration of highly automated vehicles that create a deterministic traffic environment would limit the need for high torque capability and thus make small, naturally aspirated engines with stop-start and limited torque-assist the most cost-effective and efficient option for engine-powered vehicle powertrains where towing and load-carrying capabilities are not required. At least in the near term, however, automakers will be reluctant to design general purpose, light-duty vehicles that can function well only under automated driving conditions.
8.3.4 Power Management of Hybrid Powertrains
CAV technologies offer additional efficiency opportunities in hybrid electric vehicles (HEVs) by optimizing their power management through engine load leveling and regenerative braking when the battery can accept the energy. Optimal decisions on how to divide the power delivered between an engine and a battery depend on continuous forecasting of future efficiency and opportunities to replenish the energy used from the battery. Automation can improve HEV fuel efficiency by providing a planned load profile (removing uncertainty from the optimization horizon) or by co-optimizing power management and the vehicle velocity profile in an eco-driving scenario.
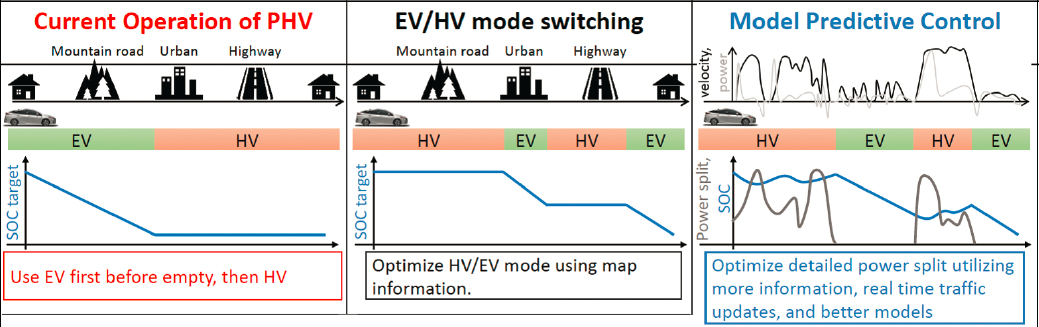
NOTE: HV = hybrid vehicle; PHV = plug-in hybrid vehicle; SOC = state of charge.
SOURCE: Butts for Huang et al. (2019).
For example, an algorithm can determine that the battery will be able to provide the demanded power with the engine off for a longer period if the system anticipates or can induce a deceleration command later to recharge the battery.
These efficiency gains (2%–3%) from power management in a strong HEV by automated driving or load preview can be smaller than the efficiency gains obtained in mild or micro-HEVs (4%–6%) for two reasons (Karbowski et al., 2020; Nazari et al., 2019). First, efficiency gains from automation can be larger in powertrains having lower baseline efficiency. Second, automation can effectively manage over-constrained systems such as micro-HEVs, where torque assist and regenerative braking are limited by the small battery size.
In plug-in HEVs (PHEVs), optimizing the charge depletion and power management depends on the vehicle speed and accelerations associated with urban or highway traffic, as shown in Figure 8.4. Generally, a strategy of charge depleting mode followed by charge sustaining mode (CDCS) is not optimal when the required trip energy is greater than the energy stored in a battery. Huang et al. (2019) find 3%–7% fuel efficiency benefits from real-time optimization of power management, relative to the CDCS strategy. The optimal battery discharge trajectory depends on the traffic or speed profile of the entire trip. Some traffic information can be obtained from historical travel data through onboard GPS and mobile navigation applications (Wang et al., 2019). Real-time information through V2X can also be integrated, but Huang et al. (2019) showed that using historical data to optimize power management can also be effective.
Through decades of development, the energy management and optimization algorithms for HEVs and PHEVs have become a mature field. Nonetheless, the computational burden of this optimization problem of planning a long trip without intermediate charging availability (for PHEVs) is still evolving from the first few sources (Paganelli et al., 2002; Guzzella and Sciarretta, 2013) with different algorithms thoroughly reviewed for PHEVs (Martinez et al., 2018) and HEVs (Sciarretta and Vahidi, 2020). Handling the engine on-off switching systematically by penalizing the cranking fuel loss provides 1%–2% fuel efficiency benefits. Additional benefits of up to 6% can be derived by co-optimizing the power management and the velocity profile leading to so-called pulse-and-glide behavior (Chen et al., 2018). This introduces passenger discomfort, however, and hence will be more appropriate for unoccupied vehicles used for delivery purposes.
Realizing the full potential of real-time engine, gear, and power management relies on accurate load prediction. Prediction to a short (1 second) horizon is extremely important for choosing correct gear values, boosting level, and engine on-off decisions, whereas a medium horizon (5 seconds) load preview is needed to avoid chattering (switching among optimum values). Longer time horizons and communication from signalized intersections improve the fuel efficiency of conventional or hybrid powertrains (Figure 8.5; Pozzi et al., 2020).
However, uncertainties at some time ahead (similar to adding an unplanned trip to a grocery store on your way home from work) will need to be accommodated in a PHEV (or battery electric vehicle [BEV]) where there
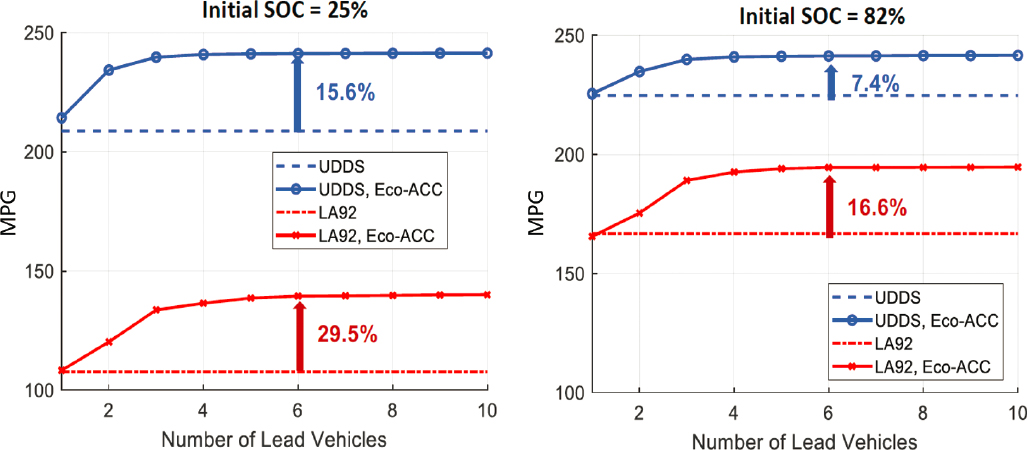
SOURCE: Team Presentation by SWRI at NEXTCAR ARPA-E 2019 Annual Meeting.
is a desired final state like a depleted battery. Automated driving of a PHEV or BEV is most efficient with a long prediction horizon, which connectivity can provide, to accommodate slow dynamics (battery state and thermal) (Lee et al., 2020). Optimization accounting for a long horizon creates a large computational load, however, which in turn draws power and reduces the benefits an EV may experience from automated driving.
In conclusion, PHEVs have the potential to achieve the highest percentage increase in energy efficiency from automation. Automated driving of PHEVs that combines optimal eco-driving and power management incorporates and even enhances all the above benefits and can achieve as much as 14% higher fuel efficiency on trips that significantly exceed the battery range (Chen et al., 2018). The efficiency gain is drive-cycle-dependent; over a combination of repeated highway cycles (similar to US06 and Highway Fuel Economy Test [HWFET]) followed by cycles that represent congested urban driving (similar to the EPA Urban Dynamometer Driving Schedule [UDDS]), fuel efficiency gains of more than 14% over a simple CDCS operation strategy are achievable (Chen et al., 2020). These benefits are not currently detected in the certification testing, however, so customer satisfaction is the sole incentive to the manufacturer to implement these optimization strategies.
FINDING 8.4: The look-ahead information provided by connected and automated vehicle technologies can be used to improve power management in hybrids, increasing efficiency by 2%–3% in strong hybrids and 4%–6% in mild hybrids. PHEVs have the potential for the highest percentage gains, with as much as 14% higher fuel efficiency on trips that significantly exceed the battery range.
8.3.5 Interactions with Electrification
The interactions between automation and connectivity technologies and electrification are diverse. Energy efficiency benefits of CAV technologies are smaller for BEVs than for other vehicles because BEV powertrains
are highly efficient and not subject to many of the energy losses that CAVs can mitigate in other vehicle types through strategies such as eco-driving. In addition, BEVs stand to lose range owing to CAV system power draw, at least until those loads have been greatly reduced. Indeed, given that BEVs will ultimately be equipped with CAV technologies to improve safety, functionality, and convenience, one might view the primary energy efficiency objective for a BEV’s CAV technologies as offsetting any range loss arising from the system’s power requirements.
On the other hand, BEVs’ electrical and auxiliary systems may otherwise be a good match for CAV packaging requirements. Eco-routing, which relies heavily on real-time traffic information, could be highly beneficial to BEV efficiency in hilly terrains, although it should be noted that travel time may increase as a result. Weather forecasting integrated with traffic could lower cabin and battery thermal loads by 2%–4% (Sun et al., 2019) and thus reduce the cold-weather impact on BEV range. Connectivity could allow BEVs to seamlessly choose routes and operating modes tailored to individual vehicles to maximize range and extend battery life (Taiebat et al., 2018). Fully networked charging infrastructure will optimize routes and advise drivers or the autonomous vehicle of available charging stations. Connectivity could also enable planning the demand response and various functionalities such as V2G to support the grid or V2B to support buildings (peak management, or renewable energy storage), which reduces offboard fuel consumption and emissions. It could also help to address range anxiety by providing accurate, route-dependent information on battery state of charge and charging options.
In developing more advanced CAV systems, and in particular for fully autonomous vehicles, automakers vary in their powertrain approaches. For example, Ford is using a hybrid platform for its first autonomous vehicle prototypes, citing range loss and battery degradation of autonomous BEVs (Iaconangelo, 2020). However, other automakers including GM, as well as autonomous vehicle startups and ride hailing companies, are moving directly to a BEV platform for autonomous vehicles (Mohan et al., 2020). Indeed, full autonomy can complement and facilitate vehicle electrification in additional ways, as discussed in Chapter 9.
FINDING 8.5: Compared to other powertrains, all-electric powertrains will see the lowest efficiency gain from connected and automated vehicle (CAV) technologies because they are already the most efficient. However, electric vehicles will benefit from other synergies with CAV technologies.
8.3.6 Effects by Powertrain and Drive Cycle
Karbowski et al. (2020) used vehicle simulation to quantify the effects of many of the CAV-enabled optimization strategies discussed above for conventional, hybrid, and battery electric vehicles. Figure 8.6 shows the percent energy savings they found from eco-driving (velocity control) with and without powertrain controls, and with and without connectivity (V2I), on multiple drive cycles. On the highway cycle, all vehicle types and control strategies achieved modest benefits of 4%–5% except for a small added gain for hybrids from powertrain control. As expected, urban cycle benefits were much larger, with biggest gains for conventional and hybrid vehicles employing speed and powertrain optimization as well as V2I communication (traffic SPaT). Velocity optimization alone showed savings of more than 10% on a suburban cycle for some powertrain types.
The results in Figure 8.6 may differ from real-world and test results for various reasons: traffic is not considered; city and highway cycles do not coincide with standard test cycles and are not statistically representative of U.S. driving; imperfections in calibration and future horizon knowledge; and unaddressed trade-offs among energy consumption, drivability, and travel time (Karbowski et al., 2020). Nonetheless, the results are instructive in that they provide a consistent basis for comparing savings across vehicle types and CAV capabilities.
8.3.7 Congestion Avoidance and Reduction
A connected vehicle has the potential to avoid or mitigate congestion and thereby reduce energy consumption. It can modify routes to avoid congested areas based on traffic flow data from the cloud. Connected vehicles may also reduce congestion by using data to drive more smoothly, stop less often, and optimize speed. This can improve the fuel efficiency not only of the connected vehicle but of following vehicles as well (Karbowski et al., 2020).
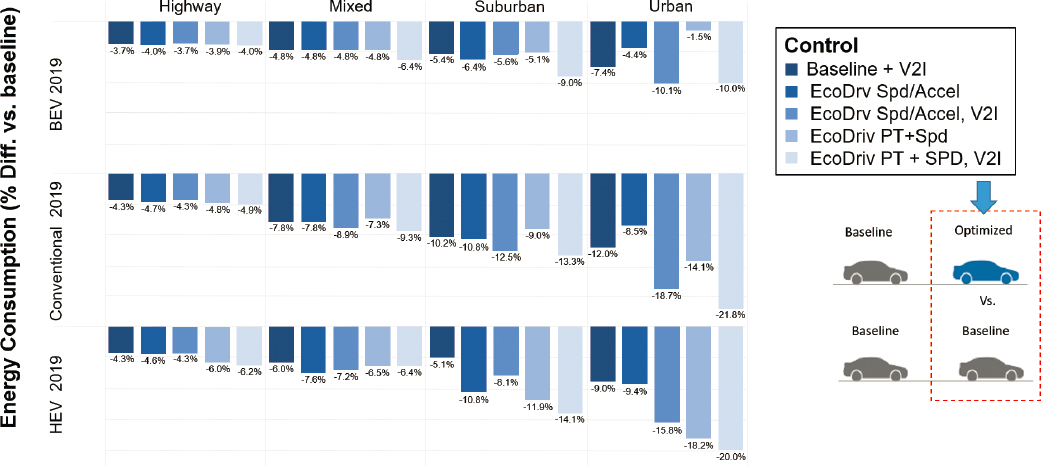
SOURCE: Karbowski et al. (2020).
Congestion reduction strategies such as traffic flow metering and traffic light management are more effective if the car can communicate with the traffic light and respond appropriately. Last, connectivity and automation can reduce the number of crashes, eliminating the congestion owing to those incidents.
8.3.8 Vehicle Load Issues for CAVs
8.3.8.1 Electric Equipment Power Demand
The electric power demanded by the computers, sensors, and actuators used in vehicle automation can be considerable. This power must be generated by a larger engine-driven alternator, resulting in an increase in fuel consumption. Table 8.4 shows approximate per-unit power draw of CAV components; the numbers and combinations of the sensor types will vary by systems at each level of automation. Total power needs for sensors are estimated to be 200–250 watts (W) for a system of Level 3–4 (Baxter et al., 2018; Gawron et al., 2018).
However, moving beyond Level 3 requires substantially more computing power owing to the variety of circumstances the systems must respond to and the ability to respond reliably without human back-up. This is likely to increase power demand by an order of magnitude, resulting in a load of up to a few kilowatts (Baxter et al., 2018). This load would far exceed that of the car’s heating, ventilation, and air conditioning system, for example, and could substantially reduce fuel efficiency and, for EVs, range as well.
Hamza et al. (2019) simulated Levels 4 and 5 automated driving systems for real-world vehicle trips from the California Household Travel Survey assuming constant system power consumption of 2.5 kW above that of a non-automated vehicle, consistent with “the likely present-day value” (Hamza et al., 2019). They found that such a load would reduce efficiency on these real-world trips by up to 35% and lower range for EVs by 23%–27%. However, targets for system power requirements in the future are several times lower (0.5 kW) (Hamza et al., 2019).
Most compact electronic equipment in the range of 500 W and above needs cooling which increases the weight, volume, and power draw. Novel cooling systems with optimized secondary-loop for heat pipes, heat pumps, and other thermal management systems are being explored intensively, primarily driven by the reduced range that such accessory systems can cause to BEVs.
The equipment and data processing required to support CAV systems will also consume energy in servers processing the data streams to and from vehicles, roadway infrastructure, and the cloud, creating large new electricity loads in the aggregate. Such electricity loads are not part of the onboard vehicle energy use but will impact total transportation system energy use. However, DOE (2020) estimate that these off-vehicle energy requirements are likely to be very small on a per-vehicle basis.
8.3.8.2 Vehicle Mass and Aerodynamic Drag
Automation equipment (sensors, actuators, power needs, computing resources, and associated thermal management equipment) will increase weight. However, the increase will likely be quite small. Examples of systems in production (Level 2) or in prototype (Level 4) showed total equipment weight of 6–16.5 kg, which would increase fuel consumption by less than 1% (Gawron et al., 2018).
Automation technologies could dramatically improve vehicle safety. Level 1 to 3 vehicles are likely to crash less frequently than conventional vehicles, and Level 4 and 5 vehicles presumably will crash very rarely. If these highly automated vehicles were to achieve full penetration, crashworthiness requirements could be substantially reduced. In this scenario, reductions in vehicle mass could be achieved by eliminating some safety equipment such as air bags (passive systems) as well as metal reinforcements required in today’s vehicles. Weight de-compounding effects, including the ability to use smaller engine and auxiliary systems while maintaining performance, would further reduce vehicle mass and fuel consumption. However, full penetration of these higher levels of automation throughout the vehicle stock will not occur by 2035.
Sensors for some early CAV systems increased drag substantially (Gawron et al., 2018), but these systems are increasingly streamlined to improve aerodynamics and appearance. If a much-reduced risk of crashes were to cause some jurisdictions to increase highway speed limits, however, fuel use would increase owing to higher drag.
Automated and connected vehicles can operate cooperatively in a convoy-like “platoon.” Because of the fast response afforded by the electronically controlled braking, acceleration, and steering systems of autonomous vehicles, especially if the vehicles are connected to one another, cars can drive closer together with much smaller interspacing. This shields cars from aerodynamic drag, saving fuel. Platooning is being tested for tractor trailers, for which each percentage point improvement in fuel efficiency brings large fuel savings. While platooning has been projected to reduce fuel consumption by several percent (NACFE, 2016), the industry is still debating the cost-effectiveness of the technology in light of the likelihood of forming platoons (Menzies, 2019). For light-duty vehicles, where per-vehicle saving would be much smaller and a mechanism for platoon formation not yet clear, effectiveness is unproven.
FINDING 8.6: Power needs of sensors and onboard computers can be considerable, reaching multiple kilowatts in prototype vehicles with high levels of automation. Power draw for a given function will decline rapidly over time as electronic systems evolve and refine, but total electrical load of these systems may remain significant as their functionality increases, due especially to growing computing requirements. Other negative efficiency impacts of automation technology owing to increased load (e.g., increased weight or drag) are likely to be minimal by the time it is commercialized.
8.4 ESTIMATES OF FUEL EFFICIENCY EFFECTS
8.4.1 Combined Potential for Fuel Economy Improvement
Table 8.6 shows estimates of combined savings potential for three generic CAV technology packages. While the package descriptions reference the SAE levels of automation, they are meant to group CAVs according to the nature of the fuel savings opportunity rather than by the human/system division of driving responsibility. The first package has Level 2 automation, basically ACC. The second package adds powertrain controls, which optimize internal combustion engine (ICE) technology calibrations, and vehicle connectivity, which expands the vehicle’s look-ahead capability and can adjust operation accordingly. Here, Level 3 is not called out, because the fuel efficiency opportunities of capabilities like automated lane change are unclear. The third package includes
TABLE 8.6 Fuel Consumption Potential and Direct Manufacturing Cost for Three CAV Packages in 2020
Technology Package Fuel Savings Principles | Technologies in Package and Technology Assumptions | 2020 Package Cost | Package Effectiveness (Fuel Consumption Reduction) | ||||
---|---|---|---|---|---|---|---|
ICE | HEV | PHEV | BEV | Power Draw | |||
Level 2 automation: Optimized velocity, minimized acceleration events |
|
Total package cost: $1,520 Itemized costs: Camera: $45 Radar sensor: $55 Ultrasonic sensor: $10 ADAS Control Module: $800 Wiring: $90 | 5% urban/ 5% hwy | 4% urban/ 3% hwy | 8% in combined hwy and urban driving (longer than battery range)a | 4% urban/ 4% hwy | ~200 W; minor increase in fuel consumption |
Level 2 automation w/ PT controls + connectivity: Above plus:
|
Previous package plus:
|
Total package cost: $2,410 Above itemized costs plus: Transceiver: $75–$90 CPU: $800 | 9% urban/ 5% hwy | 6% urban/ 3% hwy | 20% in some driving conditions (longer than battery range) | 5% urban/ 4% hwy Additional 5% with optimum thermal and state-of-charge management | ~200 W; minor increase in fuel consumption |
Level 4/5 + connectivity: Above plus: Fully autonomous driving, permitting low-cost ride hailing, lower car ownership, and more high-efficiency vehicles |
Same as above, plus:
Level 5 can be used anywhere, while Level 4 may be used only in geofenced or other limited areas. |
Total package cost: $7,210–$17,210b Itemized costs: Lidar: $2,000 CPU: $800 |
Wide range of energy outcomes including savings from increased BEV adoption, vehicle “rightsizing,” and ridesharing, as well as higher consumption from autonomous driving system power draw and more vehicle miles traveled | Currently ~2.5 kW; 47% increase in fuel consumption for ICE/HEV, 30% for BEV300 (small car). Industry aim is to reduce power needs to < 1 kW. |
NOTE: Technology requirements and costs were developed by the committee from its information gathering. CAV technologies are added primarily for safety and other non-energy related purposes, so costs should not be entirely attributed to fuel economy. Package effectiveness estimates do not reflect operation over the standardized test cycles, but rather are mostly based on testing or simulation reflecting driving patterns closer to actual conditions and optimization for individual vehicles. The technology effectiveness represents an upper bound with respect to a baseline without the CAV technology packages. Level 3 automation does not appear in the table because no additional fuel efficiency benefits have been established for Level 3 capabilities. Hwy = highway.
a High-efficiency benefits from PHEV CAVs are observed when the entire trip before an opportunity to charge is approximately known and this trip involves highway driving when the battery state of charge is high, followed by urban driving.
b Vehicle not yet commercially available; range shown reflects hardware costs only. High costs of development, data acquisition, software, etc. are not included but should be much reduced once vehicles reach substantial sales volumes.
connected Level 4 and Level 5 vehicles capable of autonomous driving (though not yet commercially available). These vehicles may produce a wide range of impacts on vehicle fuel consumption, which are largely behavior-driven and are not quantified in the table but are further discussed in Chapter 9. Table 8.7 shows cost estimates for the three CAV packages in 2025, 2030, and 2035.
FINDING 8.7: Automation technologies can produce or enable diverse fuel efficiency impacts for the vehicle in which they are installed. Individual automation technologies may result in fuel savings of more than 10% in some driving conditions, although they can also increase fuel use if not implemented for efficiency.
FINDING 8.8: With reliable vehicle-to-infrastructure information, connected and automated vehicle technologies in combination could increase fuel efficiency by as much as 20% in some driving conditions. Unreliable communication could be detrimental and lead to increased fuel consumption.
8.4.2 Technology Adoption
The aggregate energy impacts of CAV technologies will depend in large part on their pace of adoption by the consumer and on public sector investment and policy to guide and support their use. Level 1 and Level 2 automated vehicle systems are available today, at a cost of $1,500 to $3,000 (EIA, 2018). These systems are beginning to achieve substantial take rates even on high volume vehicles. In the second quarter of 2019, 10% of new cars sold in the United States had Level 2 driving capability, up from 2% a year earlier (Canalys, 2019). Toyota and Nissan have overtaken premium car brands in volume of Level 2 vehicles sold. Canalys (2019) notes that in the second quarter of 2019, more than 60% of cars sold with Level 2 automation cost less than $40,000.
Figure 8.7 shows projected penetration of safety-oriented ADAS features such as automatic emergency braking and LDW increasing dramatically over the next 20 years, starting at 10%–50% in 2020 and increasing to at least 80% in 2035 for almost all technologies shown. While these features are generally distinct from those that deliver energy efficiency benefits, they share equipment with some of the eco-driving capabilities discussed above and hence suggest a very high penetration of lower levels of automation by 2035.
It should be noted that none of the systems deployed to date include powertrain optimization, and connectivity, whether V2V or V2I, has very limited implementation at this point. Many automakers are hesitant to deploy these technologies owing to weak return on investment, especially while penetration, and hence driver benefits, of many CAV capabilities are small. Moreover, the systems may not be implemented to achieve maximum fuel savings. Hence the energy efficiency benefits of today’s Level 2 vehicles are likely at the low end of the potential gains shown above.
The effects of connectivity on fuel economy depend on its prevalence in the vehicle population. Although studies have shown that connected vehicles have an influence on non-connected vehicles, the overall improvement would be larger if all vehicles were communicating. There are several companies working on aftermarket solutions for “legacy” vehicles and this work is expected to be emphasized as the benefits for connectivity related to safety predominately are clarified. The ability for all vehicles to communicate has the potential to greatly enhance safety and fuel economy.
Level 3 systems are emerging on luxury vehicles. Mercedes has announced a Level 3 version of its Drive Pilot system, and BMW plans to introduce the all-electric iNext crossover with Level 3 automation (Davies, 2020). BMW’s mid-2021 release will be for a limited highway pilot only, however. The system may not be capable of adjusting to pedestrians, for example, and will not operate during lightning events (Fuerst, 2020). More generally, the auto industry has raised safety and liability concerns in connection with Level 3 deployment, and there is currently no consensus on when, or even whether, Level 3 will become widespread in the vehicle market (Szymkowski, 2020).
While many automakers previously announced that they would deploy fully autonomous vehicles before 2020, most early deadlines have fallen by the wayside (Figure 8.8). Now automakers are planning for Level 4 releases in the mid-2020s. Level 4 systems are far more expensive, and automakers have expressed uncertainty about consumers’ willingness to pay for them. However, those costs are declining rapidly as lidar and computing system design advance.
TABLE 8.7 Technology Costs for the Three CAV Cost Packages as of 2020, and Cost Projections in 2025, 2030, and 2035
Technology Package and Fuel Savings Principles | Technologies in Package and Technology Assumptions | Estimated and Projected Technology Cost (DMC, 2018$) | |||
---|---|---|---|---|---|
2020 | 2025 | 2030 | 2035 | ||
Level 2 automation Optimized velocity, minimized acceleration events |
|
$1,520 | $1,446 | $1,375 | $1,307 |
Level 2 automation w/ PT controls + connectivity Above plus:
|
Previous package plus:
|
$2,410 | $2,292 | $2,180 | $2,073 |
Level 4/5 + connectivity Above plus: Fully autonomous driving, permitting low-cost ride hailing, lower car ownership, and more high-efficiency vehicles |
Same as above, plus:
L5 can be used anywhere, while L4 may be used only in geofenced or other limited areas. |
$7,210–$17,210 | $3,725–$5,406 | $3,511–$5,030 | $2,545–$4,683 |
NOTE: The cost projections assume a steep cost reduction of 30% annually between 2020 and 2025, and 2% annually from 2025 to 2035 for lidar technology, and 1% annually for all years for other technologies.

SOURCE: Farmer (2019).
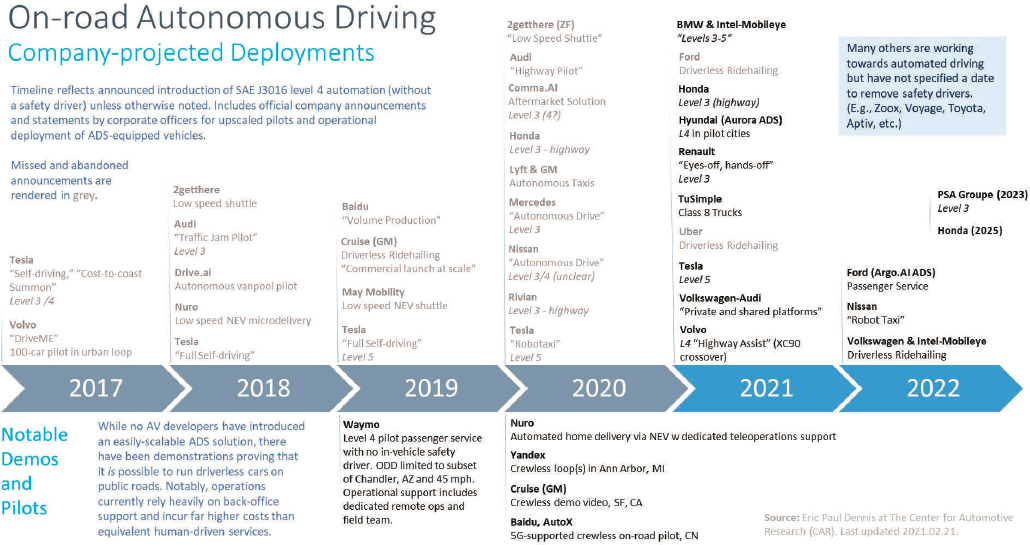
SOURCE: Dennis (2020).
Ongoing trends in cost reduction for CAV hardware and software suggest that cost of even high levels of automation could be within reach of many new car buyers before 2035. However, technology challenges and liability concerns will likely linger. This means limitations on the locations or conditions in which the vehicle can operate in autonomous mode. This in turn will limit these vehicles’ market appeal to consumers. However, such limitations may be compatible with the requirements of some applications such as ride hailing, where these vehicles may generate large economic benefit.
FINDING 8.9: The share of new vehicles equipped with Level 2 automation technology first exceeded 10% in early 2019, with a rapid rate of adoption in mainstream vehicles. Level 3 is going to appear in the market in the early 2020s in limited applications, but widespread concerns in the industry regarding the safety and liability issues associated with a system in which vehicle and driver each have primary responsibility under some conditions make it difficult to project the adoption trajectory for Level 3. Consumer acceptance of Level 4 is uncertain at present, but with continued declines in cost, increases in capabilities, and increases in consumer familiarity with automated features, it may be common by 2035. The economics of autonomous (Levels 4 and 5) vehicles will be highly advantageous for fleets, which are likely to lead in building the market for these vehicles.
FINDING 8.10: Connectivity is unlikely to be widely deployed in 2025 but could reach high adoption levels by 2035 if public infrastructure is updated to collect, process, and distribute data and if useful, affordable, connectivity services are available.
8.5 POLICY ISSUES RELATED TO CAV ENERGY IMPACTS
New technologies often necessitate changes to policy. Vehicle safety standards, for example, can be expected to change in response to CAV technologies and could ultimately eliminate equipment requirements that these new, safer vehicles will render obsolete. At the same time, proactive changes to policy can guide deployment of new technology to ensure that it delivers on potential societal benefits.
Policies for CAVs are in the early stages of development. The National Highway Traffic Safety Administration proposed Federal Motor Vehicle Safety Standard No. 150 mandating V2V connectivity on all new light-duty vehicles in 2016, but this rule was never adopted (NHTSA, 2016). The FCC’s recent decision to reduce the width of the spectrum dedicated to ITS communications and to specify that the remaining 30 MHz is to be used exclusively for C-V2X may end the option to move ahead with DSRC V2X and could limit the use of V2X altogether in the United States (FCC, 2020b). The few policies that have been adopted or explored at the federal level have focused on safety, cybersecurity, and privacy issues and standardization of equipment and protocols, not energy use implications (US Senate, 2017; DOT, 2017). At least seven states have adopted policies to promote testing and deployment of CAVs, and most others have taken steps in that direction (EIA, 2017). Some local governments have developed policies or programs to guide how CAVs will be used in urban and suburban areas. However, these policies are generally concerned with fully autonomous vehicles and their potential implications for travel behavior rather than vehicle efficiency. These include changes in vehicle miles traveled, which will be discussed in Chapter 9.
8.5.1 CAV Technologies in Fuel Economy Standards
One domain in which energy impacts of CAVs have been discussed in some detail is fuel economy and emissions standards. While some CAV technologies can substantially improve energy efficiency, these savings would not generally raise a vehicle’s test fuel economy, because the test protocols are designed to ensure consistency of driving across all vehicles. Hence, precisely the features that would distinguish CAVs—changes to driving behavior—go undetected during the test. Consequently, various parties have advocated credits for CAV technologies under the standards, either specifically to recognize fuel savings not evident in testing or more generally as a way to incentive their adoption (Bin-Nun, 2018).
However, CAV technology fuel efficiency impacts can be large, as previously discussed, so accommodating these technologies outside the normal test protocols could have major effects on the Corporate Average Fuel
Economy program. Projected savings for Level 2 automation plus connectivity can exceed 10%. This is comparable to credits available under the standards for air conditioning improvements, which are a major element of manufacturer compliance. Furthermore, CAV technologies are typically driven by non-fuel economy benefits, so providing fuel economy credits for them would not necessarily result in further efficiency improvements but rather would displace other, on-cycle cost-effective efficiency technologies. One way to avoid this problem would be for the agencies to consider CAV technologies that can save energy in setting the level of the standards.
The velocity trajectories through which CAVs can achieve fuel efficiency improvements may differ significantly from the velocity profile of the standard fuel economy test cycles. Relaxing the constant velocity error margins around the standard velocity trajectories dictated by the federal test procedures would allow CAV fuel efficiency impacts over the certification test cycle to be quantified (Prakash et al., 2016). This could encourage manufacturer adoption and optimization for fuel efficiency. However, if the error margin in the velocity trajectory were to be relaxed in the testing of all vehicles, fuel economy would likely increase for all vehicles, reducing any detected efficiency benefit for CAVs.
As discussed in Chapter 12 (Regulatory Structure and Flexibilities), automakers can earn off-cycle credits under the standards for deploying technologies whose fuel savings benefits are not fully reflected on the official vehicle test cycles. The purpose of off-cycle credits is to help bring into the market technologies that improve real-world fuel economy but do not affect tested fuel economy. In principle, CAV technologies could be eligible for off-cycle credits when they do save fuel. While these technologies are generally offered to provide benefits other than fuel savings, automakers have indicated that in some instances the ability to obtain off-cycle credits increases the likelihood that they will deploy a CAV technology.
Fuel savings from CAV technologies may be difficult to document, especially at the confidence level required for off-cycle credits, or may differ greatly across vehicles. For example, ACC can provide fuel savings by smoothing acceleration. However, benefits may vary sharply from implementation to implementation (Zhu et al., 2019). An early analysis found that ACC could increase fuel use by up to 3% or decrease it by up to 10%, depending on the system’s time headway and following algorithms (Mersky and Samaras, 2016). As an added complication, corridor-level simulations have found that CAV eco-driving strategies can dramatically increase fuel consumption owing to traffic instabilities when penetration of the technology is high (DOE, 2020). Savings are restored and enhanced when there is connectivity between vehicles (Zhang and Cassandras, 2019). Delays or interruptions in information associated with low penetration of connected vehicles will also reduce the fuel efficiency benefits of CAVs (Hyeon et al., 2019). Other efficiency benefits from simultaneously managing velocity profiles in approaching and departing stop lights or signs rely on connectivity and the reliability of information broadcasting from signalized intersections (Bae et al., 2019). In such cases, credits would need to be justified separately for each implementation of technology in a vehicle model in order to properly represent the system’s fuel efficiency impact. In addition, the energy demands of CAV systems would need to be fully accounted for in determining the amount of any credits.
To date, the agencies have stated that, in order to be eligible for credits, an off-cycle technology must have benefits that are rigorously and fully documented and must reduce emissions from the vehicle receiving the credit. Technologies that reduce emissions primarily by changing the operation of vehicles other than the one in which the technology is installed (through collision avoidance or other means of improving traffic flow) are not eligible for off-cycle credit.2 This is reasonable, because the benefits of such technologies are subject to great uncertainty, and their effects on fuel consumption and emissions are qualitatively different from those the standards programs were designed to measure and promote.
Another novel issue raised by CAVs is that their fuel efficiency impacts may depend upon the availability of related technology in nearby vehicles and, in the case of connectivity, infrastructure. If the agencies were to consider credits for these technologies, the amount of credit should reflect realistic assumptions regarding technology
___________________
2 “Thus, for a technology to be ‘counted’ under the credit provisions, it must make direct improvements to the performance of the specific vehicle to which it is applied.” Federal Register 77(199):62733. “Off-cycle credits may not be approved for crash-avoidance technologies, safety critical systems or systems affecting safety-critical functions, or technologies designed for the purpose of reducing the frequency of vehicle crashes.” 40 CFR §86.1869-12(a).
adoption levels over the life of the vehicle. However, determining the appropriate level of credit in these cases would likely add considerable complexity to the program.
FINDING 8.11: Automation and connectivity enable, but do not ensure, fuel efficiency improvement over present vehicle technologies. Current fuel economy test cycles and procedures generally will not detect these improvements. Allowing limited departures from standard test cycles would permit some of these technologies’ fuel efficiency gains—and losses—to be measured in testing.
FINDING 8.12: While connected and automated vehicle (CAV) technologies today are generally offered to provide safety, comfort, or convenience, the ability to obtain off-cycle credits for a CAV technology would in some cases increase the likelihood that automakers would deploy it.
RECOMMENDATION 8.1: The U.S. Environmental Protection Agency, U.S. Department of Transportation, and U.S. Department of Energy should conduct research on current driving patterns in the United States sufficient to support sound estimates of the energy impacts of off-cycle fuel efficiency technologies including connected and automated vehicle technologies. This research should be updated at regular intervals.
RECOMMENDATION 8.2: In setting the stringencies of the fuel efficiency and greenhouse gas emissions standards, the U.S. Department of Transportation and U.S. Environmental Protection Agency should consider the contribution that connected and automated vehicle technologies could make to saving energy. Off-cycle credits should be available for connected and automated vehicle technologies only to the extent they improve the fuel efficiency of the vehicle on which they are installed. Credits should be based on realistic assumptions, where needed, regarding technology adoption on other vehicles or infrastructure.
8.5.2 Other Policies to Promote CAV Technology Adoption
Thus far, automakers have been developing technologies that use only information acquired on the vehicle, information shared from other vehicles within the company, or through information sharing platforms such as HERE, or existing commercial and public information, including various maps and data on traffic. Advanced Transportation and Congestion Management Technologies Deployment Program Grants have been funded from an experimental perspective to be able to ascertain safety and fuel economy improvements. Also, in 2020, the Federal Highway Administration released a notice of funding opportunity for “up to $60 million in Federal Funding to provide grants to eligible entities to develop model deployment sites for large scale installation and operation of advanced transportation technologies to improve safety, efficiency, system performance, and infrastructure return on investment” (FHWA, 2020). Individual automakers or partnerships between automakers and others can provide a basis for automated and connected vehicle technologies, but efforts at the national, state, and local level will be required to instrument infrastructure for connectivity and provide consistent regulatory environment for CAVs nationwide.
RECOMMENDATION 8.3: The U.S. Department of Transportation should consider how it could promote consistency and efficiency in creating and maintaining high-definition maps of U.S. roads and develop its role accordingly.
8.6 REFERENCES
ABI Research. 2018. “V2X System Cost Analysis: DSRC+LTE and C-V2X+LTE.” https://unex.com.tw/public/uploads/shortcuts/ABI-DSRC-price-comparison.pdf.
Abuelsamid, S. 2019. “Nvidia Unveils Next-Generation Orin Chip to Power Self-Driving Vehicles.” Forbes. December 17. https://www.forbes.com/sites/samabuelsamid/2019/12/17/nvidia-announces-next-generation-orin-chip-for-automated-vehicles/#7acc35f4f373.
Autotrader. 2020. “What Is the Mercedes-Benz Driver Assistance Package?” Autotrader. August 10. https://www.autotrader.com/car-tech/what-is-the-mercedes-benz-driver-assistance-package.
Bae, S., Y. Choi, Y. Kim, J. Guanetti, F. Borrelli, and S. Moura. 2019. “Real-Time Ecological Velocity Planning for Plug-in Hybrid Vehicles with Partial Communication to Traffic Lights.” ArXiv:1903.08784 [Cs], September. http://arxiv.org/abs/1903.08784.
Baxter, J. A., D.A. Merced Cirino, D.J. Costinett, L.M. Tolbert, and B. Ozpineci. 2018. Review of Electrical Architectures and Power Requirements for Automated Vehicles. Oak Ridge National Laboratory. doi:10.1109/ITEC.2018.8449961.
Bin-Nun, A. 2018. “Using Fuel Efficiency Regulations to Conserve Fuel and Save Lives by Accelerating Industry Investment in Autonomous and Connected Vehicles.” Securing America’s Energy Future. https://secureenergy.org/AVsandFuelEconomy/.
Brooke, L. 2020a. “Origin of the Species.” SAE International. May 11. https://www.sae.org/news/2020/05/cruise-origin-chief-engineer-qa.
Brooke, L. 2020b. “Ultrasonics to Keep Lidar Clean.” SAE International. Magazine Article 20AVEP11_08, 2020-11-06. https://saemobilus.sae.org/content/20AVEP11_08/.
Butts, K., for M. Huang, S. Zhang, and Y. Shibaike. 2019. “Real-time Long Horizon Model Predictive Control of a Plug-in Hybrid Vehicle Power-Split Utilizing Trip Preview.” Paper 20199015. PFL 2019 Proceedings. Tokyo: Society of Automotive Engineers of Japan.
Cadillac Pressroom. 2020. “Cadillac to Roll Out Enhanced Super Cruise.” media.gm.com. January 28. https://media.gm.com/media/us/en/cadillac/home.detail.html/content/Pages/news/us/en/2020/jan/0128-cadillac.html.
Canalys. 2019. “10% of New Cars in the US Sold with Level 2 Autonomy Driving Features.” September 9. https://www.canalys.com/newsroom/canalys-level-2-autonomy-vehicles-US-Q2-2019.
CAR (Center for Automotive Research). 2012. “Connected Vehicle Technology Industry Delphi Study.” https://www.michigan.gov/documents/mdot/09-27-2012_Connected_Vehicle_Technology_-_Industry_Delphi_Study_401329_7.pdf.
Chen, D., N. Prakash, A. Stefanopoulou, M. Huang, Y. Kim, and S. Hotz. 2018. “Sequential Optimization of Velocity and Charge Depletion in a Plug-in Hybrid Electric Vehicle.” Conference: 14th International Symposium on Advanced Vehicle Control, Bejing, China.
Chen, D., N. Prakash, and A. Stefanopoulou. 2020. “An Iterative and Hierarchical Approach to Co-optimizing the Velocity Profile and Power-split of Plug-in Hybrid Electric Vehicles.” Conference: 2020 American Control Conference, Denver, CO, USA.
Davies, A. 2020. “BMW Takes Self-Driving to the Next Level.” Autoweek. June 23. https://www.autoweek.com/news/technology/a32852529/bmw-takes-self-driving-to-the-next-level/.
Dennis, E.P. 2020. Announced Deployment Timeline. Center for Automotive Research.
DOE (U.S. Department of Energy). 2020. “SMART Mobility Connected and Automated Vehicles Capstone Report.” Office of Energy Efficiency and Renewable Energy.
DOT. 2017. Automated Driving Systems 2.0: A Vision for Safety. National Highway Traffic Safety Administration. https://www.nhtsa.gov/sites/nhtsa.dot.gov/files/documents/13069a-ads2.0_090617_v9a_tag.pdf.
DOT. 2020a. “Connected Vehicles and Cybersecurity.” Department of Transportation. https://www.its.dot.gov/factsheets/pdf/cv_%20cybersecurity.pdf.
DOT. 2020b. “The 5.9 GHZ Safety Band.” https://www.transportation.gov/sites/dot.gov/files/2020-02/Safety_Band_Where_Is_the_Safety_Band_in_Use.pdf.
DOT. 2020c. “First Report and Order, Further Notice of Proposed Rulemaking, and Order of Proposed Modification from the Federal Communications Commission in the Matter of Use of the 5.850–5.925 GHz Band (ET Docket No. 19-138).” https://ecfsapi.fcc.gov/file/1109637413744/2020.11.06%20DOT%20Letter%20to%20FCC%20Chairman%20re%20Comments%20on%20Safety%20Band%20Decision%20(Signed).pdf.
EIA (Energy Information Administration). 2017. “Study of the Potential Energy Consumption Impacts of Connected and Automated Vehicles.” https://www.eia.gov/analysis/studies/transportation/automated/pdf/automated_vehicles.pdf.
EIA. 2018. “Autonomous Vehicles: Uncertainties and Energy Implications.” Annual Energy Outlook 2018: Issues in focus. https://www.eia.gov/outlooks/aeo/section_issues.php#av.
Farmer, C.M. 2019. “Fuel Economy and Highway Safety.” Presentation to the Committee on Assessment of Technologies for Improving Fuel Economy of Light-Duty Vehicles—Phase 3.” Insurance Institute for Highway Safety. September 19.
FCC (Federal Communications Commission). 2019a. “Dedicated Short Range Communications (DSRC) Service.” FCC web page. https://www.fcc.gov/wireless/bureau-divisions/mobility-division/dedicated-short-range-communications-dsrc-service.
FCC. 2019b. “FCC Seeks to Promote Innovation in the 5.9 GHz Band.” Press release. December 12. https://docs.fcc.gov/public/attachments/DOC-361339A1.pdf.
FCC. 2020a. “FCC Modernizes the 5.9 GHz Band for Wi-Fi and Auto Safety.” https://docs.fcc.gov/public/attachments/DOC-368228A1.pdf.
FCC. 2020b. “Use of the 5.850–5.925 Band.” Proposed rule. Federal Register. Vol. 85, No. 25.
FHWA (Federal Highway Administration). 2020. “Advanced Transportation and Congestion Management Technologies Deployment.” https://www.fhwa.dot.gov/fastact/factsheets/advtranscongmgmtfs.cfm.
Ford Motor Company. 2020. “How Ford’s Next-Gen Test Vehicle Lays the Foundation for Our Self-Driving Business.” Medium. October 20. https://medium.com/self-driven/how-fords-next-gen-test-vehicle-lays-the-foundation-for-our-self-driving-business-aadbf247b6ce.
Fuerst, S. 2020. “Automated Vehicle Technology, Public Policy and BMW’s Level 3 AV System.” Webinar. Eno Center for Transportation. July 14. https://www.enotrans.org/event/webinar-automated-vehicle-technology-public-policy-and-bmws-level-3-av-system/.
Gawron, J., G.A. Keoleian, R.D. De Kleine, T.J. Wallington, and H.C. Kim. 2018. Life cycle assessment of connected and automated vehicles: Sensing and computing subsystem and vehicle level effects. Environmental Science and Technology 52(5):3249–3256.
Green Car Congress. 2020. “ABI Research Forecasts 83M 5G C-V2X Connected Cars by 2035.” March 16. https://www.greencarcongress.com/2020/03/20200316-abi.html.
Guanetti, J., Y. Kim, and F. Borrelli. 2018. Control of connected and automated vehicles: State of the art and future challenges. Annual Reviews in Control 45:18–40. https://doi.org/10.1016/j.arcontrol.2018.04.011.
Guzzella, L., and A. Sciarretta. 2013. Vehicle Propulsion Systems: Introduction to Modeling and Optimization. 3rd ed. Berlin Heidelberg: Springer-Verlag. https://doi.org/10.1007/978-3-642-35913-2.
Hamza, K., J. Willard, K. Chu, and K.P. Laberteaux. 2019. Modeling the effect of power consumption in automated driving systems on vehicle energy efficiency for real-world driving in California. Transportation Research Record 2673(4):339–347. https://doi.org/10.1177/0361198119835508.
Hardman, G., J.H. Lee, and G. Tal. 2019. “Partially Automated Vehicles and Travel Behavior.” University of California, Davis. Presentation to NASEM Fuel Economy Committee. January 24.
He, C.R., and G. Orosz. 2017. “Saving Fuel Using Wireless Vehicle-to-Vehicle Communication.” In 2017 American Control Conference (ACC), 4946–4951. https://doi.org/10.23919/ACC.2017.7963721.
Hellstrom, E., M. Jankovic, M.H. Shelby, P. Pietrzyk, A. Richards, and J. Rollinger. 2018. “Improving Transient Torque Response for Boosted Engines with VCT and EGR.” SAE 2018-01-0861. https://doi.org/10.4271/2018-01-0861.
Huang, M., Zhang, S., and Shibaike, Y. 2019. “Real-time Long Horizon Model Predictive Control of a Plug-in Hybrid Vehicle Power-Split Utilizing Trip Preview.” SAE Technical Paper 2019-01-2341. https://doi.org/10.4271/2019-01-2341.
Hyeon, E., Y. Kim, N. Prakash, and A.G. Stefanopoulou. 2019. “Short-Term Speed Forecasting Using Vehicle Wireless Communications.” In 2019 American Control Conference (ACC), 736–741. https://doi.org/10.23919/ACC.2019.8814400.
Iaconangelo, D. 2020. “Will Self-Driving Cars Slow the EV Boom? It Depends.” Energywire. July 1. https://www.eenews.net/energywire/stories/1063483527/print.
Karbowski, D., J. Jeong, D. Shen, J. Han, N. Kim, and Y. Zhang. 2020. “Energy-Efficient Connected and Automated Vehicles—EEMS016.” Presentation at the Department of Energy Vehicle Technologies Office Annual Merit Review.
Korosec, K. 2020. “Ford Postpones Autonomous Vehicle Service Until 2022.” TechCrunch. April 28. https://social.techcrunch.com/2020/04/28/ford-postpones-autonomous-vehicle-service-until-2022/.
Krok, A. 2020. “Velodyne’s Tiny Velabit Packs a Big Lidar Punch for Just $100.” CNET Road Show. January 7. https://www.cnet.com/roadshow/news/velodyne-velabit-small-inexpensive-lidar-ces/.
Lee, S., Y. Kim, H. Kahng, S. Lee, S. Chung, T. Cheong, K. Shin, et al. 2020. Intelligent traffic control for autonomous vehicle systems based on machine learning. Expert Systems with Applications 144. https://doi.org/10.1016/j.eswa.2019.113074.
Lopez, J. 2019. “The Cadillac CTS Sedan Officially Discontinued.” Cadillac Society (blog). July 1. https://cadillacsociety.com/2019/07/01/the-cadillac-cts-sedan-is-now-officially-discontinued/.
Markets and Markets. 2019. “HD Map for Autonomous Vehicles Market by Solution (Cloud-Based and Embedded), Level of Automation, Usage (Passenger and Commercial), Vehicle Type, Services (Advertisement, Mapping, Localization, Update and Maintenance), and Region—Global Forecast to 2030.” October. https://www.marketsandmarkets.com/Market-Reports/hd-map-autonomous-vehicle-market-141078517.html.
Martinez, C.M., M. Heucke, F. Wang, B. Gao, and D. Cao. 2018. Driving style recognition for intelligent vehicle control and advanced driver assistance: A survey. IEEE Transactions on Intelligent Transportation Systems 19(3):666–676. March. doi: 10.1109/TITS.2017.2706978.
Menzies, J. 2019. “Daimler Abandons Platooning to Focus on Automation.” Truck News.com. https://www.trucknews.com/technology/daimler-abandons-platooning-to-focus-on-automation/1003089387/.
Mercedes-Benz USA. 2017. “Driver Assistance Tech FAQ.” Mercedes-Benz USA Online Newsroom. October 9. https://media.mbusa.com/releases/driver-assistance-tech-faq?firstResultIndex=0&sortOrder=PublishedDescending.
Mersky, A., and C. Samaras. 2016. Fuel economy testing of autonomous vehicles. Transportation Research Part C: Emerging Technologies 65:31–48. doi:10.1016/j.trc.2016.01.001.
Michigan Tech Research Institute. 2017. “Benchmarking Sensors for Vehicle Computer Vision Systems.” Michigan Technological University. https://mtri.org/automotivebenchmark.html.
Mohan, A., S. Sripad, P. Vaishnav, and V. Viswanathan. 2020. Trade-offs between automation and light vehicle electrification. Nature Energy 5(7):543–549. https://doi.org/10.1038/s41560-020-0644-3.
MS Foster. n.d. “Camwash Vehicle Camera Washing System.” https://msfoster.com/products/cameras-cleaning/camwash/camwash/#:~:text=The%20vehicle%20camera%20washing%20system%2C%20CamWash%2C%20cleans%20away,It%20eliminates%20dirt%20without%20scratching%20the%20camera%20lens.
NACFE (North American Council for Freight Efficiency). 2016. Confidence report: Two-truck platooning. https://nacfe.org/wp-content/uploads/2018/02/TE-Platooning-CR-FINAL-_0.pdf.
Nazari, S., R.J. Middleton, J. Martz, and A.G. Stefanopoulou. 2017. “The Elusive Consequences of Slow Engine Response on Drive Cycle Fuel Efficiency.” In 2017 American Control Conference (ACC), 5379–5385. https://doi.org/10.23919/ACC.2017.7963791.
Nazari, S., N. Prakash, J. Siegel, and A. Stefanopoulou. 2019. “On the Effectiveness of Hybridization Paired with Eco-Driving.” In 2019 American Control Conference (ACC), 4635–4640. Philadelphia: IEEE. https://doi.org/10.23919/ACC.2019.8814975.
News Wheel. 2019. “Ford Plans First C-V2X Vehicles for China by 2021.” https://thenewswheel.com/first-ford-c-v2x-vehicles-china-2021/.
NHTSA (National Highway Traffic Safety Administration). 2016. “Federal Motor Vehicle Safety Standards; V2V Communications.” Notice of Proposed Rulemaking. https://www.govinfo.gov/content/pkg/FR-2017-01-12/pdf/2016-31059.pdf.
Olin, P., K. Aggoune, L. Tang, K. Confer, J. Kirwan, S.R. Deshpande, S. Gupta, et al. 2019. “Reducing Fuel Consumption by Using Information from Connected and Automated Vehicle Modules to Optimize Propulsion System Control.” SAE 2019-01-1213. https://doi.org/10.4271/2019-01-1213.
Ors, A. 2017. “RADAR, Camera, Lidar, and V2X for Autonomous Cars.” NXP website blog. May 24. https://blog.nxp.com/automotive/radar-camera-and-lidar-for-autonomous-cars.
Paganelli, G., S. Delprat, T.M. Guerra, J. Rimaux, and J.J. Santin. 2002. “Equivalent Consumption Minimization Strategy for Parallel Hybrid Powertrains.” In Vehicular Technology Conference. IEEE 55th Vehicular Technology Conference. VTC Spring 2002 (Cat. No.02CH37367) 4(4):2076–2081. https://doi.org/10.1109/VTC.2002.1002989.
Patel, J. 2020. “Cadillac Super Cruise Pricing Starts At $2,500 On 2021 Escalade.” CarsDirect. May 15. https://www.carsdirect.com/automotive-news/cadillac-super-cruise-pricing-starts-at-2-500-on-2021-escalade.
Paukert, C. 2018. “Why the 2019 Audi A8 Won’t Get Level 3 Traffic Jam Pilot in the US.” CNET Road Show. May 14. https://www.cnet.com/roadshow/news/2019-audi-a8-level-3-traffic-jam-pilot-self-driving-automation-not-for-us/.
Pozzi, A., S. Bae, Y. Choi, F. Borrelli, D.M. Raimondo, and S. Moura. 2020. “Ecological Velocity Planning through Signalized Intersections: A Deep Reinforcement Learning Approach.” In 2020 59th IEEE Conference on Decision and Control (CDC), 245–52. Jeju, South Korea: IEEE. https://doi.org/10.1109/CDC42340.2020.9304005.
Prakash, N., G. Cimini, A.G. Stefanopoulou, and M.J. Brusstar. 2016. “Assessing Fuel Economy from Automated Driving: Influence of Preview and Velocity Constraints.” In Volume 2: Mechatronics; Mechatronics and Controls in Advanced Manufacturing; Modeling and Control of Automotive Systems and Combustion Engines; Modeling and Validation; Motion and Vibration Control Applications; Multi-Agent and Networked Systems; Path Planning and Motion Control; Robot Manipulators; Sensors and Actuators; Tracking Control Systems; Uncertain Systems and Robustness; Unmanned, Ground and Surface Robotics; Vehicle Dynamic Controls; Vehicle Dynamics and Traffic Control, V002T19A001. Minneapolis: American Society of Mechanical Engineers. https://doi.org/10.1115/DSCC2016-9780.
Qualcomm. 2017. “Top 10 Things You May Not Know about C-V2X.” October 31. https://www.qualcomm.com/news/onq/2017/10/31/top-10-things-you-may-not-know-about-c-v2x.
Qualcomm. 2019. “How 5G Low Latency Improves Your Mobile Experiences [video].” OnQBlog. May 13. https://www.qualcomm.com/news/onq/2019/05/13/how-5g-low-latency-improves-your-mobile-experiences.
Rangwala, S. 2020. “Money for Everythin’” Forbes. November 23. https://www.forbes.com/sites/sabbirrangwala/2020/11/23/money-for-everythin/?sh=2a8dbbf44e1c.
SAE International. 2019. “SAE J3016 Levels of Driving.” https://www.sae.org/news/2019/01/sae-updates-j3016-automateddriving-graphic.
Sciarretta, A., and A. Vahidi. 2020. Energy-Efficient Driving of Road Vehicles: Toward Cooperative, Connected, and Automated Mobility. Lecture Notes in Intelligent Transportation and Infrastructure. Cham: Springer International. https://doi.org/10.1007/978-3-030-24127-8.
Stewart, J. 2018. “Self-Driving Cars’ Massive Power Consumption Is Becoming a Problem.” Wired. February 6. https://www.wired.com/story/self-driving-cars-power-consumption-nvidia-chip/.
SWRI (Southwest Research Institute) Team Presentation. 2019. NEXTCAR ARPA-E 2019 Annual Meeting.
Sun, J., Y. Feng, I. Kolmanovsky, D. Zhao, and C. Mi. 2019. “Integrated Power and Thermal Management for Connected and Automated Vehicles (IPTM-CAV) Through Real-Time Adaptation and Optimization.” ARPA-E review meeting. https://arpa-e.energy.gov/sites/default/files/1_2019_ARPA-E_AnnualMeeting_Slides_iPTM_CAVs_PublicRelease.pdf.
Szymkowski, S. 2020. “Audi Hangs up Hopes for Level 3 Partial Automation System.” CNET Road Show. https://www.cnet.com/roadshow/news/audi-a8-level-3-automation-traffic-jam-pilot-system/.
Taiebat, M., A.L. Brown, H. Safford, S. Qu, and M. Xu. 2018. A review on energy, environmental, and sustainability implications of connected and automated vehicles. Environmental Science & Technologyz. 52: 11449–11465.
U.S. Senate. 2017. S.1885. To support the development of highly automated vehicle safety technologies, and for other purposes. https://www.congress.gov/bill/115th-congress/senate-bill/1885/text.
V2X Core Technical Committee. 2020. “V2X Communications Message Set Dictionary.” 6th edition. SAE International. https://doi.org/10.4271/J2735_202007.
Vajedi, M., and N.L. Azad. 2016. Ecological adaptive cruise controller for plug-in hybrid electric vehicles using nonlinear model predictive control. IEEE Transactions on Intelligent Transportation Systems 17(1):113–122. https://doi.org/10.1109/TITS.2015.2462843.
Velodyne Lidar. 2020. “Velodyne Lidar Unveils Solid State Lidar Sensor.” Press Release. November 13. https://velodynelidar.com/press-release/velodyne-lidar-unveils-breakthrough-solid-state-lidar-sensor/.
Wang, P., Y. Li, S. Shekhar, and W.F. Northrop. 2019. “Actor-Critic Based Deep Reinforcement Learning Framework for Energy Management of Extended Range Electric Delivery Vehicles.” In 2019 IEEE/ASME International Conference on Advanced Intelligent Mechatronics (AIM), 1379–1384. Hong Kong, China: IEEE. https://doi.org/10.1109/AIM.2019.8868667.
Wardlaw, C. 2020. “Mercedes Takes ’21 S-Class to Next Level of Autonomous Driving.” Wards Auto. September 23. https://www.wardsauto.com/vehicles/mercedes-takes-21-s-class-next-level-autonomous-driving.
Wayland, M. 2020. “Volkswagen Closes $2.6 Billion Investment in Self-Driving Start-up Argo AI.” CNBC. June 2. https://www.cnbc.com/2020/06/02/vw-closes-2point6-billion-investment-in-self-driving-startup-argo-ai.html.
Zhang, Y., and C.G. Cassandras. 2019. An impact study of integrating connected automated vehicles with conventional traffic. Annual Reviews in Control 48:347–356. https://doi.org/10.1016/j.arcontrol.2019.04.009.
Zhu, L., J. Gonder, E. Bjarkvik, M. Pourabdollah, and B. Lindenberg. 2019. An automated vehicle fuel economy benefits evaluation framework using real-world travel and traffic data. IEEE Intelligent Transportation Systems Magazine 11(3):29–41.